Data Scientist Interview: Skylar Lyon from EBay for Accenture
Anna Anisin2014-12-16 | 14 min read
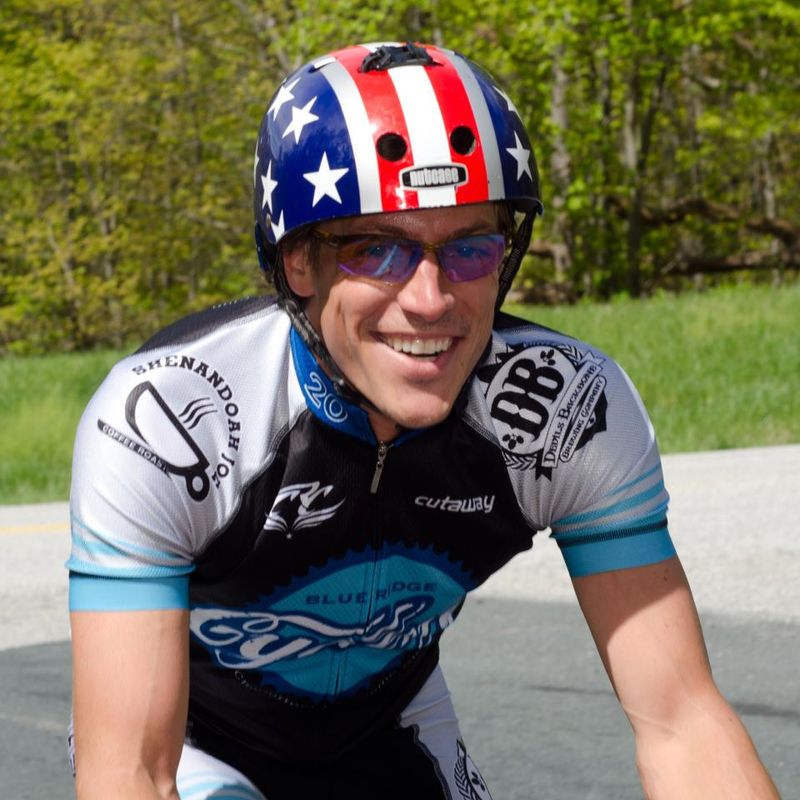
We recently caught up with Skylar Lyon, a senior data scientist working at EBay on behalf of Accenture.
What is your 30 second bio?
I was born and raised in Kansas City, MO, went out East to go to school to get my undergrad degree, which is where I got interested in data engineering, and science as well. Worked in the defense industry for about seven years developing and deploying predictive analytics, mostly spatio-temporal types of analytics and then decided to try the consulting life and see what’s going on out here in Silicon Valley. Its been short so far but very exciting, I’m really optimistic and hopeful, just the energy out here, its really contagious and a lot of fun. When I’m not playing with data or sitting in front of the computer, I’m generally participating in endurance athletics and either trying to climb or run long distances through the mountains. The mountains and the ocean are really what ground me and I think its important to take time to check in with yourself - that’s how I do it best.
How important is data in your personal life?
There was a long portion in my life not that long ago when I was completely data driven with regard to my own personal health and performance. I would get up every morning and check my resting heart rate and my heart rate variability score before getting out of bed and then immediately check hydration levels and then weigh myself. Many days I would wear a heart rate monitor throughout the day and always during exercise, just to collect all those statistics. Every Sunday I would sit down and plan my workouts for every day of the coming week and stick to that. If I was on the bike I was training by power data using a power meter, staying in very specific power/target zones that I would refine every week. I got quarterly blood tests to make sure all those levels were where I wanted them to be. Even now if someone offered me the opportunity to get a chip implanted which would record a lot of these biometrics I would absolutely take it.
What shifted your focus away from being data driven?
