Scaling data science rapidly: A conversation with CSL Behring’s John K. Thompson
Karina Babcock2020-11-02 | 11 min read
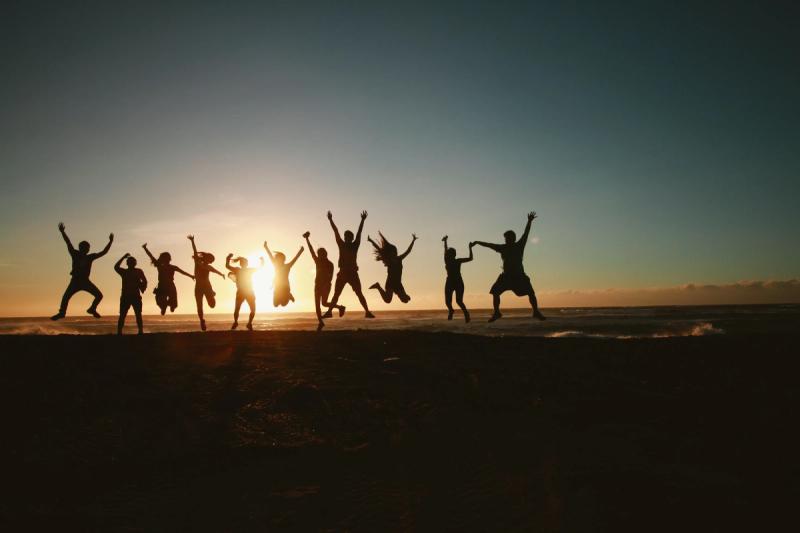
Data science’s impact has become increasingly clear in every industry as leading adopters raise the bar for personalized services and customer experiences. IDC’s recent survey on AI found that early adopters reported a 25 percent improvement in customer experience along with accelerated rates of innovation and higher competitiveness, among other benefits.
However, most leaders are realizing that to bring AI to scale, they need an enterprise strategy that includes:
- Creating a discipline for how they’ll manage the people and cultural changes necessary to embrace data science.
- Establishing scalable and repeatable processes across the end-to-end data science lifecycle.
- Ensuring data science teams have the technology foundation to foster productivity and collaboration.
Take for example CSL Behring, a leading global biopharmaceutical company that specializes in treatments for rare diseases, such as hemophilia and immune disorders. In 2018, company executives set out to turn data science into a core capability that would help scientists:
- Uncover new life-saving therapies
- More quickly bring products to market, and
- Help reduce the time for patients to receive an accurate diagnosis, which can take years as patients and caregivers struggle to make sense of a variety of vague symptoms that often overlap with other, more common conditions.
In building out the capability, executives wanted to move quickly–reflecting on their promise to
“work as if lives depend on it, because they do.”
To bring their vision to life, they hired John K. Thompson, a 30-year industry veteran in business intelligence and advanced analytics, as the company’s first Global Head of Advanced Analytics & Artificial Intelligence.
In just two years, John has created a thriving and successful advanced analytics and AI practice across all areas of the business, from research and drug safety to manufacturing to supply chain to operations, generating immeasurable benefits for patients struggling with rare disease as well top- and bottom-line business value.
We recently spoke with John about his ability to achieve so much in a relatively short period of time. Below is an edited transcript from our conversation.
When you joined CSL Behring, where did you start?
When I first came in, I built a Center of Excellence (COE), hiring a group of data scientists and augmenting them with data visualization experts and developers that could go anywhere in the corporation and do advanced analytics projects for any interested line of business or operational area.
Once I had this team together, I needed to get the word out. We started publicizing through internal emails and then setting up meetings. During the first year, I had over 1,100 meetings with everyone from C-level executives to frontline workers in our factories, talking about advanced analytics and artificial intelligence and what it could do.
More than 400 people signed up to be part of our mailing list in the first year, and we connected with 80 people who were embedded in different business units already doing data science work.
From there, we looked for ways that we could come together on a regular basis. We held the first-ever Data Science Summit for the company in Bern, Switzerland where people from all over CSL discussed what they were doing, how they were doing it, and what they wanted to do.
We also created Special Interest Groups (SIGs) based on the feedback we received. We have SIGs on everything from using AI for drug safety to image analysis to R and Python. For each of these groups, there’s an “owner” and they have regular meetings – which occur usually between every month and every quarter.
