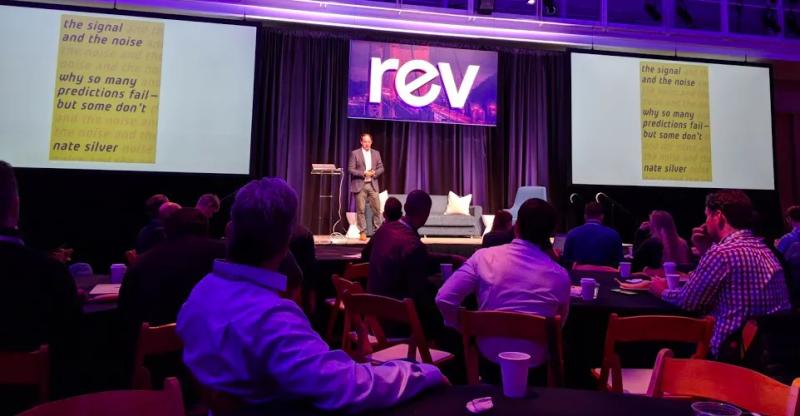
Thanks to Cassie Kozyrkov for contributing this blog, which was originally published in Medium’s Towards Data Science.
Data science has a problem. Several problems, actually, but to begin at the beginning, let’s start with one: leadership.
Today I spoke at a summit for leaders in data science (the discipline that spans machine learning, artificial intelligence, statistics, data summarization, and visualization). As I looked over at the sea of faces belonging to the brightest trailblazers guiding today’s data science teams, I found myself thinking, “There are too many of you.”
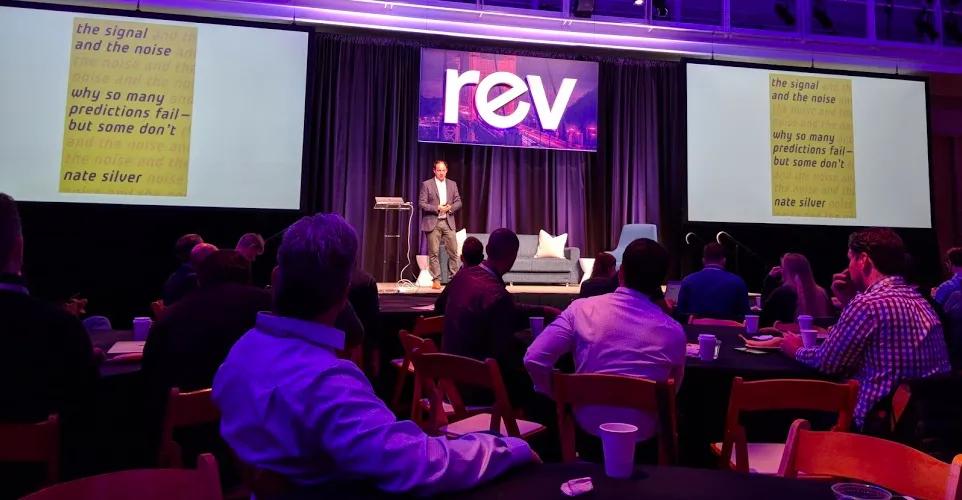
Nate Silver of FiveThirtyEight speaking to the same audience of data science leaders
What a thing to think! Ask anyone at the summit and they’d probably tell you that if you think the talent shortage is bad, the leadership talent shortage in data science is far, far worse.
Most data science leaders today are what I like to call “transcended data scientists.” People who pursued formal training in science, engineering, or statistics and then, by some miracle, woke up one day to realize that they were more interested in making data useful than chasing mathematical complexity for its own sake.
Data science leaders exist against all odds.
Data science leaders: There are too many of you because you exist against all odds. Since next to nothing was done to train you, there are more of you than we deserve. How did this happy accident happen? No one taught you how to do what you do, so we’re lucky you exist. Was the plan to hope that after studying equations for over a decade, you’d just figure out how to lead? How to make good decisions? As my SRE colleagues would say, “Hope is not a strategy.”
There should be more of you, but what’s the plan?
If you like theorems, here’s one: your time is finite, so if you’re using it to study Feynman or de Finetti, you aren’t spending as much of it to build other skills. We can’t expect data scientists to transcend and instantly know how to be good leaders and decision-makers. Who would have taught them that? You don’t learn it by writing code or proving theorems all day.
Instead, to become good leaders and decision-makers, they had to have the humility to recognize weakness in their atrophied muscles and the diligence to master a second craft. Sometimes with a lot of bruises while they learn the hard way. As someone who started out thinking that probability theory is the hottest thing in the universe, I know all too well how painful this can be.
There’s an attitude problem. Do we really value these skills?
If your experience was anything like mine, you might have grown up in a pro-math subculture in which it’s fashionable to display disdain for anything that smells like “soft” skills. It’s all chest-thumping about how hardcore you are for staying up all night proving some theorem or coding in your sixth language. It might not occur to you that you should value leadership (or communication, business sense, creativity, and empathy, for that matter) when you’re caught in the middle of that perspective… and will your classmates respect you if you go soft?