I wanted to return to "truer" just racing for the fun of it and not worrying about my results and performance quite as much and just enjoy the moment
You can’t improve what you don’t measure. I was measuring everything, seeking athletic improvement at that period of my life. I was racing much more competitively and I got burnt out by racing competitively and I wanted to return to “truer" just racing for the fun of it and not worrying about my results and performance quite as much and just enjoy the moment. So it was the combination of that and also my Garmin watch broke. I found that I couldn’t work out without recording and analyzing the data. I had to buy a new watch immediately. I had become dependent on the data and couldn’t perform a workout without seeing in real time what my numbers were. It got a bit obsessive, and now I don’t look at any of that. But I’m sure that I'll be back on that train before we know it; it will be hard to stay away from the Apple Watch.
How did you get interested in working with data?
I joined the systems engineering program at the University of Virginia and really I only chose that because it closed the fewest number of doors, it didn’t pigeonhole me in any career path. I wasn’t really sure what I wanted back then. I remember in my 3rd year, we really dove into systems engineering, which is really applied mathematics and statistics-heavy business applications — general problem solving. In our data modeling class we were studying the Challenger disaster and running regression models on the o-ring, the gasket that caused the explosion. It was at that point that I realized how powerful and insightful data can be. You can save thousands of hours of labor, human life and capitol that all went up in smoke in that accident, by simply understanding the data better and asking better questions to gain these insights. That was my “aha moment”, and that’s when I really got interested in the power of data.
What was the first data set you remember working with? What did you do with it?
That first canned data set [the Challenger data] got me excited. In the real world, I was working for Commonwealth Computer Research, Inc. on a predictive analytics platform that we developed for the army. The idea there was to help predict roadside bombs, IEDs (improvised explosive devices) and that was very exciting and interesting because I really felt like I was helping force protection and saving lives and using data toward that. These models that we were developing were deployed through Iraq and Afghanistan and I actually got to travel out to Iraq twice to deploy these models and sit with the soldiers and work with them and it was a life-changing experience.
Can you share a couple more interesting experiences that you had in the trenches?
The idea there was to help predict roadside bombs, IEDs ... It rose out of need for IED prediction but we generalized the model for all sorts of event prediction.
At more of an adventure level, I got to fly around in blackhawk helicopters over the city of Baghdad and out to Eastern Iraq provinces and to drive around in convoys through the city. Looking out through the very small, very thick windows of the MRAPs at the country as we passed by, it was just fascinating. Beyond that, another thing that was incredible was the size and scope of our operations over there because you don’t really understand what (VBC) Victory Base Camp is; its hard to really fathom what its like before actually arriving there. And it really is its own separate city with its own infrastructure and power grid and laundry facilitates and dining facilities. It’s HUGE! I never would have known from news reports in America. That really changed my perspective on the scope and scale of modern operations.
Another thing that was really cool about our predictive modeling, is that it rose out of need for IED prediction but we generalized the model for all sorts of event prediction. Just showing how a model can be developed with one very specific use case and then generalized to capture a much broader problem set. That was exciting.
What have you been working on this year, and why/how is it interesting to you?
The amount of data EBay has captured over the years is absolutely enormous! They have one of the largest data warehouses in the world. So the data is all there, now it’s a matter of figuring out what we do with it.
A little less than a year ago I pivoted and decided that I wanted to see what other data applications were out there in the world apart from defense. So I started working with EBay and in the world of marketing and trying to better understand the customer and her intent and behavior. Especially out here in Silicon Valley, what has been so fun for me in this most recent project has been working with outside vendors and incorporating other tool sets into pre-existing environment and looking at how we really can make sense of it all. The amount of data EBay has captured over the years is absolutely enormous! They have one of the largest data warehouses in the world. So the data is all there, now it’s a matter of figuring out what we do with it. A lot of traditional tools that were utilized in the past won’t cut it for this sort of size of truly big data.
Speaking of tools, have you found any great tools or applications that you like?
I think that there were two primary focuses for my initial study and those have been in-database in-memory processing and I think both offer practical solutions to sort of a different problem. In-memory is really good for interactive data manipulation and insight modeling and such and it can also be great for data-viz. Whereas in-database has proven extremely effective with EBay for just working at a whole different scale; scaling out across petabyte size data which is harder to capture in memory at the moment but is equally important for nightly batch jobs and such. These two technologies are complementing each other nicely.
