How a 90-year-old insurer is pushing the innovation pedal
Karina Babcock2019-12-09 | 6 min read
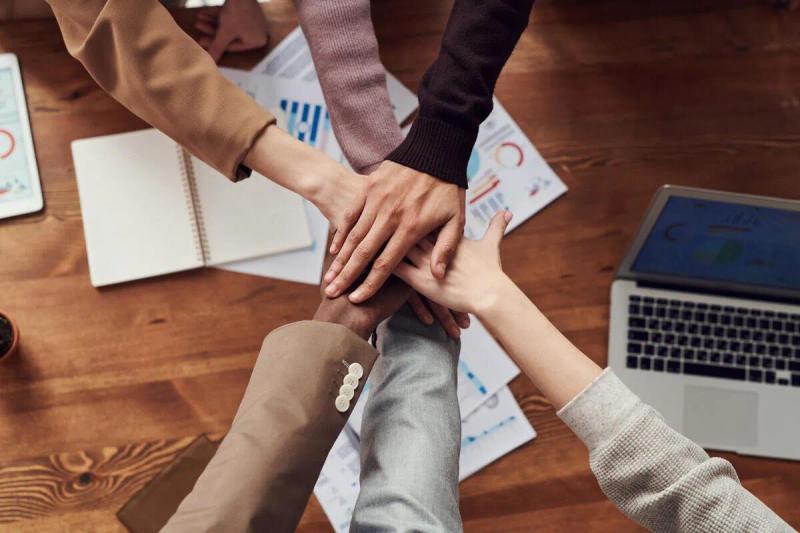
Allstate’s journey to becoming model-driven
Editor’s note: This post is part of a series of articles sharing best practices from companies on the road to becoming model-driven. Some articles will include information about their use of Domino.
Many people may be surprised by Allstate’s vast and innovative use of models. Often companies with such deep roots and deeply ingrained ways of doing business are hesitant to alter the status quo.
But instead of defaulting to business-as-usual, this leading US insurer has made significant inroads using data science to drive what Chief Data and Analytics Officer Eric Huls calls a “step-change” in capabilities for the more than 16 million households Allstate serves.
“We are creating more consistent, positive interactions for our customers and fundamentally transforming insurance,” says Huls. “Our vision is to shift from merely making things right when bad things happen to finding ways to prevent those adverse events in the first place.”
Already, the company uses models to inform marketing efforts, support claims processing, help produce quotes, and predict thousands of decision-making actions throughout every part of the organization, including products, sales, operations, marketing, and claims.
So how has Allstate’s data science team pushed the pedal on innovation so successfully?
According to Huls, two efforts have been key:
- Breaking down barriers to change by building strong relationships with business units.
- Empowering data science teams with the tools to more easily test new ideas and develop models.
Engaging business leaders in analytics
Allstate has pursued a proactive and pragmatic process to engage the business in becoming model-driven. It is an extensive, multi-pronged effort that includes, among other things:
- Investing in staff training and expanding hiring criteria to ensure Allstate’s data science experts have the “soft skills” and business knowledge to present their work in a way that makes sense to the business.
- Investing in education for business leaders. “We launched educational opportunities and communities across the company,” says Huls. “We also set up informal talks with industry experts so Allstate officers could learn about cutting-edge data science techniques and how they could advance the company’s strategy.”
- Hiring business analytics consultants to support initiatives. This newly created role gives data science teams a front-row view of the core business problems and landscape to ensure they work on issues meaningful to the business.
- Integrating data science teams within business units. Allstate’s centralized analytics team (known within the company as D3—the Data, Discovery, and Decision Science team) mirrors and integrates with Allstate’s larger organizational structure. Each data science team has dotted-line responsibility to its assigned business unit, and team leaders actively participate in senior leadership discussions.
- Co-creating a vision, plan, and roadmap with each business unit. This joint ownership reinforces the importance of data and analytics in Allstate’s future and ensures business adoption and support when it comes time to put models into production.
- Tying analytics initiatives to business outcomes and results. “At the beginning of any project, we ask how we will measure whether a model has the intended effect,” explains Huls. “If we can’t measure the value in business terms, we don’t move forward on the project.”
- Building products, not models. Data science teams consider all the capabilities needed for deployment, such as creating feedback loops to make reporting and tracking model performance management easier for the business. “We find when we focus on the bigger picture, adoption skyrockets and there’s greater interest in refining and improving upon the models once we get them in place,” says Huls.
Effectively managing model research and development
As the demand for models grew across Allstate, and the D3 team expanded from 30 to 300 data science experts, they needed a tool to help better manage model research and development. “The less time we spend relearning or recreating results, the more time we can spend creating additional value and the more projects we can support,” says Huls.
Here, the Domino platform delivered the capabilities they needed, including:
- Ensuring data scientists could quickly access tools and technology resources. Most data science teams know the drill. Submit a request for a software tool or compute resources, and then wait. With Domino, the company’s data scientists now have one-click access to the compute infrastructure and software tools they need, including R, Python, and SAS. so they don’t have to wait. And new team members can take advantage of pre-built compute and tool environments so they can get started much more quickly.
- Improving collaboration among data scientists. Allstate’s data scientists use Domino to collaborate on code development, share feedback, and iterate, and Huls can easily track the progress of their efforts.
- Institutionalizing knowledge, insights, and artifacts. In Domino, everything is now documented– code, environment details, data, and so on for both research and regulatory compliance. As a result, staff can build on past work, answer compliance queries quickly, and review all past results. Ultimately, by eliminating time-consuming processes and strengthening collaboration, Allstate’s D3 team can focus their time and expertise where it matters: finding ways to make a difference for customers.
Learn more
Watch Huls’ presentation, Pushing the Innovation Pedal in a Conservative Industry, originally given at the Rev 2 data science leaders’ summit in May 2019.
View the case study to learn more about how Domino is helping Allstate drive innovation.
RELATED TAGS
Subscribe to the Domino Newsletter
Receive data science tips and tutorials from leading Data Science leaders, right to your inbox.
By submitting this form you agree to receive communications from Domino related to products and services in accordance with Domino's privacy policy and may opt-out at anytime.