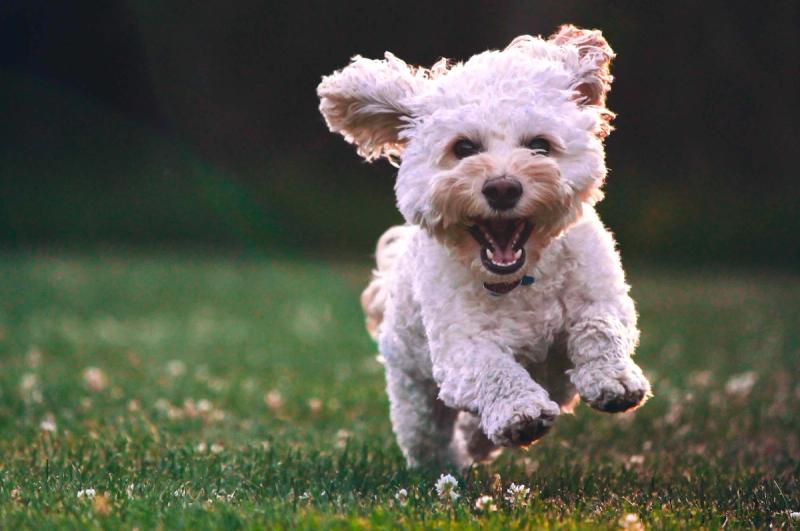
Paco Nathan's column covers themes of data science for accountability, reinforcement learning challenges assumptions, as well as surprises within AI and Economics.
Introduction
Welcome back to our new monthly series! September has been the busiest part of “Conference Season” with excellent new material to review. Three themes jump out recently.
First up: data science for accountability. A controversial metric called Dunbar’s Number describes how many relationships a primate can sustain based on neocortex size. The rough idea sets 150 as a threshold size for a cohesive group. It speaks to the fact that humans aren’t particularly good at making decisions in much larger groups. Data science, however, helps augment that human condition – especially for collaborative data insights across large organizations. And while the dialog about ethics in data science has been going strong for the past few years, we’re beginning to see tangible actions for enterprise: checklists that teams can use, examples of better ways of measuring and modeling, as well as excellent analysis and visualization about issues that compel change. As data science gets applied for corporate and public accountability, issues turn up in unexpected ways.
Secondly: reinforcement learning challenges assumptions. Data science practices are moving out across mainstream business, as Strata NY 2018 demonstrated. At the same time, many “givens” about data analytics, machine learning, and their relationships with engineering are changing rapidly. Reinforcement learning is one big factor that challenges prior assumptions about the field. New kinds of biologically-inspired learning disrupt established practices, decades’ old models with renewed priorities, as well as questions about how much data do we need to build models? In other words, does “more data” necessarily win? Also, learning is proving to be more general-purpose than coding within enterprise IT practices.
Third: AI and Economics have surprises in-store. There’s a lot of public discussion about the potential for AI to displace jobs. Much of the econometrics involved focuses on measures of labor and employment, meanwhile, there are disturbing trends about R&D efforts declining in aggregate. However, some of those assumptions appear to be wrong. Multiple independent research efforts point to problems in the math of those economic models, how AI accelerates R&D in unexpected ways, in turn boosting capital stocks (in the economic sense, not company valuation). Also, the economics of newer hardware, newer cloud architectures, faster networks, etc., imply some movement away from the era of data practices based on Hadoop/Spark. These factors have profound implications on industry, where data science and data engineering provide foundations for a sea change.
Data Science for accountability
Strata NY ’18 (Sep 11-13): NYC Fashion Week this year caused the 4-day event to get squeezed into 3 busy days. Even so, people mentioned how big – not in terms of people numbers, though it was large – but in terms of how pervasive analytics has become throughout mainstream business. Attending one of the Executive Briefing talks, the row in front of me had people from an insurance company in Iowa. I’d introduced the same speakers/talk in 2017 where only a handful attended. One year later this talk was standing-room only. The people from Iowa remarked how they were new to the concepts presented and eager to learn. As data science practices spread through mainstream business, we’re getting better optics at scale. Some issues of corporate and public accountability can move from key individuals’ opinions and biases, toward objective analysis and visualization from data at scale. That’s good for data science and for business, although instances of this may show up in some less than intuitive ways…
Quantifying forgiveness
One of the most poignant Strata keynotes was “Quantifying forgiveness” by Julia Angwin. Julia is the author of Dragnet Nation, who led the famous ProPublica investigation of racial bias in ML models used for criminal risk scores in parole hearings. She explored what she’s learned about forgiveness from investigations of algorithmic accountability. In other words, who gets a hall pass for legal infractions vs. who goes directly to jail, due to built-in biases in automation based on ML models? These are “lessons we all need to learn for the coming AI future” where algorithms increasingly become the arbiters of forgiveness. Overall, this work illustrates many examples of outstanding data science practice, and especially compelling visualizations.
