How to pump up data science productivity? Re-imagine your workbench!
Domino Data Lab2022-08-04 | 5 min read
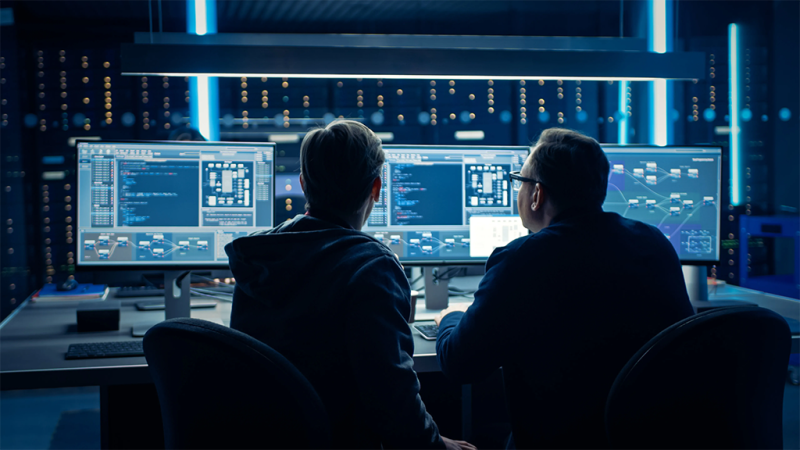
Doing data science productively is akin to the work of a master craftsman: efficiently producing a quality job is wholly dependent on having a unique set of tools, and a workbench that allows the skilled, systematic use of those tools for a custom finished product.
The idea of a workbench makes perfect sense as an invaluable helper for data science practitioners who combine data, code, and parameters to produce models that generate predictive results. The permutations drive enormous complexity that is impossible to manage without a data science workbench.
The biggest challenge for data scientists is doing this work at scale to deploy models into production. One data scientist, or even a small team, can leverage modest workbench capabilities. But large teams of dozens of data scientists working on multiple enterprise-class problems are a whole different challenge. Stakeholders in this scenario need specialized capabilities in a data science workbench. For the emerging model-driven enterprise, Domino has reimagined the data science workbench specifically for creating and deploying models at scale.
Three pillars of a data science workbench
Our new whitepaper, “Pump Up Data Science Productivity with a Modern Workbench”, describes three pillars of capabilities to enable doing productive data science at scale. These are Consistency, Context, and Coordination. Each requires specific features in a workbench for doing productive data science at scale. Addressing these with a scalable workbench allows all stakeholders to have instant access to all the tools and infrastructure to support diverse experiments. The workbench also breaks down silos in which data scientists usually work, providing deep visibility and reproducibility for all aspects of every experiment to all stakeholders. The three pillars enable enterprises to operationalize models into full-scale deployment and manage ongoing improvement in performance of algorithms.
Consistency in using any tool or process required for a model
This pillar is about the consistent application of tools and processes used for conducting data science. Consistency helps ensure logic, accuracy, and fairness in the result, which is creation and deployment of a model. Consistent patterns and practices drive productivity and cost savings. They also enable trust in the models backed by on-demand reproducibility of the output.
Context for collaboration and knowledge acceleration
Collaboration is a vital element of data science, where practitioners work with each other in conducting experiments and developing models. Data scientists collaborate because it can accelerate progress as hurdles are overcome faster. Different approaches to solving problems can trigger better problem-solving. Sharing knowledge can bring new and innovative ideas as practitioners learn from each other.
Coordination of projects to solve complex business problems
Far too often organizations fail to finish the swing on data science projects and the model never makes it into production. According to Gartner, “Through 2021, 75% of AI projects will remain at the prototype level as AI experts and organizational functions cannot engage in a productive dialogue.” The biggest culprits in this failure are a lack of coordination with the business and an inability to govern a large portfolio of projects as initiatives scale. Coordination capability within the workbench is essential for project management and portfolio governance at scale.
A model-driven business is dependent on a foundation of tools and processes that enable teams of data scientists to efficiently create and tune these engines of transformation. To meet this productivity challenge, data science practitioners need help with easing the use of any tool they need to do the job – and to align the efforts of teams such that business leaders can understand and rely on the results of predictive modeling. This is the role of a scalable data science workbench.
To learn more about the three pillars of a data science workbench, read our whitepaper, Pump up data science productivity with a modern workbench, which includes a Top Feature Checklist for workbench productivity at scale to help teams evaluate options.
Domino powers model-driven businesses with its leading Enterprise MLOps platform that accelerates the development and deployment of data science work while increasing collaboration and governance. More than 20 percent of the Fortune 100 count on Domino to help scale data science, turning it into a competitive advantage. Founded in 2013, Domino is backed by Sequoia Capital and other leading investors.
RELATED TAGS