Tackle the three “R”s of trustworthy AI for ethical, legal and reliable models
Kjell Carlsson2022-04-27 | 8 min read
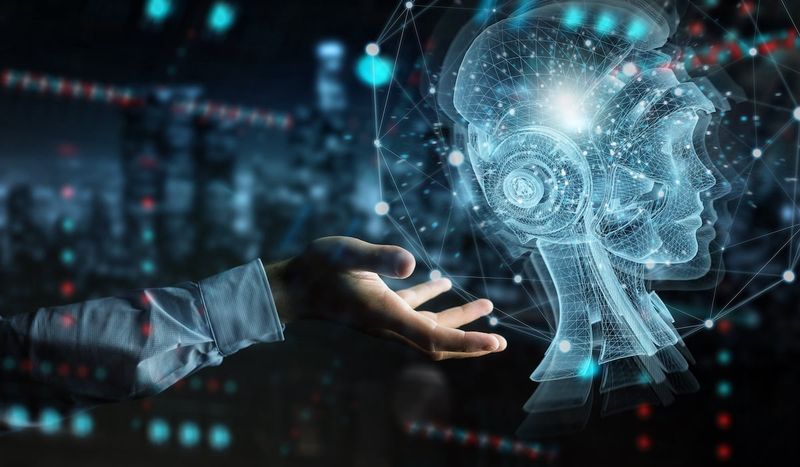
By Kjell Carlsson, Head of Data Science Strategy & Evangelism at Domino on April 27, 2022 in Perspective
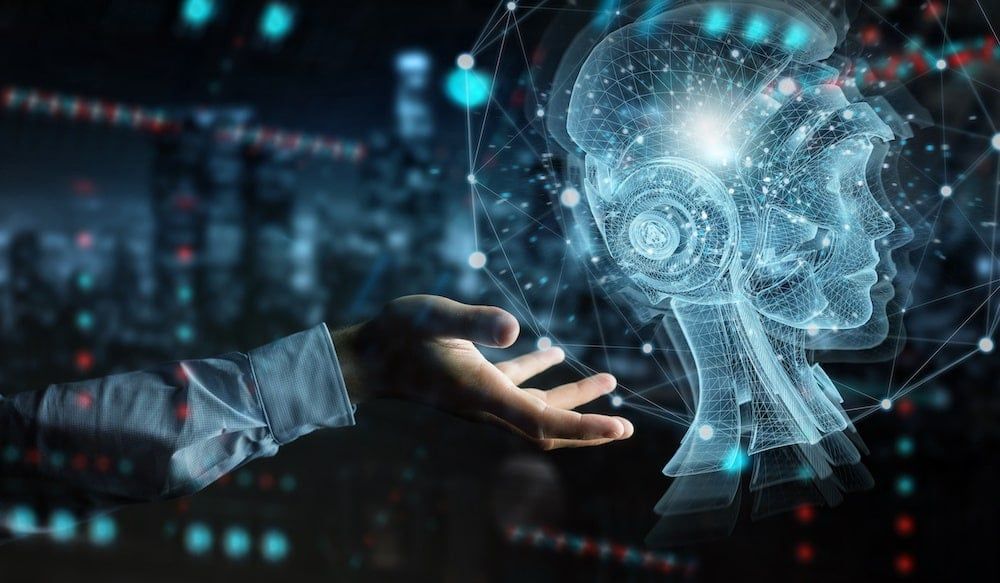
Your business Results, Reputation and Regulatory compliance depend on trustworthy AI
Despite the attempts by popular fiction (and Elon Musk) to convince us otherwise, we do not face a threat of an AI-induced apocalypse. Worrying about rogue AI remains, as Andrew Ng put it, like worrying “about the problem of overpopulation on the planet Mars.” Instead there is a growing consensus that the real AI threat to guard against is untrustworthy AI. In a recent survey, a full 82% of data science leaders said that executives need to worry about the severe consequences of the bad and failing models that underpin untrustworthy AI solutions.
Untrustworthy AI already causes real – though usually hidden – damage. In the same survey, 46% of data science leaders said that they lost sleep over the bad decisions and lost revenue of these models, and 41% pointed to the risk of discrimination and bias. Worse, the risks to your customers, employees, and your enterprise are almost certainly growing as you scale your own and third-party AI solutions.
Trustworthy AI and – by definition, trustworthy ML– is about the very real challenge of ethical, legally compliant, and above all, reliable AI solutions, through the proper design, development, and maintenance of the machine learning models that power them. Let’s explore the reasons trustworthy AI is so important, and the challenges you will need to overcome to ensure it. (Also, come attend the Rev 3 panels and presentations on this topic on May 5-6.)
