Why Hybrid Cloud is the Next Frontier for Scaling Enterprise Data Science
Kjell Carlsson2022-06-22 | 9 min read
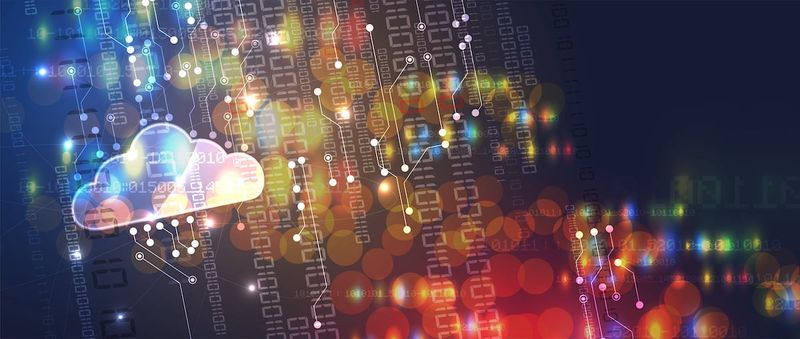
An exciting new trend is rising in enterprise data science, and it’s breaking down the silos between on-premises and cloud environments to unlock the benefits of each, all while improving collaboration and regulatory compliance. Advanced, model-driven companies—especially the ones that are out-innovating their competitors with machine learning and AI—are adopting hybrid cloud strategies for their data science initiatives. The most advanced are even repatriating data science workloads back on-premises, while simultaneously exploiting the flexibility of multiple cloud environments.
In so doing, data science as a whole is taking an evolutionary step forward. It's also following the path already taken by compute, storage, and data platforms and making the jump from an on-premises strategy, to cloud, and now hybrid. This trend is so pronounced that in a recent Forrester survey of AI infrastructure decision makers, 91% said they will be investing in hybrid cloud within two years and 66% said they already had invested in hybrid support for AI workloads.
It’s a strategy that seems to be working. In the same survey, those businesses that had invested in hybrid cloud reported fewer challenges than their cloud-focused brethren, at every step of the data science lifecycle—from data preparation through deployment and monitoring.
A Hybrid Strategy vs. 'Stuck with Hybrid'
It’s important to differentiate hybrid cloud strategies from the status quo afflicting many organizations that are stuck with a set of siloed environments scattered across on-prem locations and sometimes, across several cloud service providers. Both are technically hybrid cloud because they're running workloads on-premises and in at least one other cloud environment. However, the similarities end there.
Most large organizations today are “stuck with hybrid." They have ended up with multiple environments with little if any integration between them, due to piecemeal modernization efforts, regulations, acquisitions, shadow IT and a lack of coordinated strategy. Data is siloed, tools are restricted, and utilization is simultaneously low in certain areas, while capacity is insufficient elsewhere. This situation stifles collaboration, innovation and efficiency.
In contrast, the new generation of hybrid cloud organizations are breaking down the silos between environments to run data science workloads where they make the most sense based on cost, performance, and regulatory considerations. They are implementing strategies to leverage the strengths and avoid the weaknesses of different environments, to provide the holistic picture needed for governance and operational efficiency, and facilitate access and collaboration across teams.
Hybrid Cloud Successfully Tackles Four Key Data Science Challenges
In conversations with data science leaders, they point to four key factors that are driving them to embrace strategies that are explicitly focused on hybrid cloud. In order of importance they are:
- Data localization and sovereignty: Gartner is predicting that 65% of the world’s population will have its personal data covered by new privacy regulations, while Forrester is predicting a new era of “cloud nationalism.” Indeed, many countries already have data privacy laws that require customer data to be processed locally. Hybrid cloud enables data locality, i.e. you can push model training and inferencing to where the data reside, whether that be in a regional cloud instance or a local on-prem data center. In addition to regulatory compliance, this helps meet the performance requirements, such as for latency, for particular workloads, and enables you to leverage data science in regions where your cloud vendor of choice does not operate. Google, for example, does not operate in China.
