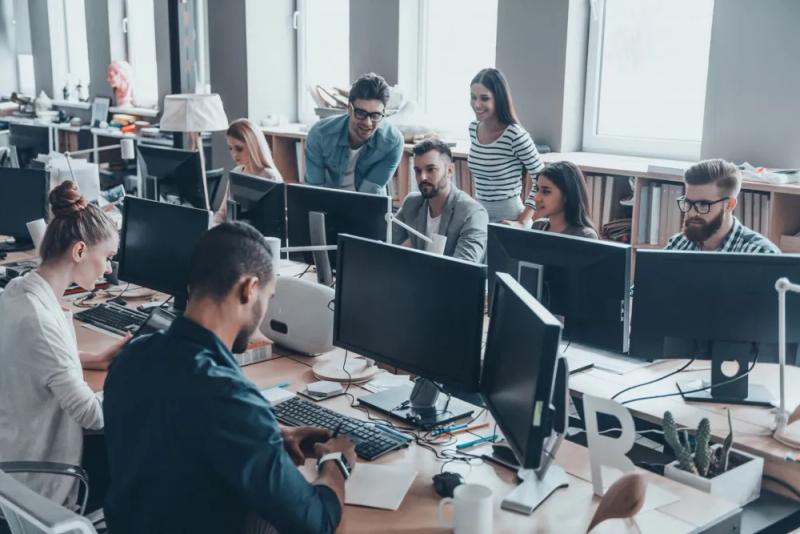
This week Gartner published their latest Magic Quadrant for Data Science and Machine Learning (DSML) Platforms. Meanwhile, Forrester has two separate Waves for Predictive Analytics and Machine Learning (PAML) platforms — one notebook-based and one multi-modal — plus a third New Wave for automation-focused machine learning solutions. And Matt Turck’s 2020 Data & AI Landscape lists hundreds of companies in dozens of sub-categories.
What’s most clear and consistent from all this analysis is that it’s not clear and consistent — about how to define the space and which vendors address which needs. Against that backdrop, we wanted to articulate the need that we at Domino see in the market and what problems we are focused on solving for our customers.
Put simply: Our focus is on helping enterprises with large teams of “code-first” data scientists.
We believe enterprises with large code-first data science teams are having the biggest impact on the world’s most important challenges. Enterprises have large, often global impact. If a company has grown a sizeable data science team, it demonstrates they see data science as strategic and critical to their business. Why else would they be making such a large investment in data science? And while “citizen data scientists” are certainly valuable for some problems, in our experience it’s the code-first data scientists (i.e., folks working primarily in statistical programming languages) who are providing the greatest value, and delivering the most innovative, mission-critical data science work.
The past year has provided strong validation for us that enterprises with code-first data scientists create outsized value. We are fortunate to have a number of pharma and healthcare companies among our customers. It’s been a privilege to help accelerate the work they have been doing during this critical time.
Data science teams in these organizations have unique challenges given their scale and complexity. Supporting them is not merely a matter of providing the algorithms or infrastructure to let one data scientist train and deploy a model. Nor is it merely about supporting larger volumes of data or speeding up ETL tasks. These enterprises have problems of scaling data science as a discipline — across people, processes, and infrastructure. These are the problems we are solving with Domino. Here are three of them:
- Balancing infrastructure agility with IT governance. All data scientists benefit from access to powerful compute resources and being able to experiment with new, open-source tools and packages. In an enterprise, providing this agility is challenging because data scientists are spread across different departments and use a variety of analytics stacks: e.g., R, Python, even SAS or MATLAB. This increases support burden, support cost, and operational and security risk for IT organizations. Unfortunately, enterprises feel stuck between two bad choices: restrict data scientists’ agility, or run the risk of “ungoverned” data science. Domino has solved this problem with a unique “open” architecture that lets enterprises consolidate multiple tools (e.g., RStudio, SAS, Jupyter, MATLAB) on central infrastructure that can sit on elastic, scalable compute and be managed and governed by IT. This creates self-serve, governed sandboxes for data scientists.
- Eliminating siloed work. In any sizable research organization, collaboration and knowledge management are critical — to unlock new ideas and breakthrough insights, and to save people time by letting them build on past work rather than reinventing the wheel. Tracking work and knowledge produced during the data science process is tricky, and traditional knowledge management tools (wikis or source control) don’t work. Domino has solved this problem with unique capabilities for automatically tracking work to make it fully reproducible, searchable, and discoverable.
- Delivering enterprise infrastructure and security. Data science work requires powerful compute resources, access to high-value data, and the development of core intellectual property. As a result, data science platforms are subject to the most complex IT and security requirements within an enterprise. We have invested heavily to support the most stringent and sophisticated IT/security requirements. As a result, Domino is embraced by some of the most security-conscious, complex IT organizations in the world.
We believe these are some of the biggest barriers holding back data science from addressing the world’s most important challenges. Many of these problems are not sexy — but they are hard, and they are valuable.
We also know that not everyone agrees with us that these are important and valuable problems to solve. Many other vendors in this space are focused on different problems. And some analysts are critical of Domino because we focus on large teams of code-first data scientists in enterprises.
From our perspective, we know we are creating a ton of value for enterprises. Lockheed Martin is a great example. When they wanted to centralize access to data science tooling, streamline collaboration and knowledge sharing, and automate DevOps tasks to increase data scientist productivity, they turned to Domino. Today, they attribute $20 million in annual cost savings to their use of our platform thanks to reduced IT costs, increased onboarding efficiencies, and a 10x increase in data scientist productivity.
We are ruthlessly focused on helping enterprises accelerate code-first data science at scale. And that focus is paying off for our business: over 20% of the Fortune 100 use Domino to develop, deploy, monitor, and manage hundreds, or in some cases, thousands of models — to beat competitors, upend industries, and drive unprecedented growth. And we’re looking forward to accelerating data science for many more companies in the years to come.
Nick Elprin is the CEO and co-founder of Domino Data Lab, provider of the open data science platform that powers model-driven enterprises such as Allstate, Bristol Myers Squibb, Dell and Lockheed Martin. Before starting Domino, Nick built tools for quantitative researchers at Bridgewater, one of the world's largest hedge funds. He has over a decade of experience working with data scientists at advanced enterprises. He holds a BA and MS in computer science from Harvard.
RELATED TAGS
Subscribe to the Domino Newsletter
Receive data science tips and tutorials from leading Data Science leaders, right to your inbox.
By submitting this form you agree to receive communications from Domino related to products and services in accordance with Domino's privacy policy and may opt-out at anytime.