https://twitter.com/ginablaber/status/1040238561726025728
Julia’s next venture in data journalism and accountability was just announced: The Markup, a nonpartisan, nonprofit newsroom in New York “illuminating the societal harms of emerging technologies” which is funded by Craig Newmark, Knight Foundation, Ford Foundation, MacArthur Foundation, etc.
Sound and its effects on cognition
In a very different example of accountability, can data analytics point to harmful business practices, at scale, which are (1) invisible, and (2) cause long-term physical damage to consumers?
“Sound design and the future of experience” by Amber Case, opened with a subtle, profound soundscape. No images, solely an audio recording from her Manhattan hotel room the evening before, exploring audible textures coming in from the street. We process sounds constantly, even while sleeping, with significant effects on our cognition. For example, sounds we hear aboard a commercial flight affect our ability to taste food: Finnair composed a custom soundtrack to make their inflight food service taste better.
Amber is co-author of the upcoming Designing Products with Sound, and I’ve been pouring through an early release. Highly recommended for serious data science work. In her keynote, showing designs of various products such HVAC, hair dryers – or my pet-peeve on corporate campuses, the inescapable leaf blowers – Amber made the point how “these are all compromising our ability to relax, think better, and be more interested in our environment.”
Backstage at Strata, Amber meandered through A/V gear with Spectrogram Pro, pointing out which workstations generated the most noise, which keynote speakers needed filtering adjustments to make them more understandable. Ironically, the evening before I’d been at John Dory Oyster Bar for an industry dinner. Had to cup my ears, struggling to hear Saar Yoskovitz, Augury CEO, describe how they use ML on acoustic sensors to diagnose industrial equipment failures. We measured 100+ decibels in the “quiet” corner of the John Dory basement dining room. While trendy for high-end eateries, that noise level is enough to cause damage – and urban millennials are projected to experience “aging” effects of hearing loss two to three decades earlier than their parents. Fortunately, there’s an app for that – which hopefully leads to better accountability for health standards being enforced in spaces open to the public.
SoundPrint restaurant noise levels in SF
A central theme in Amber’s work is about calm technology, the opposite of products that steal attention. This should inform how we build analytics and reporting. For example, how readily do decision-makers learn from the data visualizations and other interactive materials which we produce as data scientists? Are there ways of calming our analytics results, allowing people to think better about data insights and business strategy? Another example of accountability – about the effects of how we present analytics.
BTW, if you haven’t already caught the one-day course by Ed Tufte, “Presenting Data and Information”, place that into a high-priority bucket list for $380 invested wisely. Each participant receives a set of his fantastic hardcover books, which together sell on Amazon for about 50% of the course registration fee. Plus it’s an astoundingly solid day filled with statistics and data visualization. I mention this because Tufte’s practices such as data ink, sparklines, etc., fit well alongside Amber Case’s work.
The Data of Bias in Hiring
One of the excellent Data Case Studies was Maryam Jahanshahi at TapRecruit: “‘Moneyballing’ recruiting: A data-driven approach to battling bottlenecks and biases in hiring”. They’ve analyzed lots of data about data science hires. One observed issue is title inflation among junior data scientists. Consequently, using the phrase “senior data scientist” in a candidate search creates a bottleneck instead of a good filter – reducing success rates for hiring by 50%. Also, the two most important perks and benefits in job descriptions turn out to be mentions of health insurance and 401K – while the phrase “unlimited vacation” translates across age demographics as stingy company, overly competitive environment, can’t imagine fitting in there. Another important point: tech firms often taut “hacking diversity” as quick fixes, but that’s a myth. Maryam showed that when you have larger candidate pools you end up with more diversity among new hires. In contrast, people tend to makes biased microdecisions in hiring, especially at the margins, which result in smaller candidate pools.
