Rev 3 Shows Data Science Really Is Running the World–and MLOps Is Spurring On the Trend
Lisa Stapleton2022-05-06 | 7 min read
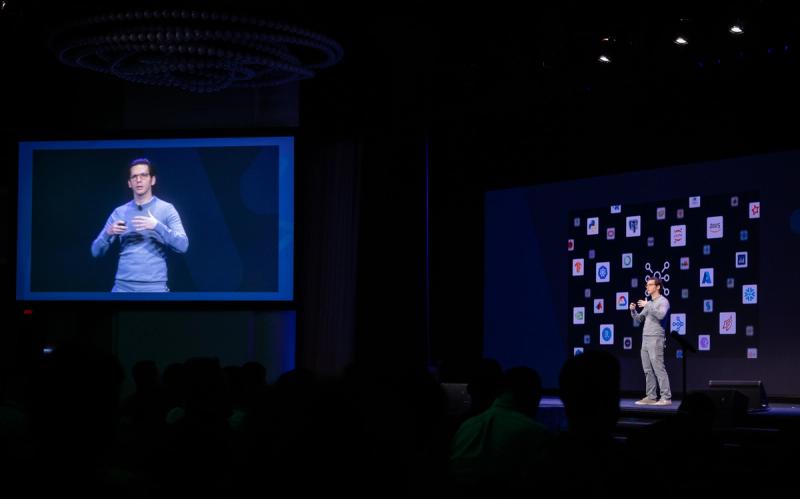
The emerging power of data science to run the world–long partially obscured even to the individual practitioners of the craft–was on full display on the opening day of the far-ranging Rev 3 AI and data science conference in New York City this week, and it felt like the debutante ball for a young profession.
Day 1 of the Rev 3 program featured keynotes from leaders in business, life sciences, computer technology, and of course, data science itself, highlighting everything from the importance of the profession in undertaking critical business decisions, to its impact in particular industries such as pharmaceuticals and finance. To boot, conference organizer Domino Data Lab announced new machine learning operations (MLOps) tools that make it faster and easier to create and maintain the models that make the world go round.
“Always remember there’s a connection between what you do and making the world better. It’s absolutely clear to me, and it should be clear to you,” former SEAL commander and VMware’s chief digital transformation officer Mike Hayes told the audience, which was composed primarily of data scientists, IT pros, and data science leaders gathered for the two-day conference.
“MLOps is a massive uplift in our ability to make decisions,” Hayes said, adding that data science informs decisions made today in virtually every discipline, from business to the military to government, and beyond.
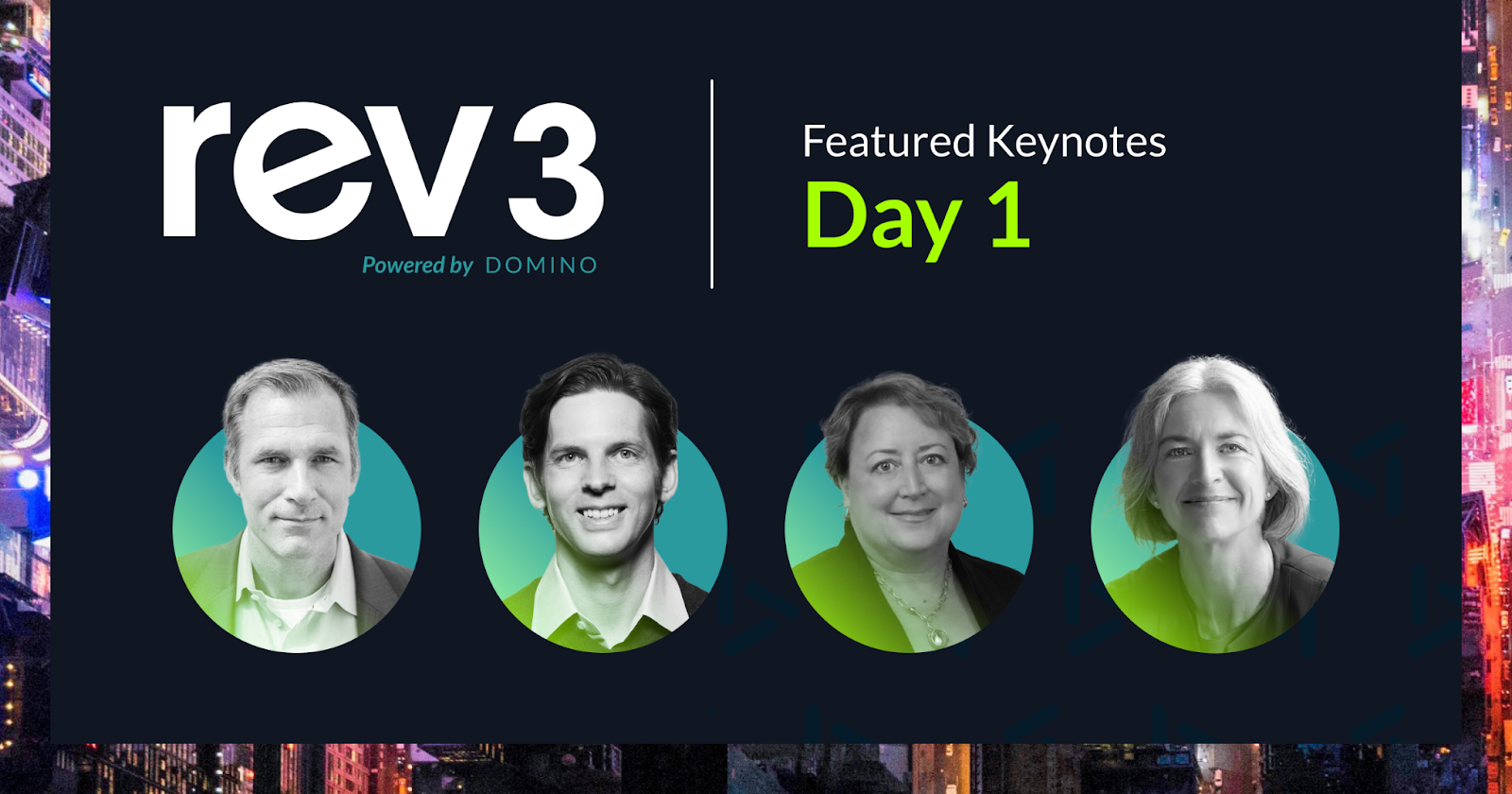
Pandemic, Spectacular Results Fuel Stratospheric Rise of Data Science as a Discipline
The story of keynote speaker Linda Avery, Verizon’s chief data & analytics officer, underscores the almost breathtaking speed of the ascendency of the data science profession in business. A little more than two and a half years ago, Avery was, as she put it, “a department of one.”
