Domino 5.0: Accelerate the Root Cause Analysis of Model Drift
Vinay Sridhar2022-02-03 | 5 min read
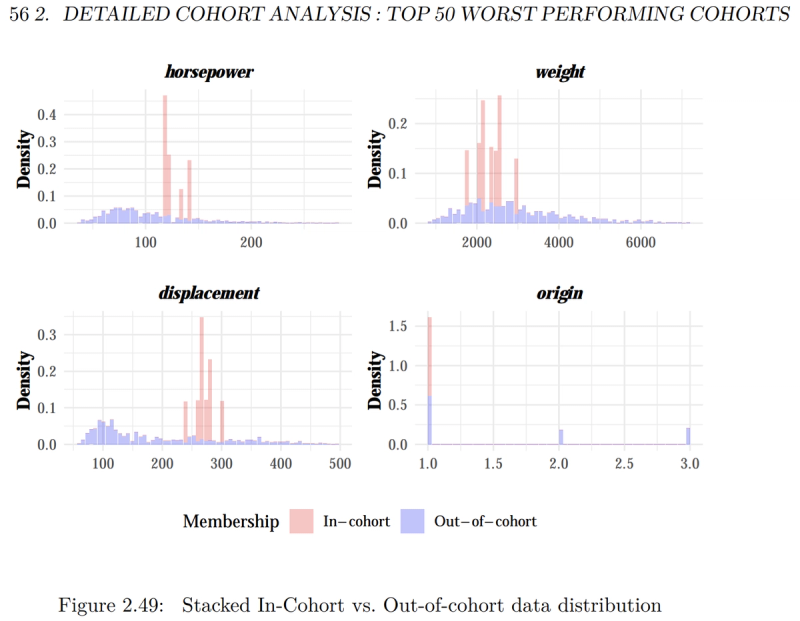
Introducing Automated Model Quality Insights in Domino 5.0
Models are frequently deployed into applications that process large volumes of data. They often include hundreds or thousands of features with a high degree of cardinality. When such a model with this level of complexity is not performing as expected, it can be difficult to diagnose the root cause. Domino addresses this problem by helping data scientists find input data cohorts impacting model quality. This allows data scientists to quickly retrain the model to account for these cohorts, improving the overall performance of the model.
Cohort Analysis Report
Domino automatically generates a report that highlights the worst performing cohorts (or segments) on the basis of model quality. For each cohort, the report details the features within that cohort that form a hotspot impacting the model’s accuracy. With contrast scores assigned to each feature in a cohort, users can discern the difference in model quality compared to the rest of the data outside this cohort. This helps a user prioritize cohorts and features within a cohort to investigate further and take remedial action.
Cohort Analysis Stats and Customization
In addition to the report, Domino automatically sets up a project which includes:
- Python code used to perform the cohort analysis
- Cohort analysis results in the form of JSON files that represent aggregate summary stats across cohorts and per-cohort performance details with contrast scores
Data scientists can easily customize the cohort analysis code to generate custom reports, or use the outputs of these reports to perform deep, custom analyses of the model as they move on to the remediation step.
Domino is the Enterprise MLOps platform that seamlessly integrates code-driven model development, deployment, and monitoring to support rapid iteration and optimal model performance so companies can be certain to achieve maximum value from their data science models.
