Key takeaways: Gartner Data & Analytics Summit 2025
Domino2025-03-13 | 5 min read
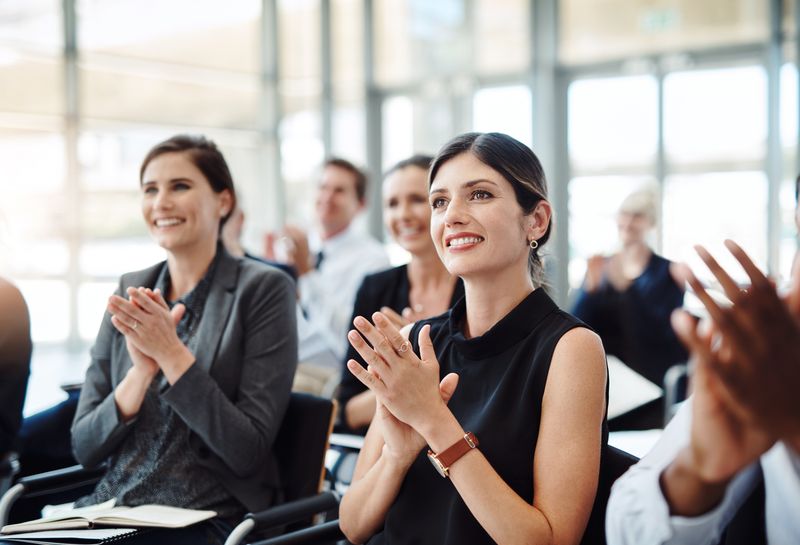
This year’s Gartner Data & Analytics Summit gave analytics, data, AI and data science leaders a forum to share ideas and insights vital to developing and validating their enterprise AI strategies.
Gartner organized the summit along five tracks:
- Leadership: Developing talent and maturing data-driven cultures by building trust
- Artificial intelligence: AI strategies, responsible AI, risk and governance, LLMs, data science, machine learning, and scalability
- Analytics: Hard resets to drive modernization, modern dashboarding, and more
- Data management: Data fabric, products, and observability; resilience and reliability of data management architectures
- Data and analytics governance: Alignment, risk, trust, privacy, ethics, literacy, and accountability
For each track, Gartner analysts led sessions to discuss the latest developments in their domains of expertise. For example, Dr. Maryam Hassanlou covered AI explainability, a crucial technique for fostering trust and responsible AI. It adds transparency to decision-making processes, enabling accountability and mitigating biases. Research VP, AI Strategy, Svetlana Sicular showed how an AI governance operating model can map governance pillars to key AI components and differentiate AI capabilities.
Governance as an enabler
Summit keynote speakers Carlie Idoine and Gareth Herschel further reinforced a crucial theme: governance is not a constraint — it’s an enabler. The move from a “tech stack” to a “trust stack” ensures data integrity while optimizing performance, cost efficiency, and strategic impact. As Herschel memorably put it, “If AI is the frosted deliciousness we want to eat (a cupcake), governance is the kale salad.” The Gartner Data & Analytics Summit also emphasized how trust is the foundation of AI success. Without governance and reliability, trust erodes, and AI adoption falters.
Data readiness is essential for AI success
Beyond governance, data readiness emerged as a defining factor in AI adoption. Businesses often focus too much on data quality when they should be prioritizing readiness — ensuring data is fit for purpose. Another critical takeaway was the need for organizations to shift their mindset from single-use cases to “reUSE cases,” where data is leveraged multiple times for greater efficiency and value generation.
An integrated AI strategy
The CDAO’s role is rapidly evolving, and with AI being a critical enterprise asset, leadership alignment is paramount. AI is no longer an isolated function – it must be embedded across every business unit. Organizations that champion storytelling, cultural transformation, and data reUSE strategies will lead the charge.
Top enterprise AI trends
Domino Data Lab’s team at the Summit included CMO Thomas Been. In an interview with Ravit Jain of the widely followed The Ravit Show, he shared the four most important trends according to customers:
- Governance: This is the #1 challenge facing enterprise AI leaders. They have to modernize antiquated governance methods to keep up with new technologies, evolving regulations, and a rapidly expanding set of use cases. This modernization is essential to unlocking the full potential of AI.
- Upskilling: Companies have tremendous pools of non-data-scientists who have learned the ease of coding with Python. They also have many legacy scientists who want to go beyond tools like SAS and learn new technologies. Smart enterprises are building new onboarding programs in enterprise-grade AI platforms that can seamlessly integrate these diverse tools.
- Hybrid cloud environments: With increasing pressure to realize ROI from big investments in AI, enterprises are challenging their cloud-only deployment strategies. They often have huge volumes of on-premises assets that are simply too expensive to move to the cloud, yet they want to push their workloads closer to where the data is. This is particularly important when it comes to meeting data sovereignty requirements. Hybrid cloud environments are often the answer.
- Open always wins: The successes of ventures such as Mistral, Llama, and DeepSeek are proving the power of an open source approach to AI development. Making code and models publicly available increases collaboration and transparency and drives faster innovation. It also helps reduce vendor lock-in to help organizations of all sizes build state-of-the-art solutions.
Been and Jain also discussed emerging use cases such as life-science researchers using statistical computing environments to discover new drugs and financial groups rebuilding whole risk-management processes with AI models.
Thank you!
Thanks to all of the Gartner analysts, Domino users, and friends that took the time to say hello and connect with us at the show including Rita Sallam, Aura Popa, Adam M. Ronthal, Yogesh Bhatt, Deepak Seth, Lauren Kornutick, Peter Krensky, and Maryam Hassanlou.
Domino Data Lab empowers the largest AI-driven enterprises to build and operate AI at scale. Domino’s Enterprise AI Platform provides an integrated experience encompassing model development, MLOps, collaboration, and governance. With Domino, global enterprises can develop better medicines, grow more productive crops, develop more competitive products, and more. Founded in 2013, Domino is backed by Sequoia Capital, Coatue Management, NVIDIA, Snowflake, and other leading investors.