Subject archive for "domino-data-science-field-note," page 2
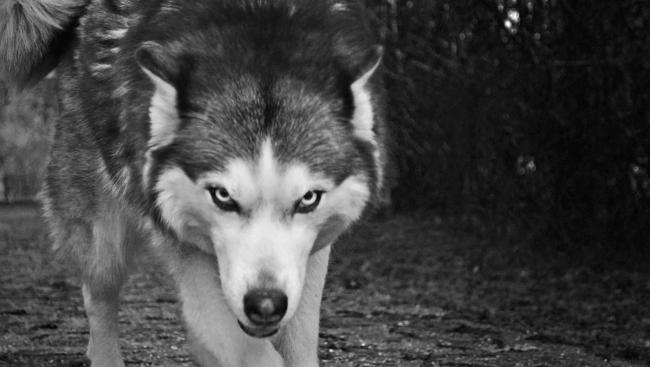
Trust in LIME: Yes, No, Maybe So?
In this Domino Data Science Field Note, we briefly discuss an algorithm and framework for generating explanations, LIME (Local Interpretable Model-Agnostic Explanations), that may help data scientists, machine learning researchers, and engineers decide whether to trust the predictions of any classifier in any model, including seemingly “black box” models.
By Ann Spencer7 min read
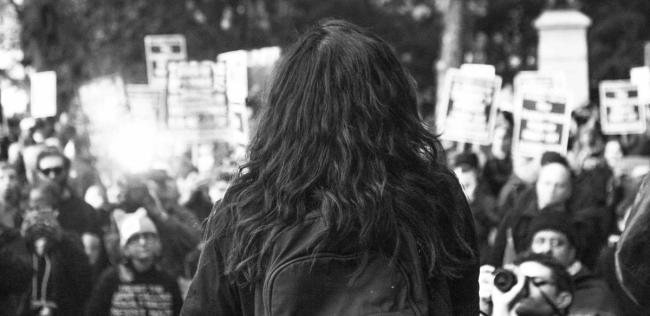
Make Machine Learning Interpretability More Rigorous
This Domino Data Science Field Note covers a proposed definition of machine learning interpretability, why interpretability matters, and the arguments for considering a rigorous evaluation of interpretability. Insights are drawn from Finale Doshi-Velez’s talk, “A Roadmap for the Rigorous Science of Interpretability” as well as the paper, “Towards a Rigorous Science of Interpretable Machine Learning”. The paper was co-authored by Finale Doshi-Velez and Been Kim. Finale Doshi-Velez is an assistant professor of computer science at Harvard Paulson School of Engineering and Been Kim is a research scientist at Google Brain.
By Ann Spencer8 min read
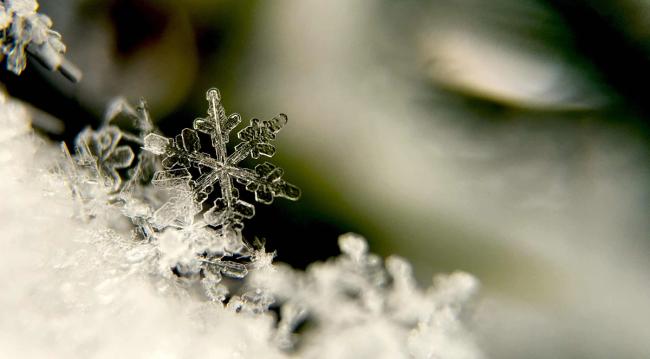
Learn from the Reproducibility Crisis in Science
Key highlights from Clare Gollnick’s talk, “The limits of inference: what data scientists can learn from the reproducibility crisis in science”, are covered in this Domino Data Science Field Note. The full video is available for viewing here.