- Cost: As a company looks to apply data science for more use cases in more parts of the organization, the cost of infrastructure compounds. Ultimately it becomes a major barrier to both growth and innovation, particularly when it comes to deep learning models e.g., models for computer vision or NLP tasks. Hybrid cloud gives you the power to repatriate these workloads down from the cloud to take advantage of the cost benefits of on-prem hardware, significantly reducing TCO. Cloud vendors charge a very significant premium for AI-optimized hardware, and even as the price of compute goes down, there are few signs of these markups going away.
- Flexibility: Data science workload demand fluctuates wildly even by the standards of already volatile application workloads. Model training jobs can vary dramatically depending on the volume of data, the type of model, and the extent of your hyper parameter optimization - as well as such capricious factors as the timing of projects, the work schedule of your data scientists, and changes in your data. Hybrid cloud enables you to “burst” from on-prem to take advantage of the ability to rapidly scale up (more powerful instances) and scale out (larger clusters) in the cloud as needed.
- Lock-in: As Michael Warrilow, VP analyst at Gartner, has said: “Most organizations adopt a multi-cloud strategy out of a desire to avoid vendor lock-in or to take advantage of best-of-breed solutions.” The same is even more true for hybrid cloud. As companies start to rely increasingly on the cloud they are also starting to worry about the hold these vendors have over their businesses. At its most extreme, the cloud vendor can even become a competitor. Retailers avoid AWS for this reason, but financial services, insurance and healthcare will need to find alternatives whenever Amazon decides to enter their verticals. Hybrid cloud provides companies an escape route to on-prem or another cloud and helps a company hedge its bets between cloud vendors.
The First Enterprise Hybrid Cloud Data Science Platform
A hybrid cloud approach has many benefits and recognizes the reality of the on-prem systems and regulations companies face. However, to take full advantage of hybrid, companies must move on from the manual processes and disconnected platforms they have today.
Data science teams can collaborate better and be more productive with a true hybrid platform that enables them to access data, compute resources and code in every environment where the company operates, in a secure, governed fashion. The alternative is spiraling cost, wasted effort, suboptimal models, and higher risk.
Unfortunately, there have been no hybrid cloud platforms that could support all data science teams across an enterprise. Neither the cloud vendors, nor cloud-focused data science platforms, have made any meaningful investments to create them, because doing so inevitably runs against their own interests. Others offer only point solutions that support a fraction of all data scientists.
However, that is changing, starting today. Together with its partner NVIDIA, Domino is announcing Nexus, the first hybrid cloud platform for enterprise-wide data science. It provides a single pane of glass for data science across all regions and environments of an enterprise, whether they be on-prem, in the cloud, or in multi-cloud settings. Core features are:
- One-click access. Nexus lets you launch new jobs and workspaces on-premi or on different cloud platforms and allows you to select hardware tiers based on your cost and performance requirements.
- Unified data access control. With Nexus, you can designate which environments can access specific data sets. It thus allows you to restrict access to data by region, helping you enforce compliance with data localization and sovereignty regulations.
A beta version of Nexus will be available in the next few months and general availability is set for early next year. If you would like to implement the next generation hybrid cloud platform for data science, get in touch to partner with us as we build it.
Kjell Carlsson is the head of AI strategy at Domino Data Lab where he advises organizations on scaling impact with AI technologies. Previously, he covered AI, ML, and data science as a Principal Analyst at Forrester Research. He has written dozens of reports on AI topics ranging from computer vision, MLOps, AutoML, and conversation intelligence to augmented intelligence, next-generation AI technologies, and data science best practices. He has spoken in countless keynotes, panels, and webinars, and is frequently quoted in the media. Dr. Carlsson is also the host of the Data Science Leaders podcast and received his Ph.D. from Harvard University.
RELATED TAGS
Subscribe to the Domino Newsletter
Receive data science tips and tutorials from leading Data Science leaders, right to your inbox.
By submitting this form you agree to receive communications from Domino related to products and services in accordance with Domino's privacy policy and may opt-out at anytime.