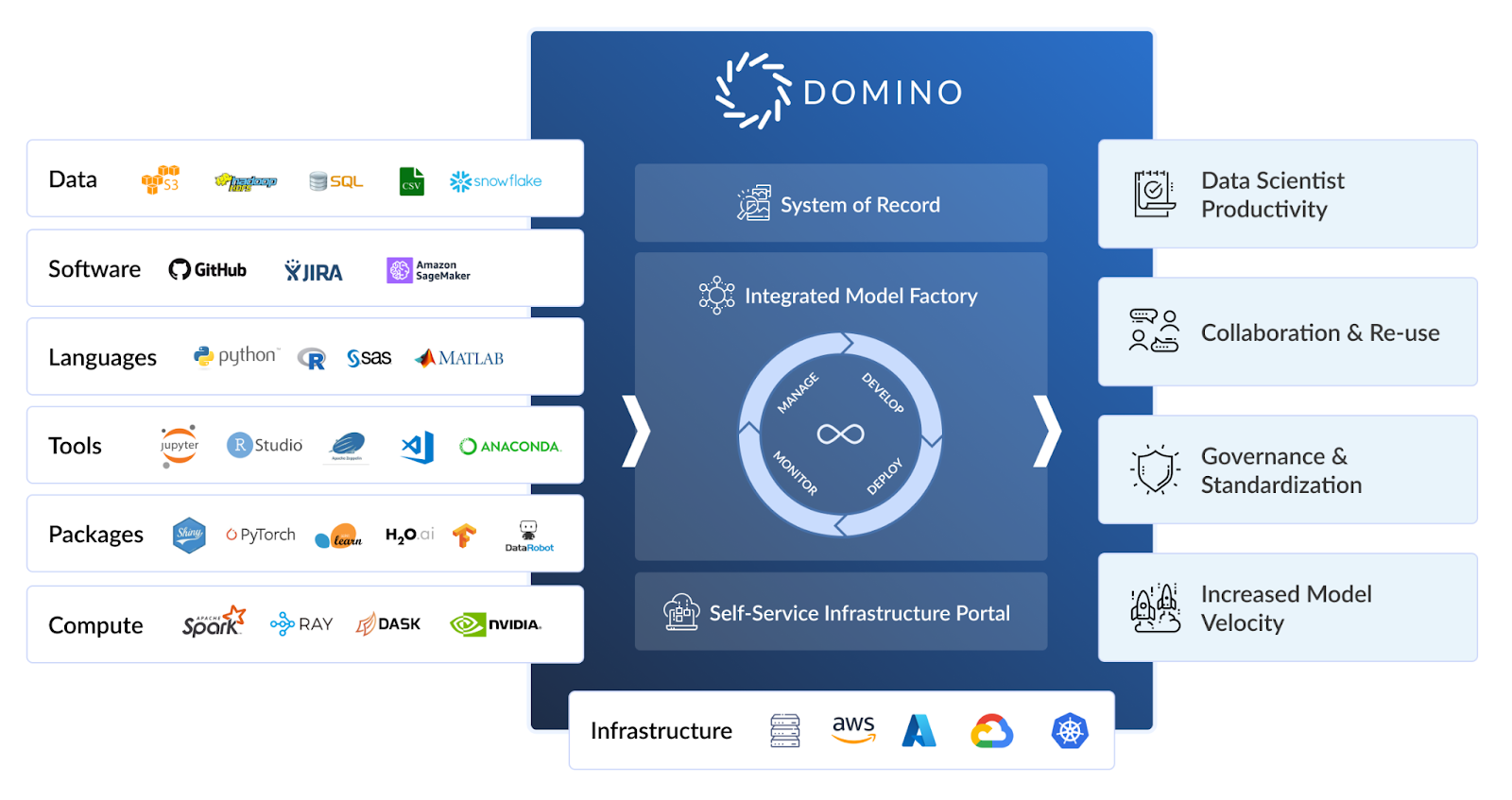
How it Works
Register Data to Enable Model Quality Analysis
Register Predictions
To generate a model quality analysis, Domino first requires you to register prediction data and ground truth data. For models built and automatically monitored in Domino 5.0, Domino automatically registers the prediction data for you. For all other models, follow the instructions provided here.
Register Ground Truth with Cohort Analysis
Next, register ground truth and trigger a model quality analysis. Follow the steps provided here to register ground truth data with Domino and trigger the cohort analysis.
