Lessons from 20 Data Science Teams
Nick Hotz2019-02-13 | 4 min read
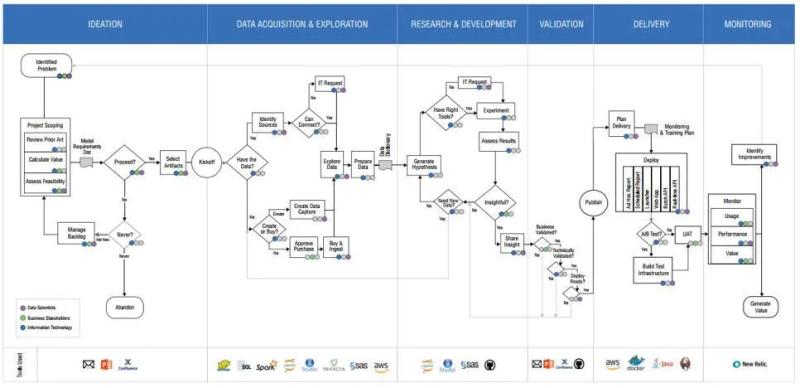
What can you learn if you observe data science teams across 20 large companies?
I asked Mac Steele, Director of Product at Domino Data Lab, to find out. Mac combined the lessons he learned from observing data science teams with concepts from CRISP-DM and agile to create the Domino Data Science Lifecycle. It is defined in a 25-page whitepaper, The Practical Guide to Managing Data Science at Scale.
Motivation to Create a Different Methodology
Early in his career, Mac realized that software methodologies like Scrum when applied to data science “didn’t work so well because things didn’t magically happen in two-week blocks in the same way…you didn’t have incremental functionality that could be shipped and could be observed by the user every two weeks.” He found that “a very traditionally enforced Scrum process seemed more often unhelpful in grading on the data science workflow than helpful.”
More recently while working at Domino, he likewise found several customers struggle to successfully execute data science projects. The “bookends” of projects, namely the inception phase at the start and the monitoring phase at the end, were particularly challenging, in part because existing methodologies did not properly define these phases. Moreover, team role definitions were lacking. Together, this led to ineffective scenarios where “data science does something and then there was a hard wall, and they lobbed their work over to IT who wound up re-writing it,” but did not understand how to monitor and maintain the productized models. Rather to be successful, “you should have IT, data science, and business involved from the beginning” with each team taking on well-defined roles from ideation to product retirement.
Mac acknowledges that Domino’s methodology, CRISP-DM, Microsoft’s Team Data Science Process, and other methodologies are “not all that different because there are several non-negotiable dimensions in data science. The difference is where people add emphasis and the different dimensions they bring. The dimension we tried to bring in was the people, process, and technology dimension.”
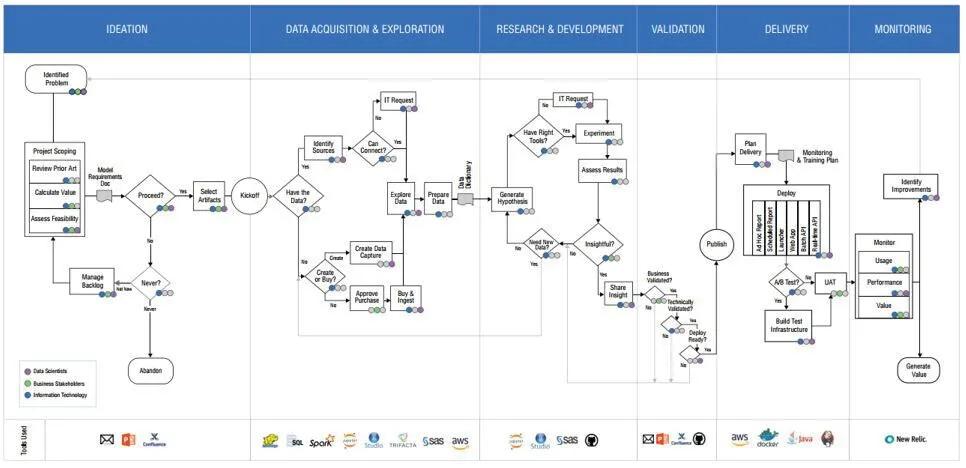
Domino Data Lab’s Lifecycle Methodology. See the last page of their whitepaper for full-scale version.
Future Trends
I asked Mac what he would do differently if he were to re-write an updated version of the methodology and whitepaper. He responded that “Last year I’ve seen and heard a lot more around better checks for bias and fairness – like good data science ethics checks – that are going to become standard operating practices, regardless of whether or not they are regulated, because consumers are going to increasingly demand that.”
Moreover, when asked about the future of data science project management, he responded that “people will learn a lot more from academia and research organizations because they’re going to start realizing that what they’re doing is fundamentally research […and…] that you’re taking on projects that probabilistically won’t succeed and that is okay. Companies like pharmaceuticals and other medical research companies have been doing this for a long time, and I bet people are going to come to learn more from them.”
Similarly, he sees the concept of product management maturing as a distinct sub-discipline for the data science function, much as it is in software engineering. “Product management in the software world is very much responsible for conceptualizing the problem and coming up with the shape of the solution and then on backend working on user adoption and user engagement and driving the value you hope for. Right now, we have data scientists playing the role of developer and product manager and I don’t think that that’s going to last.”
Time will tell, but I think he’s right.