4 Ways to Better Manage and Govern Financial Services and Insurance Models
Nathan Greenhut2022-07-15 | 9 min read
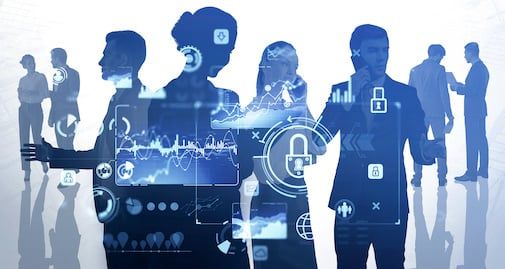
The financial services industries are starting to realize the full import of the fact that, like household chores like dishwashing and garden work, ML models are never really done. Rather, AI and ML models need to be monitored for validity, and often, they also need to be re-explained and re-documented for regulators. So the spotlight is on model risk management (MRM) and governance (MRG), two related critical processes for financial services and insurance companies, and the importance of these two disciplines is only expected to grow.
"You can’t rely solely on well-respected models anymore. You need to understand why a model is applicable for your use, its relevance against other models, how it was built, and its pros and cons," says Jacob Grotta, managing director at Moody’s Analytics.
"Transparency in the modeling process is critically important," he explains.
Both MRG and MRM are therefore big issues for FSI companies. MRG involves a set of rules and processes that describe how models will be created, used, and retired, while MRM is generally the practice of implementing MRG rules and processes. MRG is generally considered the broader term, so in what follows, I have used it to include MRM as one of its sub-disciplines.
Getting MRG and MRM right is difficult. A number of pitfalls and issues can arise that can sap efficiency and productivity. These include the lack of automation, efficient processes, collaboration and communication.
MLOps Helps with MRG and MRM
A full-featured enterprise MLOps platform can solve many of these issues, making the end-to-end process easier and more efficient for everyone involved. Think of a proper enterprise MLOps platform as a concierge that matches information to your role as a data scientist, analyst, data quality manager, risk or compliance manager, IT manager, an executive or even a regulator. Like a good hotel concierge, it gives you the latest, most pertinent information about the steps in the MRG, Audit, Compliance and IT processes, the model, the data and risk that are identified and mitigated, to take away the guesswork in getting your work accomplished. In this perspective, we will discuss MRG, and how an Enterprise MLOps platform can help make it easier for everyone involved.
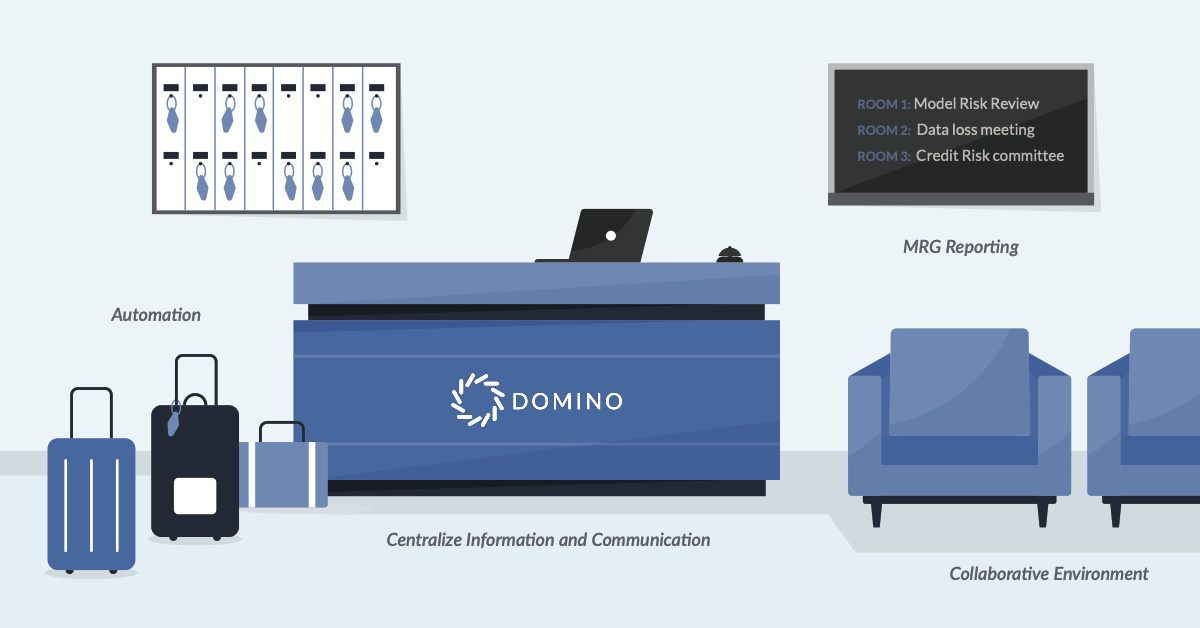
MRG identifies the risks associated with a particular model through a risk assessment. Once these risks have been identified, they can be managed and monitored using a variety of tools and techniques. The following solutions of a robust MLOps platform increase the efficacy, efficiency and effectiveness of your MRG group and enhance business capabilities.