These groups now feed their interests back to the COE and we feed that back out to the Community of Practice to create an ecosystem to guide what needs to happen around the organization. These three structures allow people to have small enough, cohesive conversations whereby they can make progress, and a large enough community that can have impact.
Why a COE?
The Center of Excellence is a great concept that works well for many different kinds of organizations.
First of all, it gives organizations clarity around where they can ask questions. I have many executives, operational managers and subject matter experts coming to me asking:
“Hey, I’m thinking about doing X. Do we have any technology in this area? Have we done it before? Have we done anything similar?”
Having a COE gives people clarity on where they can go to ask these questions.
Second, it provides a focus on the technology to help the organization understand what’s possible with AI and what isn’t. There are some things that AI can currently predict with a great deal of certainty, and some things it can’t. So it’s a place of expertise to navigate this.
Finally, the COE provides an anchoring point to help bring together people from IT, operations, and other disciplines that are vital in bringing this work to fruition.
Line of business staff can sometimes be skeptical of centralized teams. How did you build support for your COE?
We made it very clear from the beginning that we were happy to help do projects on our own for other people, as consultants, but we were also happy to provide advice and support to those that were already doing data science projects. So we have people that come to us and ask for a data scientist or data visualization person to fill in a gap, and we can loan them someone from our team. And they come to us for expert advice on the best technologies to use to solve a challenging problem.
Hiring is always challenging. How did you build up a team from scratch?
You need to take your time and know what you’re looking for. My view is you need a team with a few senior, PhD-level data scientists, and the rest can be talented up-and-comers. So, we just started putting it out there and we were quite pleasantly surprised with the quality and the volume of the people that came forward and said they wanted to work with us.
We also have five or six interns working with us at any one time because we like to hire right from either undergraduate or graduate levels. We have a relationship with a number of universities: the University of Michigan, University of Texas at Austin, Drexel, and Oklahoma State University to name a few. And we have an intern come back two or three times before making a hiring decision. We want to have people around for a long time to make sure it’s a good fit. And it pays off. Of our first six hires from 2018, for example, five are still with the team today.
How do you set your priorities?
From a use case perspective, we look at the business, and where the biggest need is right at that moment to make a difference for patients and our company. For example, with COVID-19, we had to shift our focus to help ensure we have enough plasma donations. Since everything that we do comes from human plasma, anything that causes people to not leave their house and come to the donation center can create challenges across all areas of the business. So we’ve been focused almost exclusively in the last six months on the plasma business, understanding donors and their concerns and how to respond.
From a process perspective, we generally focus on building systems and containers to go into production environments. Once a new model goes over to production, IT then takes over, handles the infrastructure, compliance and regulatory compliance. We retain authority and responsibility for the models themselves, monitoring model performance and retraining models when needed.
What advice would you give to others starting to build a COE?
First, as I mentioned earlier, you have to hire well. You need people on your team who can collaborate. Someone who can’t collaborate and cooperate with the other team members is going to erode team cohesion and productivity. And all individuals need to have a sense of humility. If something isn’t right, we need to be the ones to raise our hand and correct it.
Second, I believe the COE is an environment for creative professionals. It’s not a technology team, it’s not an IT team (and I think it’s a bad idea for COEs to report to IT given the differences). We’re not building the next ERP system or CRM system. We’re taking many sources of data, and we’re mashing them together, and we’re trying to come up with new insights around what the possibilities are. Thinking about data science as a creative endeavor allows data scientists to investigate things that they’re interested in. And sometimes you’ll get nothing out of it. But other times you change the way the business operates. So inside our group, while we’re constantly working to serve the needs of our line of business users and executives, we have a very free-flowing, experimentation-based approach.
Finally, the COE has to be able to effectively set expectations with subject matter experts, managers and executives who may not know what’s involved in an advanced analytics project and what it takes to get it done. So it’s important to set expectations that they’ll get the groundbreaking work they want, but it can take time.
That said, we still want to be a nimble organization to help subject matter experts understand their business in new ways, as quickly as possible. I want to show that these things don’t have to take weeks and months. So we iterate quickly with subject matter experts, and then once a model is built, we slow the cycle down for governance and production processes. And I do believe that having a nimble approach to building models really gives enjoyment and excitement to not only the data science team, but the people in the business.
Learn more
- Read John’s books – Analytics: How to win with intelligence and Building analytics teams
- Tune into John’s fireside chat with Domino’s Chief Customer Officer, Dave Cole, on the topic of Building Your Best Data Science Team.
RELATED TAGS