By Domino5 min read
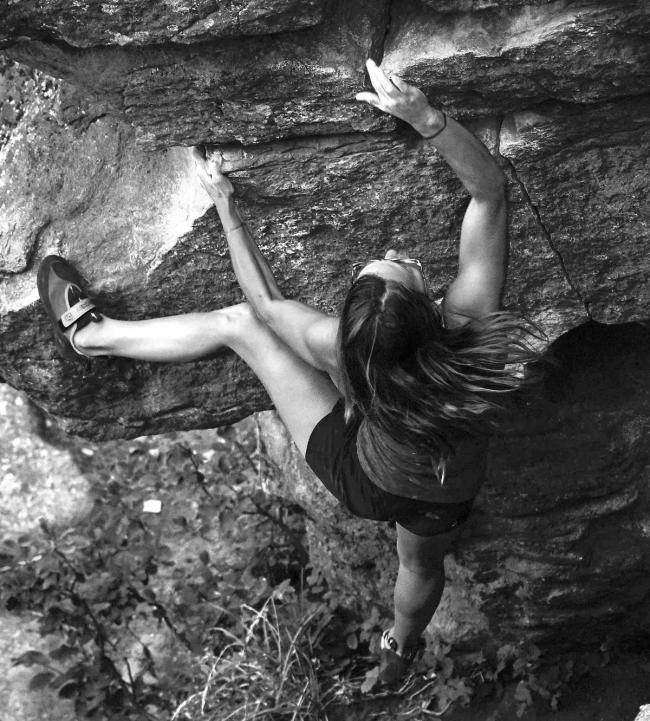
Feature Engineering: A Framework and Techniques
This Domino Field Note provides highlights and excerpted slides from Amanda Casari’s “Feature Engineering for Machine Learning” talk at QCon Sao Paulo. Casari is the Principal Product Manager + Data Scientist at Concur Labs. Casari is also the co-author of the book, Feature Engineering for Machine Learning: Principles and Techniques for Data Scientists. The full video of the talk is available here and special thanks to Amanda for providing permission to Domino to excerpt the talk’s slides in this Domino Field Note.
By Ann Spencer11 min read
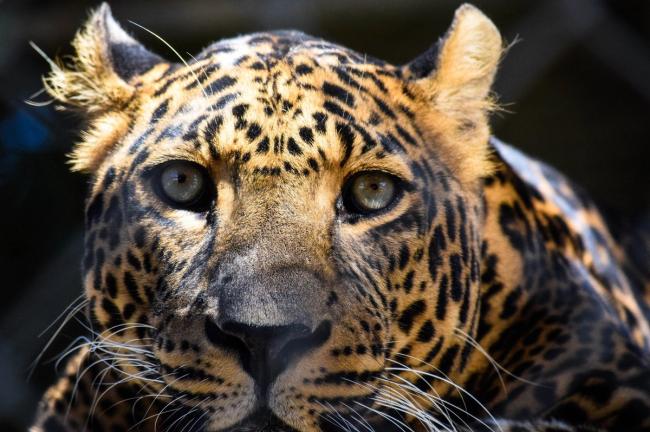
Model Evaluation
This Domino Data Science Field Note provides some highlights of Alice Zheng’s report, "Evaluating Machine Learning Models", including evaluation metrics for supervised learning models and offline evaluation mechanisms. The full in-depth report also includes coverage on offline vs online evaluation mechanisms, hyperparameter tuning and potential A/B testing pitfalls is available for download. A distilled slide deck that serves as a complement to the report is also available.
By Domino10 min read
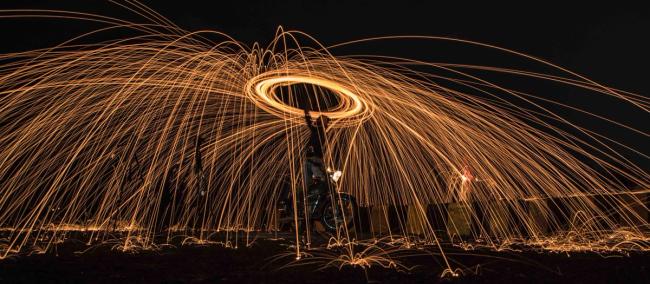
Data Science Models Build on Each Other
Alex Leeds, presented “Building Up Local Models of Customers” at a Domino Data Science Popup. Leeds discussed how the Squarespace data science team built models to address a key business challenge as well as utilized a complex organizational structure to accelerate data science work. This Domino Data Science Field Note provides highlights and video clips from his talk. The full video recording is also available for viewing. Also, if you would like additional information on building and managing models within an overall data science practice, then consider Domino’s model-management paper or practical guide for managing data science at scale.
By Domino6 min read
Subscribe to the Domino Newsletter
Receive data science tips and tutorials from leading Data Science leaders, right to your inbox.
By submitting this form you agree to receive communications from Domino related to products and services in accordance with Domino's privacy policy and may opt-out at anytime.