Rule #1: Automate Everything Everywhere to Increase MRG Efficiency and Business Velocity
A recent McKinsey survey on model risk management found that 54 percent of banks believed that automation will be the top solution for enhancing MRG efficiency. That's where an Enterprise MLOps platform comes in. Automation of MRG processes, such as checking for data quality, above the line and below the line testing, model or data drift reports, not only streamlines workflows across teams, but can improve both documentation standards and consistency in the model development and deployment processes. Teams get more verifiable and accurate model results through integrated peer review and analysis, as well as the ability to compare current results against previous results. The platform can also provide valuable insights and information about the risks that are associated with a model, which helps businesses make better decisions about how to manage them.
For example, a leading Fortune 500 insurer enhanced its MRG processes with the Domino Enterprise MLOps Platform, taking advantage of automatic tracking and full reproducibility of experiments, datasets, tools, and environments. Now the company has an audit trail of exactly what work has been done and who accessed what data. This eliminated issues with passing model code and data to the risk manager prior to deployment. Before Domino Enterprise MLOps, this was done with little documentation, which added time and complexity to the governance and compliance processes.
Rule #2: Collaborate & Create a Shared Environment for All Stakeholders
An Enterprise MLOps platform can also improve collaboration between different departments within a company, particularly when it comes to model risk management and governance. Many different departments are involved in MRG and MRS, such as data scientists, analysts, risk managers and the audit department. They’ll have access to the same consistent, correct version of the relevant information, and they can also build upon the work of peers.
For example, Allstate uses Domino’s Enterprise MLOps platform across its data science teams. A key benefit to them is the audit cabability of going back to an analysis at any point in time, which makes it possible to show senior management or a regulator how a number was obtained. This really improves auditability. Another plus is that enterprise MLOps makes data science a repeatable process.
DBRS, a top-four credit-rating agency, also takes advantage of Domino to foster collaboration across teams, without any team member having to replicate environments or work with tools that are not familiar.
“It’s a time saver,” said Michael Sinclair, a quantitative analyst for the firm. “You don’t have the usual difficulties of following a rigorous documentation program; you can just do your work and [Domino will] track for you. You can go back and change something if you need to, you can move things around, or you can tag somebody if you need help. It’s a centralized hub.
Rule #3: Improve Intelligent & Instrumented Reporting to Help the Model Review Process
Risk reporting also benefits from an Enterprise MLOps platform. Experimentation and due diligence on information from the models can be properly monitored and even automated for future model reviews. Additionally, these components can be stored, tagged and shared for future reuse in the analytics and AI libraries, making it easier for businesses to produce accurate and up-to-date reports on model risk.
Denmark’s second-largest insurer, Topdanmark, struggled to monitor models in production with high confidence about the quality of automated decisions. For example, it took two data scientists three months to dig through data sources to determine that one data set feeding an algorithm wasn’t formatted correctly and was causing the model to drift. Now they use Domino to automatically monitor models, sending alerts for model drift and identifying where to look to understand the issue.
“I believe real-time model monitoring capabilities within an Enterprise MLOps platform are crucial if you’re going to show you’re delivering the value you expected and resolve any issues quickly.” says Stig Pedersen, lead of data science CoE at Topdanmark.
Rule #4: Use One Platform to Centralize Information and Communication to Mitigate Risks
An enterprise MLOps platform can help financial services and insurance companies to improve the way they communicate model risk information to their entire universe of internal and external stakeholders. Everyone can easily access model risk information from the central repository. This helps to ensure that everyone is aware of the risks associated with a model, and that they can be managed effectively.
DBRS also uses Domino to provide visibility into their credit scoring methodologies. It is critical that DBRS’s rating decisions, model and methodology development have strict governance and auditability. Finding ways to automatically demonstrate governance relieves researchers from having to compile documentation drives faster time to market of models. Today they can easily show and communicate all the data and code that went into analysis as Domino preserves the full experimental record automatically—who was involved, what methodologies were attempted and discarded, when was it promoted to production and so on.
"Domino helps mitigate risk by not having to solely rely on humans to communicate, and simply capturing all that communication in the code base,” says Thomas Little, global head of Product Management for DBRS.
Nathan Greenhut has worked in the banking and financial services industry for over 20 years in data, analytics, data science, risk management, governance, among other areas, and has helped to develop model risk management and governance processes for many of the world's leading banks and financial institutions.
Summary
Subscribe to the Domino Newsletter
Receive data science tips and tutorials from leading Data Science leaders, right to your inbox.
By submitting this form you agree to receive communications from Domino related to products and services in accordance with Domino's privacy policy and may opt-out at anytime.