Part of the solution is changing the fashion so that these skills are a not-negotiable part of being hardcore at something as attractive as raw data science. If strutting must be part of it for youngsters, then lets at least convince them that the highest honor is in having both kinds of muscles to flex. It’s the truth, after all.
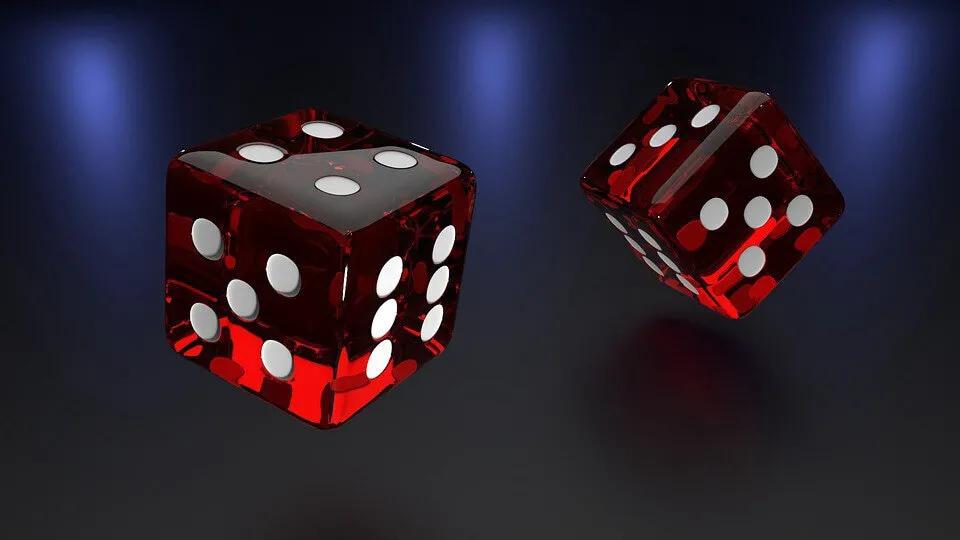
When it comes to ensuring that data science teams are lead effectively, are we relying on luck or training?
The bar is high and not everyone who has the job actually meets it.
Leadership in data science isn’t just leadership with a semester of numbers sprinkled on top. It’s its own strange beast. Not only must you have a deep understanding of decision-making and how information should drive actions, but you need a keen nose for the nuances of how to usefully impact your particular business domain and, as if that weren’t enough, you also need to understand the ecosystem of diverse skills that need to come together to make a large-scale data science project successful. And that’s just the minimum for entry into this game.
That’s a pretty high bar, and not everyone who leads data science teams meets it. Employers, how do you know if you’re hiring the real deal to lead your data team? What if your team already has bad data science leadership? How could you tell? There’s hardly any wisdom about this role for you to lean on. Whom could you even ask?
Is data science a bubble?
Today’s world is generating data like never before. And yet, sometimes I’m asked questions like, “Is data science a bubble?” I wish I could answer, firmly and with conviction, “Definitely not!” The truth is, it depends. Sadly, when I chat with folks across industry, I keep hearing the same story: “Our data scientists are useless! All they do is sit around publishing papers.” Will we have enough skilled leaders to prevent this all-too-common phenomenon? If data scientists don’t prove themselves valuable, they won’t be in those jobs for long.
It’s unfair to expect that a freshly-minted science PhD knows how to contribute meaningfully to business. That’s not what they spent all those years learning. Without guidance from someone who understands what data science involves and knows how to connect data with the business, the deck is stacked against them. To make sure data science is not a bubble, we urgently need specialized leadership. Where will it come from?
Where are the training programs for data science leaders? Hope is not a strategy.
People, let’s appreciate how lucky we are! Somehow, good data science leaders do exist and the skills are there. Not efficiently-acquired skills, since the double mastery was earned in series and perhaps painfully, but the right skills nonetheless. I hope you feel the urgency as strongly as I do. The few of us who learned the hard way need to start training more of us a better way.
I, for one, am committed to doing my part. For the past few years, I’ve been working hard within Google to grow a new breed of thinker, positioned to lead or work effectively as part of a team focused on the application of data science to real problems. To build the right skills, we took ideas from data science and engineering and augmented them with the behavioral and managerial sciences. The result only looks interdisciplinary until you see the common core: decisions and the information that drives them. That’s why we started calling it decision intelligence engineering (though you can think of it as applied data science++ if you prefer).
Let’s train a new breed of thinker: the decision-maker who has the skills to make data science teams successful.
I’ve always believed that data science is a team sport that benefits from skills diversity, so I designed our training program to encourage participation from, and be accessible to, humans of all backgrounds. It turns out that a great data science leader doesn’t have to be a transcended data scientist.
I’m proud of what we’ve achieved in fostering these skills among Googlers… but it’s not enough. There’s no need for everyone else to rely on happy accidents and full immersion in more than one discipline to build the same skills. I hope that reading this inspires at least a few of those who know the data science decision-maker’s craft to join me in recognizing it as a discipline in its own right and sharing our wisdom as widely as possible.
RELATED TAGS
Subscribe to the Domino Newsletter
Receive data science tips and tutorials from leading Data Science leaders, right to your inbox.
By submitting this form you agree to receive communications from Domino related to products and services in accordance with Domino's privacy policy and may opt-out at anytime.