Given ~150 talks, there’s much more to summarize from Strata, although the full video set is still getting placed online. Here’s a good summary by Maggie Seeds:
“That future has arrived, and the buzz is now around how to best store, process, and analyze data to efficiently deliver insights to drive decisions.”
Ethics and Privacy in AI
Meanwhile, The AI Conf, SF (Sep 4-7) sold-out. I recorded video interviews with attendees and vendors on the expo floor and remarks were consistent:
“In previous years, people would ask ‘What is AI?’; however, this year they come up and describe their use case then ask ‘How can you help me on that?’ instead.”
That fits well with the Strata NY takeaway of data science moving across mainstream business.
Two talks at AI SF ’18 explored AI in terms of ethics, privacy, and what tangible steps can your team take today? Although slides and videos aren’t online yet, Susan Etlinger from Altimeter Group will present the talk “Ethical AI - How To Build Products that Customers Will Love and Trust” again at the AI London conference on October 11. Susan was on a podcast by In Context in June, covering related topics:
- defining ethics and trust in a consumer enterprise setting
- why enterprise firms “should view ethics as a competitive differentiator, rather than a boring compliance exercise”
Check out her Altimeter Group white paper, “The Customer Experience of AI: Five Principles to Foster Engagement, Innovation and Trust”. One of Susan’s key takeways, reinforcing Maryam Jahanshahi’s points above, is that individuals make microdecisions based on their own biases (which everyone has) that cascade into microaggressions in aggregate. Again, this is related to how people do not naturally make good decisions in large groups. Even so, data science can help augment that baseline human condition, so that large organizations can collaborate to reach decisions objectively and ethically – as a competitive differentiator.
Mike Loukides, Hilary Mason, and DJ Patil wrote a series of data ethics articles this summer, working with Susan Etlinger and others for core material. A data ethics checklist resulted, and now Data For Democracy is working to coach data science teams to put that checklist into production. I spoke with D4D community manager Mo Johnson recently, and she’ll be traveling far and wide to present. D4D is a volunteer organization, and check out how to get involved.
Amanda Casari presented about ethics and privacy in the enterprise, “When Privacy Scales: Intelligent Product Design under GDPR”. New stuff: Deon provides an ethics checklist for data scientists which is GitHub-friendly. Along these lines, see the Aequitas “open source bias audit toolkit” for ML developers, from U Chicago.
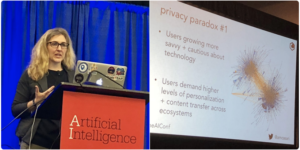
Amanda Casari at AI Conference, Photos by Paco Nathan
Also, I presented “Open source decentralized data markets for training AI in areas of large shared risk”, and learned about a related open source project PySyft “for encrypted, privacy preserving deep learning” based on PyTorch. As dialog deepens about privacy, ethics, bias, compliance, expect to see more tooling which decouples private data from model training. Ultimately risk management plays the “thin edge of the wedge” for these changes in the enterprise.
Reinforcement Learning challenges assumptions
Danny Lange’s talks about Artificial General Intelligence (AGI) got recommended here last month. Let’s take a look at key points, plus a related paper just published. In brief, there are assumptions in data modeling work that we must start with lots of labeled data to arrive at a good ML model. Then if you want to improve the predictive power of that model, obtain more/better data. Sometimes, however, you can leverage domain knowledge so that models don’t need to be trained from scratch or be based solely on mountains of data. R&D work in reinforcement learning is challenging some of those assumptions, pulling in decades of preexisting practices from other fields to augment AI.
Training Puppies
At AI SF, Danny Lange presented how to train puppies: “On the road to artificial general intelligence” – game simulations Unity3D plus reinforcement learning used to train virtual puppies to play “fetch” and other skills. Building on this, Danny described several forms of learning inspired by biology, which go beyond deep learning. He showed examples of virtual puppies for:
- Imitation Learning: e.g., see https://bit.ly/2zvYH51 (start 0:15)
- Curriculum Learning: start with an easy problem, then make learning challenges progressively harder
- Curiosity-driven Exploration: gets beyond problems which random exploration would never reach, i.e., agents don’t get stuck in a room (saddle points) because they want to explore other rooms
https://twitter.com/danny_lange/status/1045017157539713031
Two interesting footnotes:
- it’s simple to run thousands of those training simulations in parallel, then combine traits from successful learners
- difficult machine learning was accomplished within a virtual environment without people providing labeled data
A recent paper describes this in detail: “Unity: A General Platform for Intelligent Agents”, and their team is making their implementations open source. While some point to “Artificial General Intelligence” as a harbinger for the end of the world (or something), Danny urges the value of open source combined with readily computable methods: “The safest way to build AI is to make sure that everybody can use it.” Speaking of readily computable methods, Unity runs in 50% of mobile games.
