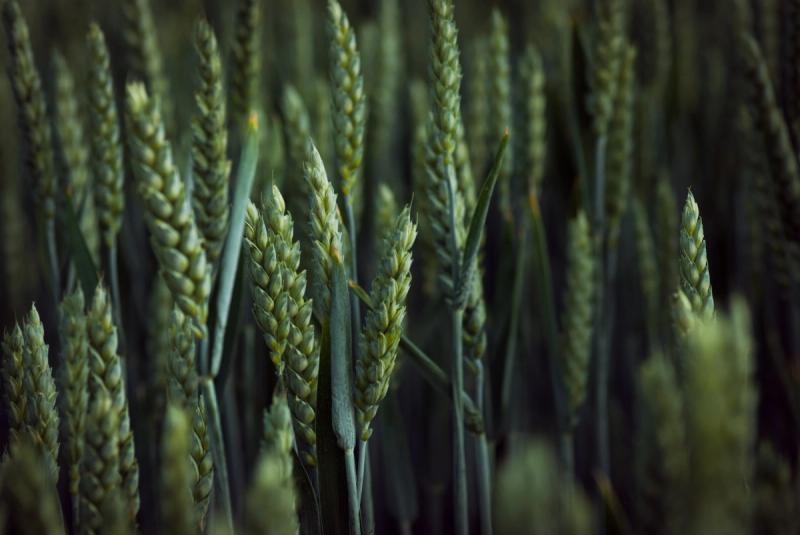
By Brian Loyal, Cloud Analytics Lead, and Naveen Singla, Vice President of Data Science, Bayer Crop Science
Editor’s note: This is part of a series of articles sharing best practices from companies developing an enterprise data science strategy. Some articles will include information about their use of Domino.
A few years ago, we joined the ranks of a growing number of firms creating a Center of Excellence (COE) for data science. One Deloitte survey found that more than one-third of large firms have a Center of Excellence (COE) or competency center. McKinsey put this figure as high as 60 percent among companies that are successfully scaling advanced analytics.
At the time, data science efforts were burgeoning across Bayer Crop Science, with business units building out their cadre of practitioners so they could take advantage of advanced analytics and machine learning capabilities. But as more and more data science use cases sprouted across different corners of the business, we wanted to ensure we had the right guardrails in place. It led us to reevaluate how we managed data science from an organizational perspective and, ultimately, to create a COE to help build out an enterprise strategy for governing data science. Since then, our COE has expanded its charter to cultivate common capabilities and best practices for enabling data science at scale.
Ultimately, what we learned was there were a lot of advantages to having a group focused on problems that span the org and best practices.
Listen to Brian Loyal discuss why Bayer Crop Science launched a COE.
What are the advantages?
For us, these advantages include, among others:
- Greater sharing of knowledge across data science teams. For example, we’ve observed increased reuse of code and models among practitioners that is helping accelerate the development of new data science products.
- Increased efficiency in data science as the COE works on solving overarching challenges that cross multiple business units, such as making it easier for practitioners to mine genomics data.
- Better alignment of data science and business strategy with investments focused on the data science capabilities that leaders are trying to enable.
- Improved talent acquisition, with the focus on a data science community and knowledge sharing attracting the attention of high-performing data scientists.
What lessons did we learn along the way?
Standing up a COE, or any type of hub for that matter, isn’t easy. In our conversations with others working to implement or expand their enterprise data strategy, we typically recommend the following:
Wait until you have a critical mass
While we believe a “hub” (in our case, the COE) is important for governing data science from an enterprise perspective, don’t set it up from day one. Let the practice grow a bit, then see when you’re at the critical mass that needs a governing body.
Here at Bayer Crop Science, more than 200 data science practitioners were working within the business units when we began building our COE. Our initial strategy focused squarely on a growing concern among company leaders, which enabled us to gain traction more quickly than otherwise possible.
Think about how to add value
Once our COE tackled the immediate issue of governance, we then turned our attention to identifying ways to accelerate the value of data science. We called it our “COE reboot” and created two key operating teams to support our efforts:
- A vertical team aligned to key business units, such as Research and Development, Supply Chain, and Commercial, to help them meet their revenue and cost reduction goals. Here our COE staff mainly identify and deliver overarching data science capabilities, such as predictive pricing models or genomics models, that might benefit multiple business units or functions. In cases where a business line doesn’t yet have a data science and analytics team in place, our COE will fill the gap to help with LOB-specific data science projects while LOB leaders begin building out their own data science teams.
- A horizontal team tasked with building foundational capabilities and practices that can benefit everyone. This has included implementing the Domino data science platform to make it easier for siloed teams to share data, code, and models and instituting community building practices to further encourage collaboration. We feel this emphasis on community is vital and has had a multiplying effect. For example, spontaneous meetups and forums among practitioners company-wide have now become commonplace, creating a self-sustaining cycle of collaboration and knowledge sharing.
Listen to those in the trenches
Often, there can be some friction between centralized teams and the business as federated teams worry that corporate edicts will stand in the way of business goals. To combat this, we took both a top-down and bottom-up approach. This included creating a Data Science Council that brings together data science leaders and senior data science staff from across the company to shape the future of data science within the organization. This council ensures that data science teams within each business have a say in new processes and feel a sense of ownership in decisions, which helps ensure adoption of new capabilities and get over the inevitable bumps in the road when concerns arise about standardizing processes and practices.
Measure your success
This is critical to elevate the value of data science in general and the work of the COE in particular. We track a number of different metrics including:
- Adoption of data science to drive better business outcomes, such as the number of models deployed to drive business decisions and the number of business decisions being made based on data science.
- The financial impact of models where possible. In some areas, like R&D, where it can take up to seven years for a new product to make it to market and deliver a financial return, we look beyond traditional return on investment. For example, we often focus on the “hit rate” for R&D projects—how many products that enter the pipeline are successful at each step—with an eye for continuous improvement. We want that number to go up year-over-year, and we’ve seen a positive trend over the past few years.
We also conduct an annual survey with anyone doing or enabling data science work at the company to get input on what’s working and what’s not. These surveys have uncovered surprising insights that have led to changes in how we do things.
There’s no silver bullet solution to getting data science to scale. Still, we’re finding that having an enterprise data science strategy is helping us get there faster. And these practices, among others, can help smooth the way.
Watch the webinar, “Best Practices for Driving Outcomes with Best Science” where I (Brian) discuss more best practices along with Matt Cornett, Director of Data Science for a leading provider of insurance solutions, and Patrick Harrison, Director of AI Engineering for a global financial intelligence company.
RELATED TAGS
Subscribe to the Domino Newsletter
Receive data science tips and tutorials from leading Data Science leaders, right to your inbox.
By submitting this form you agree to receive communications from Domino related to products and services in accordance with Domino's privacy policy and may opt-out at anytime.