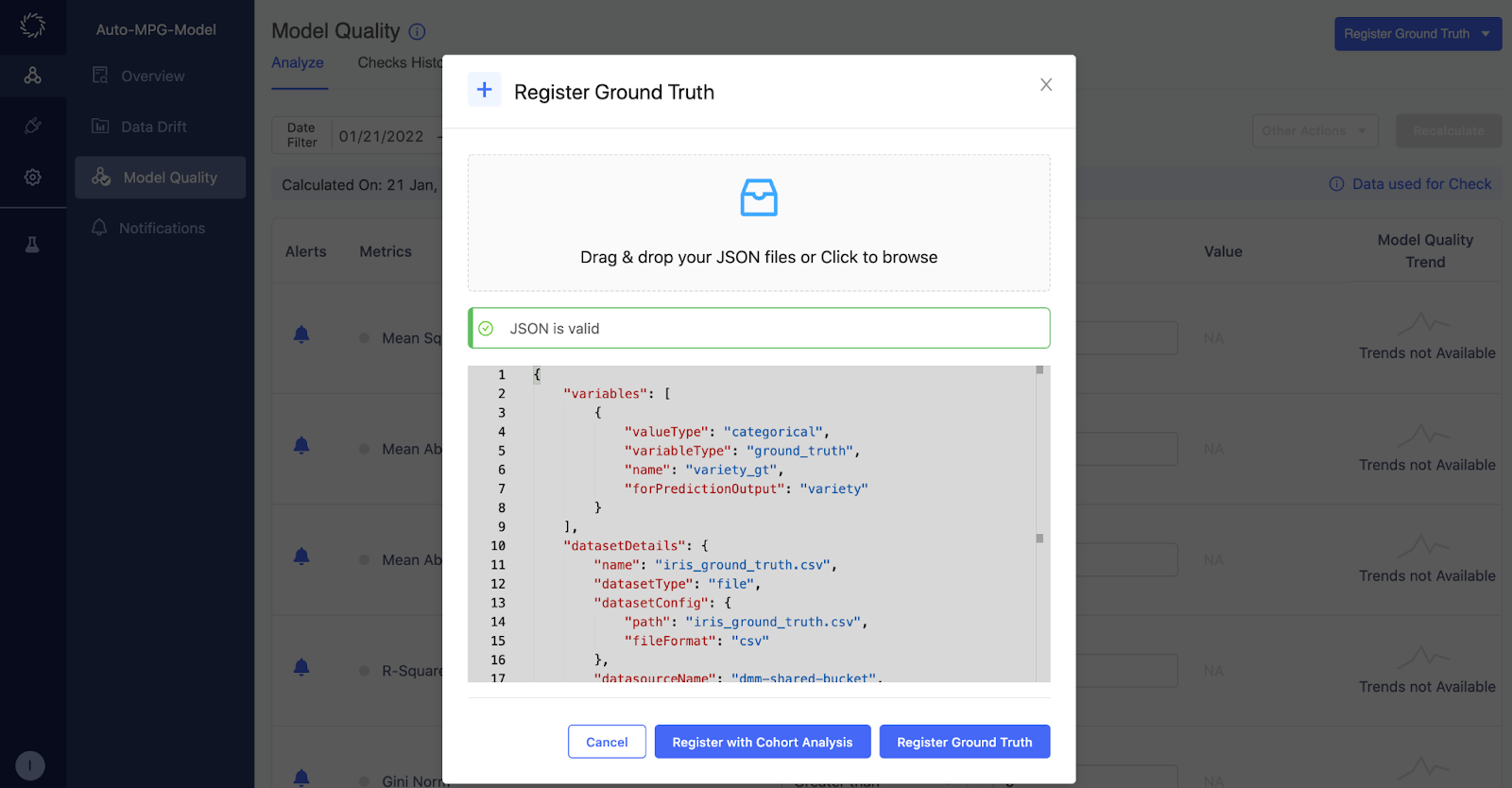
Access Cohort Analysis Report
Once the ground truth dataset has been successfully ingested, the cohort analysis report will be created. You can track the progress of the data ingestion and the cohort analysis generation from within the model’s Overview page.