Pywren and other new projects
Brace yourself for a whirlwind, with the caveat that I had to listen to the podcast interview “Building accessible tools for large-scale computation and machine learning” with Eric Jonas and Ben Lorica eight times before sketching their outline. You won’t find a broader perspective of full-stack, seriously. While the core of the interview is about Pywren, Eric and Ben go into exquisite detail about reinforcement learning and its relationship with optimal control theory, going back into the 1950s. We would not fly on airlines or drive cars on freeways without this. RL provides ways to leverage domain knowledge so that models need not be trained from scratch solely from data. Moreover, those models can have more predictable results. The math involved gets into Bayesian methods, calculus of variations, etc. Brace yourself, but the upshot is optimistic for robust ML models in the industry.
Pywren is a new open source project that “lets you run your existing python code at massive scale” on serverless computing in the cloud. It works well with scikit-learn, pandas, etc., scaling-out on cloud/serverless for:
- rapid ETL for ad-hoc queries (not pipelines)
- quantitative analysis of ML approaches (e.g., the science)
The project began with Eric’s realization, as a post-doc at UC Berkeley’s RISE Lab, that many grad students at AMP Lab and RISE Lab had never written an Apache Spark job. Repeat that last sentence a few times, let it sink in… while running PySpark in standalone mode is simple, the set up for a Spark cluster requires non-trivial amounts of data engineering before science begins to happen.
A sea change in architecture
Apache Hadoop and HDFS emerged back when there were lots of cheap “spinning disks” and “commodity hardware” Linux servers, albeit those disks had high failure rates. Hadoop provided a reliable framework for completing a batch job, even if disks failed while the job was in progress. The novel strategy was to host your datasets on a cluster of commodity hardware, then bring your compute to the data – leveraging data locality for parallelism as much as possible. Circa 2008, I led a team that ran one of the largest Hadoop instances on Amazon AWS. Gluttons for punishment can watch Dave Patterson wield his “thesis defense” Jedi tricks in my 2009 guest lecture at Berkeley.
A few years later, along came Spark. Much the same model, with more flexible use of combinators, plus much more leverage of in-memory data. Later we got multicore, large memory spaces, and cloud storage turned out to work amazingly well – plus Moore’s Law died.
These days with fast commodity networking, the economics of cloud services don’t resemble “commodity servers” circa early Hadoop at all. The argument centers more on networks than servers, but anywho Eric Jonas and crew did tons of research about bottlenecks, throughput, failure rates, economics, etc. Instead of bringing your compute to the data, now bring your data to the compute.
Eric describes cluster utilization in Spark and Hadoop in terms of “damped sinusoidal” curves where you define the max cluster resources needed, but only use portions in the later stages of a pipeline. However, “pipelines” are about batch – and the world is moving away from batch. In contrast, running Python functions at scale on Pywren tends to be 2-3x less expensive than comparable work on Spark. While serverless is not a panacea (i.e., because trade-offs) we’ll see more analytics running on the likes of Pywren. An up and coming cohort of open source frameworks leverage newer hardware and cloud architectures: Arrow, Crail, Nuclio, Bullet, etc. The latter is a real-time query engine from Yahoo for large data streams, with zero persistence layer.
Learning over coding
Tying this back to IT practices and software engineering in general, note how Eric Jonas referenced issues related to unbalanced joins, where ML models have much to offer. That’s a cue for yet another data point showing how learning is proving to be more general-purpose than coding. Following on that, Zongheng Yang from RISE Lab recently published “SQL Query Optimization Meets Deep Reinforcement Learning”:
“We show that deep reinforcement learning is successful at optimizing SQL joins, a problem studied for decades in the database community. Further, on large joins, we show that this technique executes up to 10x faster than classical dynamic programs and 10,000x faster than exhaustive enumeration.”
In other words, using RL to predict how to optimize SQL joins. That’s potentially a big win for enterprise IT, where efficiency of SQL joins is so much of the game.
