Subject archive for "featured," page 2
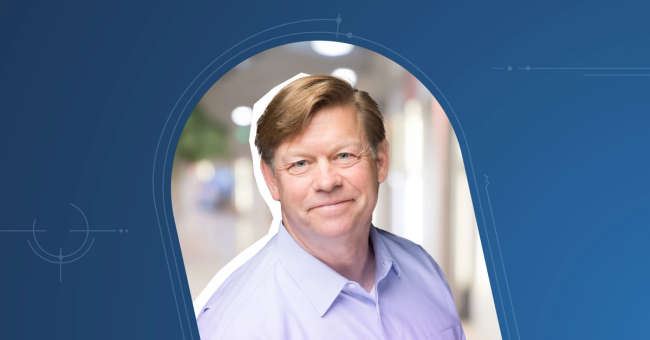
Top Innovator John Thompson: Why Managing Analytics Teams & Data Scientists Is a Unique Problem
Has the management of data science teams kept up with the meteoric rise of the discipline? A recent Domino Data Lab ebook, The Data Science Innovator’s Playbook, explores new approaches and thinking on the problem, from top data science innovators who have had a hand in building some of the world’s most successful teams. Some of them say that managing data science and analytics is a distinct, new problem that demands fresh approaches and rethinking old ideas learned in managing other types of teams, such as IT, operations personnel, and software developers.
By Lisa Stapleton4 min read
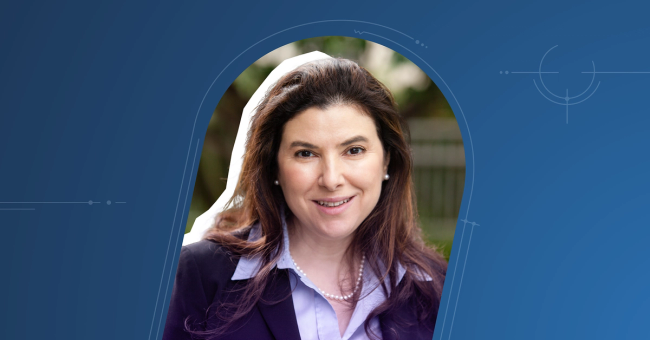
NVIDIA’s Mona Flores: How Medical AI and Federated Learning Power Innovation
What if machine learning and data scattered around the world held the keys to the cures for a variety of rare or new diseases? Until recently, it was often nearly impossible to use that data, due in part to the data privacy restrictions in place around the world. That’s starting to change, thanks to federated learning and the innovative work of doctors such as Mona Flores, head of medical AI at NVIDIA.
By Lisa Stapleton4 min read
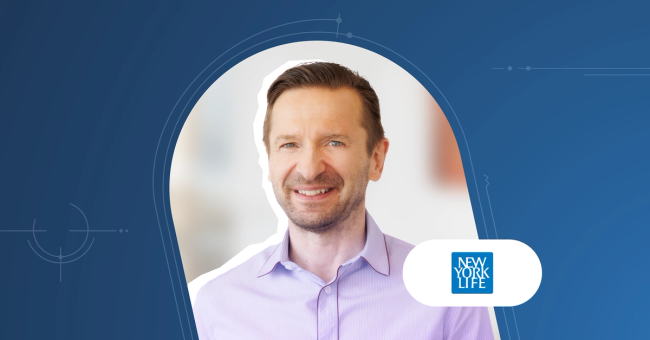
What the Rise of Data Science in Insurance Says About the Profession–and How It’s Changing
How do you build a data science capability into a powerful force for making decisions about virtually all facets of your business? And how do you recruit, train, organize, and retrain members of your team, in a field where competition for talent is intense and growing? These are just a couple of the questions that Glenn Hofmann, chief analytics officer at New York Life Insurance Company, has confronted and mastered in his tenure there.
By Lisa Stapleton4 min read
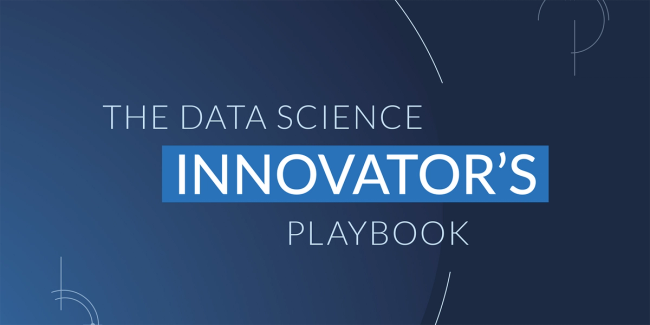
7 Top Innovators Share Insights, Trends and Career Advice in 'The Data Science Innovator’s Playbook'
Who’s doing the most innovative things in data science? Where is the profession going? And most importantly, what can you learn from some of the brightest in the business?
By Lisa Stapleton5 min read
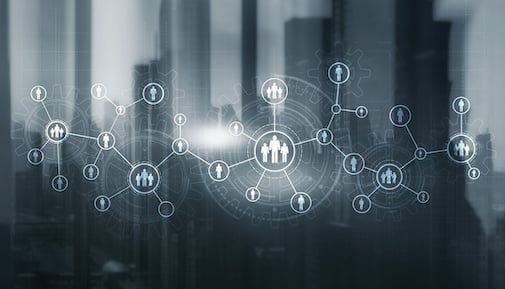
High-standard ML validation with Deepchecks
We've blogged before about the importance of model validation, a process that ensures that the model is performing the way it was intended and that it solves the problem it was designed to solve. Validations and tests are key elements to building machine learning pipelines you can trust. We've also talked about incorporating tests in your pipeline, which many data scientists find problematic. The issues stem from the fact that not all data scientists feel confident about traditional code testing methods, but more importantly, data science is so much more than just code. When validating pipelines we need to think about verifying the data integrity, inspecting its distributions, validating data splits, model evaluation, model comparison etc. But how can we deal with such complexity and maintain consistency in our pipelines? Enter Deepchecks - an open-source Python package for testing and validating machine learning models and data.
By Noam Bressler14 min read
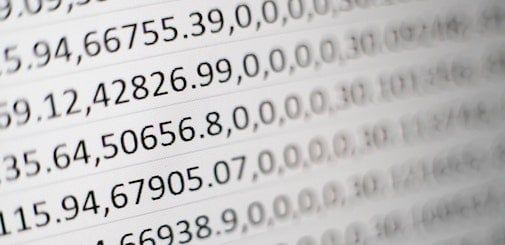
The Case for Reproducible Data Science
Reproducibility is a cornerstone of the scientific method and ensures that tests and experiments can be reproduced by different teams using the same method. In the context of data science, reproducibility means that everything needed to recreate the model and its results such as data, tools, libraries, frameworks, programming languages and operating systems, have been captured, so with little effort the identical results are produced regardless of how much time has passed since the original project.
By Sundeep Teki8 min read
Subscribe to the Domino Newsletter
Receive data science tips and tutorials from leading Data Science leaders, right to your inbox.
By submitting this form you agree to receive communications from Domino related to products and services in accordance with Domino's privacy policy and may opt-out at anytime.