The three “R”s of trustworthy AI
Outside of data science, few executives have understood the extent that trust is important for their organization’s ability to take advantage of AI. Financial services (finserv) and insurance firms know the importance of trustworthy AI, in the form of regulatory compliance, such as the rules surrounding credit risk scoring. Security firms have been keenly aware of upcoming regulation, particularly around the use of facial recognition by law enforcement. And, everyone has seen the embarrassing incidents in the press where AI solutions have gotten into trouble - think Microsoft Tay’s racist outbursts or Google Photos’ tagging people as gorillas. However, these touch on just a fraction of the ways in which trustworthy AI is crucial for the AI operations and ambitions of every organization. All are affected by the three “R”s of trustworthy AI.
Results
Untrustworthy AI solutions that have undergone less validation, robustness testing, and examination of the drivers of predictions, deliver worse business outcomes than trustworthy ones. Why? Because they perform worse, are more likely to fail in the future, and are less likely to be adopted. Additionally, unfair AI models are usually taking shortcuts by using discriminatory information such as ethnicity and gender and are less accurate than models that have been trained on actual drivers of behavior. Further, untrustworthy AI solutions slow innovation because they fall under suspicion and pushback from stakeholders throughout the process of development, implementation, and adoption.
Reputation
When AI solutions are found to be unfair, unreliable or even illegal, a company takes a hit to its reputation, affecting the willingness of customers to do business with the firm and of talent to apply for or stay in their positions. That most of the notable blunders have come from tech companies like Google, Facebook, Microsoft and Amazon, should not be taken as a sign that others are less at risk. These firms just started their AI journeys earlier, and use AI models more. However, they can also weather the reputational impact of high-profile mistakes – because they are effectively monopolies in their core businesses. Your competitive business, which has spent a fortune and many years building up your relationship and reputation, probably does not have that luxury. An internet giant will not have a problem recruiting even when its recruiting tool turns out to be sexist. Don’t count on your organization being so lucky.
Regulation
Outside of a limited (albeit highly valuable) set of use cases, predominantly in financial services, which have been heavily regulated since long before AI came on the scene, we have mostly been living in an anarchist’s paradise in terms of AI regulation. There has been strong data regulation in the EU, in the form of GDPR, which indirectly affects AI use cases that leverage customer data. Plus, China introduced sweeping AI legislation last month, though its implications remain unclear. In the US there has been no federal, and only minor city and state level legislation on AI, much of which has been on the use of facial recognition and customer data. However, regulation is coming. The EU has proposed extensive AI regulation – with fines up to 6% of global revenue – and the US is expected to introduce milder federal AI legislation in the near future.
There is no panacea, but there is a growing ethical AI & ML toolkit
Though most firms almost certainly underestimate its importance, there is an overwhelming consensus that trustworthy AI is important. That same consensus doesn’t exist on how to bring it about. Articulating a set of ethical AI principles has been popular - such as the ones postulated by Microsoft, IBM, Google, Deloitte and the Vatican. Unfortunately, many of these sets of principles provide little guidance to practitioners, contradict themselves, and are often remarkably disconnected from the actual technology and its applications.
There is also, unfortunately, little evidence that the AI ethics advisory boards that companies have been establishing have provided sound, practical guidance. Few individuals with expertise in AI technologies seem to have been involved in either set of endeavors. It is remarkable, for example, how many of these entities have called for absolute customer data privacy while simultaneously insisting on eliminating all harmful bias. This misses the fact, immediately obvious to any practitioner, that you cannot remove a discriminatory bias without the customer information to detect or mitigate it.
Trustworthy AI does not come about by decree from an AI ethics board, but ultimately relies on the hard work and constant vigilance of the teams involved in developing and maintaining these AI solutions. Thankfully, there is an ever-expanding toolkit of methods that practitioners can use to improve trustworthiness. These range from explainability techniques, fairness tests, and methods for bias mitigation to the always important traditional methods for model validation and monitoring.
Practitioners will invariably need to use a host of these techniques, depending on the context and use case, because of the many different dimensions of trust itself. Platforms (such as Domino Data Lab’s) that make it easy to apply the full range of these tools, implement new methods as they become available, ensure model reproducibility, and facilitate ongoing monitoring are vital to ensuring trust. But having the people – with an understanding of data science, trustworthy AI methods, and ethics – and processes – to consistently document, evaluate, test and monitor your solutions – is critical. As with everything in artificial intelligence, human intelligence is the most important ingredient.
For more on how to ensure that real world AI solutions are reliable, ethical, and comply with new and future regulations check out the sessions at Rev 3.
Kjell Carlsson is the head of AI strategy at Domino Data Lab where he advises organizations on scaling impact with AI technologies. Previously, he covered AI, ML, and data science as a Principal Analyst at Forrester Research. He has written dozens of reports on AI topics ranging from computer vision, MLOps, AutoML, and conversation intelligence to augmented intelligence, next-generation AI technologies, and data science best practices. He has spoken in countless keynotes, panels, and webinars, and is frequently quoted in the media. Dr. Carlsson is also the host of the Data Science Leaders podcast and received his Ph.D. from Harvard University.
RELATED TAGS