Enterprise data science, machine learning, and AI at scale
Strategies to drive value from AI investments
Get the eBook
Are you on the brink of data- and AI-driven transformation but grappling with how to prioritize investments? You’re not alone.
Many enterprises stand where you are, navigating the complexities of hybrid and multi-cloud environments, data governance, centralized management of AI workloads, and managing AI talent challenges.
Domino and NVIDIA surveyed data science and IT leaders and created this eBook as a beacon to guide you through these complexities.
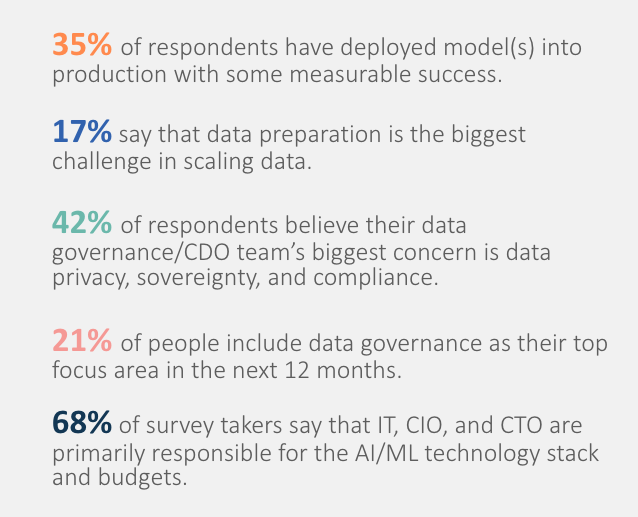
- Discover why most organizations are still in their infancy when it comes to operationalizing AI at scale.
- Understand the unique data requirements for internal and third-party sources that fuel AI workloads.
- Uncover technical challenges across the model lifecycle attributed to lack of data science tooling and infrastructure.
- Recognize the implications of a divide between data science leaders and IT on AI/ML stack decisions so you don’t underinvest in critical capabilities.
- Understand investment decision implications on your broader analytics, data, and AI/ML talent strategy.
Here's a sneak peek at the takeaways
Hybrid- and multi-cloud is the future
Hybrid is here to stay, with most organizations working with massive amounts of disparate, distributed data across on-premises, cloud, and multi-cloud environments.
Data privacy, sovereignty, and regulation compliance are key
Managing data scientists' access to sensitive data is one of the top concerns for organizations.
Lack of MLOps tooling impedes scale
Many of the challenges impeding AI at scale around data preparation, model training and evaluation, model deployment, etc., could be solved with investment in a the platform to unify AI initiatives.
IT holds the budget for AI investments
AI has unique tooling and infrastructure requirements, and IT must balance these investments against traditional expenditures.