Strategy for Hadoop/Spark was about leveraging batch and mini-batch. For ML applications you’d focus on coding a pipeline to clean your data, run joins at scale, extract features, build a model on a cluster, then deploy the model into production. Eric Jonas and others from RISE Lab now point toward a sea change in architecture and practices where RL is more concerned about bringing data updates (streaming/real-time) to the compute (i.e., serverless at scale, mobile devices out in the world) for optimal control approaches. The math can be daunting, however results are potentially more predictable and robust.
The world is long-tailed
Assumptions challenged, then how much data is needed for effective modeling? Here are a few recent gems on that subject…
Did you know: you can use regression instead of t-tests? For those who run customer experiments online with A/B testing, that may change your approach to collecting data, plus how much data you need before taking action – which affects the velocity of tests. Stitch Fix has an excellent presentation that goes back to the math (e.g., between-condition variability) to dispel prevalent mythology about the statistics of A/B tests.
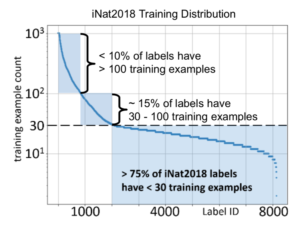
Source: Deep Learning Analytics in iNat 2018 Challenge
In terms of deep learning, it’s accepted that DL models require 106 as a minimum size for training datasets. The excellent paper “The Devil is in the Tails: Fine-grained Classification in the Wild” by Grant Van Horn, Pietro Perona / CalTech (2017-09-05) explores quantitative relationships between size of training data, number of classes, and model error rates:
“The number of training images is critical: classification error more than doubles every time we cut the number of training images by a factor of 10. This is particularly important in a long-tailed regime since the tails contain most of the categories and therefore dominate average classification performance.”
Van Horn and Perona open with a brilliant one-liner: the world is long-tailed. The diagram above shows analysis from Deep Learning Analytics, the #2 team placing in the iNaturalist 2018 competition. Part of that challenge was how many of the classes to be learned had few data points for training. That condition is much more “real world” than the famed ImageNet – with an average of ~500 instances per class – which helped make “deep learning” a popular phrase. The aforementioned sea change from Lange, Jonas, et al., addresses the problem of reducing data demands. I can make an educated guess that your enterprise ML use cases resemble iNaturalist more than ImageNet, and we need to find ways to produce effective models that don’t require enormous labeled data sets.
DARPA, one of the prime movers in the field, recently published “Reducing the Data Demands of Smart Machines”, along with announcing a $3B program:
“Under LwLL, we are seeking to reduce the amount of data required to build a model from scratch by a million-fold, and reduce the amount of data needed to adapt a model from millions to hundreds of labeled examples,” said Wade Shen, a DARPA program manager in the Information Innovation Office (I2O) who is leading the LwLL program. “This is to say, what takes one million images to train a system today, would require just one image in the future, or requiring roughly 100 labeled examples to adapt a system instead of the millions needed today.”
Techniques such active learning, transfer learning, meta-learning, k-shot learning, etc., are suggested for research to make machine learning work effectively with smaller data samples – i.e., long-tailed data. FWIW, I presented “Best Practices for Human-in-the-loop: the business case for active learning” at AI SF, which provides examples of addressing data demands in the enterprise.
There’s also work on synthesizing data in some cases. Augmentor, as an example, is a Python package for “augmentation and artificial generation of image data for machine learning tasks”.
AI and Economic Theory
As mentioned earlier, there’s a lot of public discussion about AI displacing jobs, declining R&D efforts, etc. Some of those assumptions appear to be wrong. For example, see another recent Lange paper, “The Impact of Artificial Intelligence on Economic Growth: Examining the Role of Artificial Intelligence in Idea Generation" by Yina Moe-Lange.
Yina, who’s also an Olympic skier, presents a fascinating thesis complete with lit surveys about the propagation of newly invented ”general-purpose technologies", the changing relationship of R&D vs. labor, the dramatic evolution of hardware capabilities, plus contemporary theory about capital stock creation (in the economic sense, not company valuation). This builds atop Agrawal, et al., on prospects for technology-driven economic growth. After Yina identifies a few math bugs in earlier theoretical work, her summary: AI amplifies R&D, boosting capital stocks, with more profound effect on jobs and global economics than labor displacement.