Avery set about organizing, staffing, and resourcing a “Center of Excellence” built around data science capabilities, and today commands an organization of more than 1,000 people involved in the application of the discipline to everything from the positioning of cell towers to the staffing of Verizon stores during the pandemic. That group now powers “almost $2 billion in revenue,” she says, and is now a driving force in Verizon’s strategic decision making.
“As horrible as COVID-19 was for the world, it was a great opportunity for data science,” Avery says, as companies like Verizon had to answer critical questions and make predictions using data models and MLOps to remain operational and cope with unprecedented conditions.
Similarly, in fields from finance to pharma and life sciences, relatively new armies of data scientists and technologists in related fields have mobilized to tackle problems that have burgeoned in organizational and technical complexity. This theme was touched on by Nobel laureate Jennifer Doudna, whose work on CRISPR gene editing has revolutionized biotech and the search for cures for serious diseases.
“What’s happened over the last decade is that research in biology has really changed, and is more focused on the kinds of questions that require a complex approach and the need for the expertise of lots of people with different specialties,” Doudna told the Rev 3 audience.
That increased level of complexity often calls for huge amounts of data to crack particularly difficult but extremely important questions.
“You have to get to a certain amount of data understanding before you can get to a point where you realize you’ve got something new,” she says.
MLOps Is Critical to the Success of a Discipline Under Pressure to Deliver Consistently
The successes of data science in so many fields–and its acceleration and enablement of business decisions under the tremendous pressures of the pandemic–have also put pressure on the young discipline to deliver successful insights and outcomes each time, every time.
“Playtime is over for data science,” Elprin said. “If companies can’t turn data science into business impact and describe that impact – if work is still relegated to an AI innovation lab – they are already behind, whether they realize it or not.”
Domino Data Lab’s release of Domino 5.2 is the solution for the problem of accelerating the work of data scientists–while still giving them the freedom to innovate and use whichever tools they need to solve important problems, Elprin told his audience.
As data scientists use more software and compute, IT needs to manage more cost and operational burden. Elprin says Domino's Durable Workspace development environments are now smarter and more efficient, with a new capability called Intellisize that recommends the optimal size for an environment, saving money that would otherwise be wasted on unused resources. For data science teams, this means less complexity and more productivity. For IT, eliminating excess workspace capacity and automatically deleting abandoned workspaces can significantly reduce monthly cloud storage costs.
Flexible model deployment using Snowflake for in-database computation
Orchestrating the movement of data takes custom development work and forces manual workarounds that consume valuable time for data scientists and ML engineers, and introduce unnecessary risk. Domino has partnered with Snowflake to integrate end-to-end workflows across the MLOps lifecycle. Domino 5.2 combines the flexibility of model building in Domino with the scalability and power of Snowflake's platform for in-database computation. Customers can train models in-database using Snowflake's Snowpark, then deploy those models directly from Domino to the Snowflake Data Cloud for in-database scoring – simplifying enterprise infrastructure with a common data and deployment platform across IT and data science teams.
Domino 5.2 Provides a Solution to the Threatening Problem of Model Decay
Finally, Elprin demoed new capabilities for streamlined real-time model monitoring in Snowflake Data Cloud environments, a response to a looming threat of model decay, which occurs when models quit performing as accurately as they did when they were originally tested and deployed. This is usually due to changes in business conditions, customer preferences, and other factors. Without proactive monitoring for data drift and accuracy, companies risk making bad business decisions based on outdated models, particularly in times of rapid change.
With Domino 5.2, data science teams can now automatically set up prediction data capture pipelines and monitoring for models deployed to the Snowflake Data Cloud. Domino will also now continuously update data drift and model quality calculations to drive increased model accuracy and ultimately better business decisions.
Lisa Stapleton is a technology writer and editor in San Jose, CA. She has written and edited for Infoworld, InformationWEEK, LinuxInsider.com, and many other business and technical publications. She is now Domino's Content Director.
RELATED TAGS