From a modeling perspective, it’s interesting to see the resurgence of neural networks and deep learning models. They’ve proven especially powerful lately with lots of exciting recent developments. Models, like most things, come in and out of use, and at the moment Neural Networks are quite hot, proven to take regression and random forest type models a step further. Then it becomes about the amount of compute available, and can you train these models and run them fast enough to justify their complexity, and increasingly the answer is yes - and this is extremely powerful and interesting.
Where do you see the future of machine learning and deep learning? Where are we going?
This field is semi new, it’s new again. I think that is because now we have the compute capabilities and power that we didn’t have in the earlier days to really harness deep learning, so now as I mentioned earlier, there’s a resurgence on it and everyone is pouring money and time and energy into this right now and it’s a hot topic. I see trends forming and the entire landscape – the speed of innovation is only accelerating and I think that its going to catch a lot of people off guard, just how quickly the world around them will change. By the time I’m my mother’s age it will be a completely different world, my parents have seen a lot of transformation in the world but the world they see now, for the most part would be recognizable to them 30 years ago and I'm not sure how recognizable the world is going to be in another 30 years. I think that I lot of this will be driven by machine learning models and the incredible amount of compute that is now possible to run these models. Autonomous vehicles and drones, that’s just the tip of the iceberg. Once everyone is moving in a pod that they are all connected, that when the efficiencies will really be at a level we have trouble understanding and recognizing.
What are some of the innovators and thought leaders in the machine learning space? Who are the innovators that are doing the right things early on?
The community, quite honestly. I think that a lot of it is community driven and I think research institutions on down to kids just hacking on an old computer, they are all driving it. And I think it’s because of the promise and potential of it. Google has taken huge strides in providing thought leadership in this regard and have committed a lot of capital and human resources toward these initiatives. It would be foolish to discount them and the impact they’re having. H2O is another vendor that I’ve worked with, they are doing a lot to advance these mathematical models. They just had their first conference - H2O World - this year and there was a really deep crowd of very smart people not only presenting and proving the thought leadership you mentioned but also attending, learning. Those folks in the attendance will run with ideas of their own and figure out ways to leverage these tools and come up with new applications. One of the neat applications I saw recently was helping predict if a growing year will produce a top vintage wine. That’s fun.
Any words of wisdom for Data Science students or practitioners starting out?
I think the most important part is to keep an open mind and also not discount assumptions in the data because it’s very easy to do and to gloss over that.
Data science is such a hot field right now, it’s exciting and it's changing fast. I think the most important part is to keep an open mind and also not discount assumptions in the data because it’s very easy to do and to gloss over that. A lot of the time you just want to take the data and just slap a model on top of it, and say here are the outcomes and here are the results, but it’s much more nuanced than that. Really so much of it involves the prep stages, going back to the old 80/20, so much of it involves cleansing the data, searching for outliers, fitting the right distribution and really understanding the shape of the data. I think that the shape of the data is the most important part. And I think that once you understand that and are able to keep a very open mind and perspective about the data, the assumptions, the tools available, the model to use, and then you’re not just following the crowd but discovering original insight - that’s the exciting piece. And it all stems from understanding the shape of the data and keeping an open mind.
We are very grateful to Skylar for his time. You can follow him @skyyhigh
An American Entrepreneur, Anna Anisin was named a Tech Industry Insider by CNN three years in a row. Anisin was appointed as a CEO for 4Sync and VP of US Ops at 4Shared the fastest growing cloud storage provider of 2012. After stepping down from 4Sync Anna Co-Founded Passare, the number one collaboration software in the funeral industry. After exiting Passare Anna joined the founding team at Domino Data Lab to assist building the most powerful enterprise data science management platform on the market. Currently Anna is running a boutique B2B marketing firm, Formulated.by, and is the founder of the leading data science community and event series, DataScience.Salon.
RELATED TAGS
Subscribe to the Domino Newsletter
Receive data science tips and tutorials from leading Data Science leaders, right to your inbox.
By submitting this form you agree to receive communications from Domino related to products and services in accordance with Domino's privacy policy and may opt-out at anytime.