Economic Outlook
Yina Moe-Lange isn’t alone. At AI SF, Mehdi Miremadi from McKinsey Global Institute corroborated in “Have we reached peak human? The impact of AI on the workforce”:
- economic futures look bleak when based on current trends in labor supply
- “productivity growth” over the past five decades was based on large numbers of people entering the workforce
- our ability to automate (up to 30% of many jobs) is how the story of AI will unfold
To wit, advanced analytics have near-term potential to unlock $11–13T in the economy (~15% of global GDP), with deep learning accounting for ~40%. Historical analogies exist, e.g., the era of early PC adoption created jobs despite dire warnings to the contrary.
However, there’s one catch: robust data infrastructures and effective practices for data engineering and data science are table stakes in this game. Tomorrow’s capital stock creation based on AI begins with good data science practices today.
Enterprise AI adoption by segment, partly based on MIT SMR 2017
Note that the top segment in the table above has about a dozen firms, while the second and third segments split roughly 50-50. Human-in-the-loop is a good approach for the second segment to gain an advantage over the first, while even the third segment will typically have legacy assets – in terms of unused data exhaust – which may be leveraged for advantage.
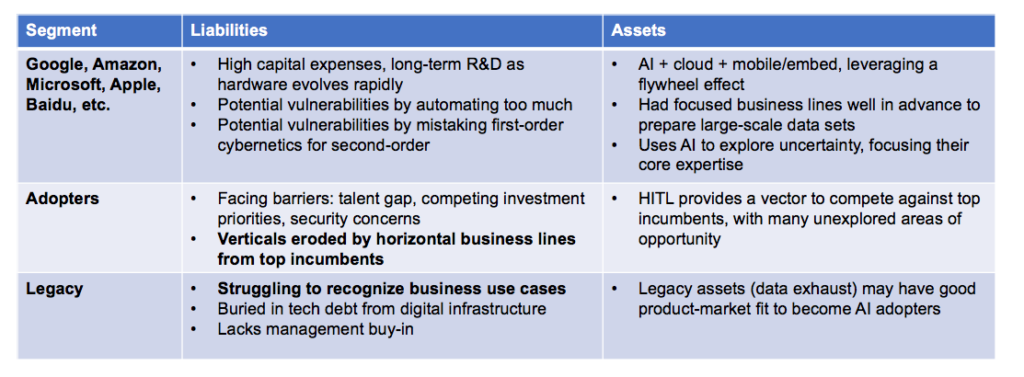
Enterprise AI adoption by segment, partly based on MIT SMR 2017
Here’s a counter-example: “AI” done badly. As a “hypothetical”, consider segmentation of AI adoption among three competitors in consumer shipping: Amazon in the top segment as an incumbent, FedEx in the second segment with opportunity plus an evolving data culture, while UPS struggles with data infrastructure.
Pulling it all together
Okay, taking a deep breath, that was much to cover. To recap, data science is getting leveraged for better corporate and public accountability – to augment/overcome the inherent human condition of microdecisions, microaggressions, and lack of making good judgments in large groups. Meanwhile, the economics of newer hardware, newer cloud architectures, faster networks, etc., imply movement away from the era of data practices based on batch, moving toward a sea change that involves more streaming, reinforcement learning, serverless, etc. These practices challenge assumptions in ML and are beginning to demonstrate ROI for AI in the enterprise. That’s good because we’re feeling pressure from data demands associated with “smart machines” as well as the reality of long-tailed data in real-world use cases. Taken up another level of abstraction, the economics of AI are also challenging assumptions about econometrics, employment rates, effective R&D, capital stock creation, etc. Again there we see how best practices associated with data science are table stakes for the future.
Summary
- Introduction
- Data Science for accountability
- Quantifying forgiveness
- Sound and its effects on cognition
- The Data of Bias in Hiring
- Ethics and Privacy in AI
- Reinforcement Learning challenges assumptions
- Training Puppies
- Pywren and other new projects
- A sea change in architecture
- Learning over coding
- The world is long-tailed
- AI and Economic Theory
- Economic Outlook
- Enterprise AI adoption by segment, partly based on MIT SMR 2017
- Pulling it all together
Subscribe to the Domino Newsletter
Receive data science tips and tutorials from leading Data Science leaders, right to your inbox.
By submitting this form you agree to receive communications from Domino related to products and services in accordance with Domino's privacy policy and may opt-out at anytime.