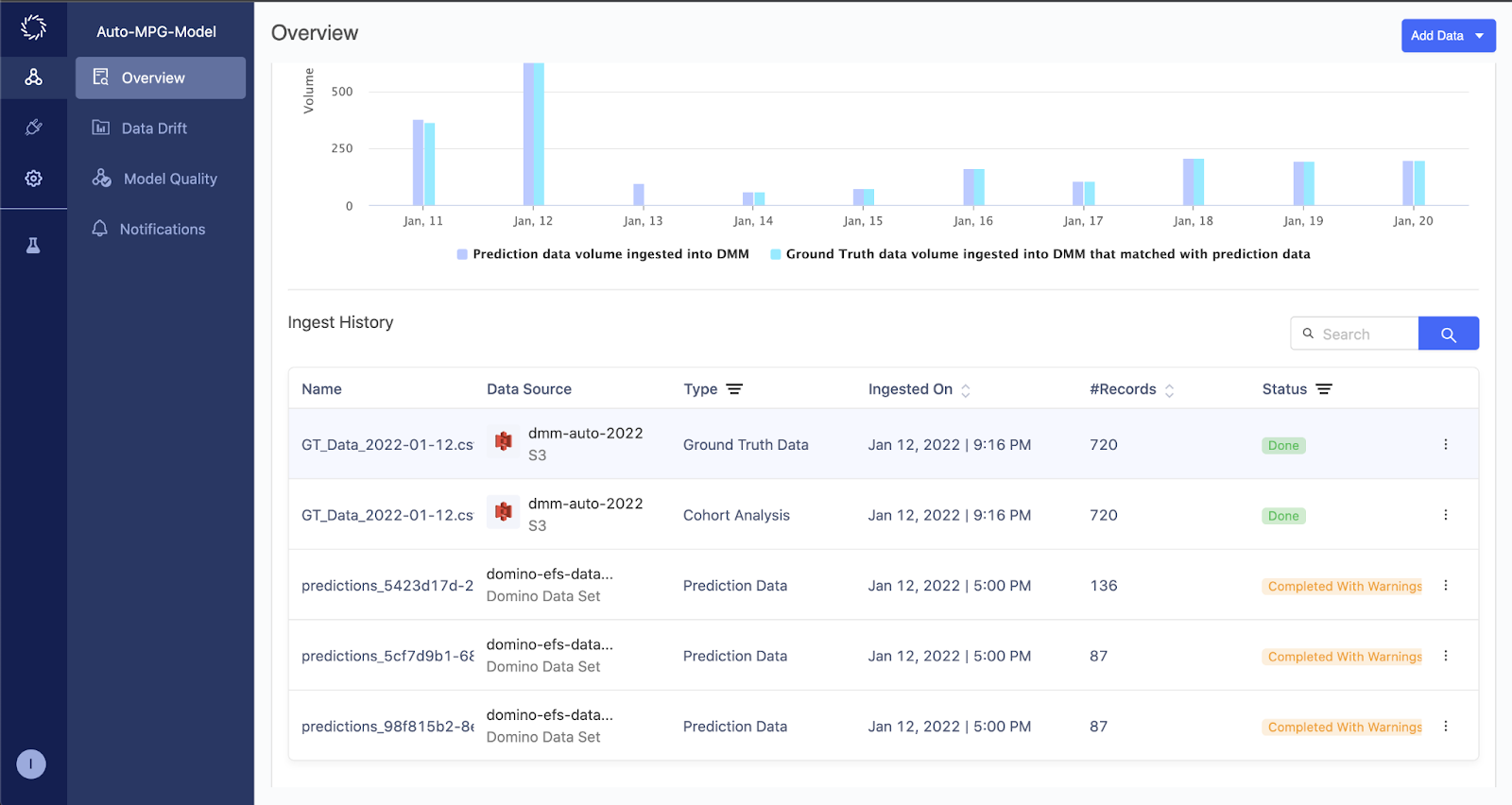
Once the ground truth data and cohort analysis are completed, you can access the reports within the model’s ‘Model Quality’ page.
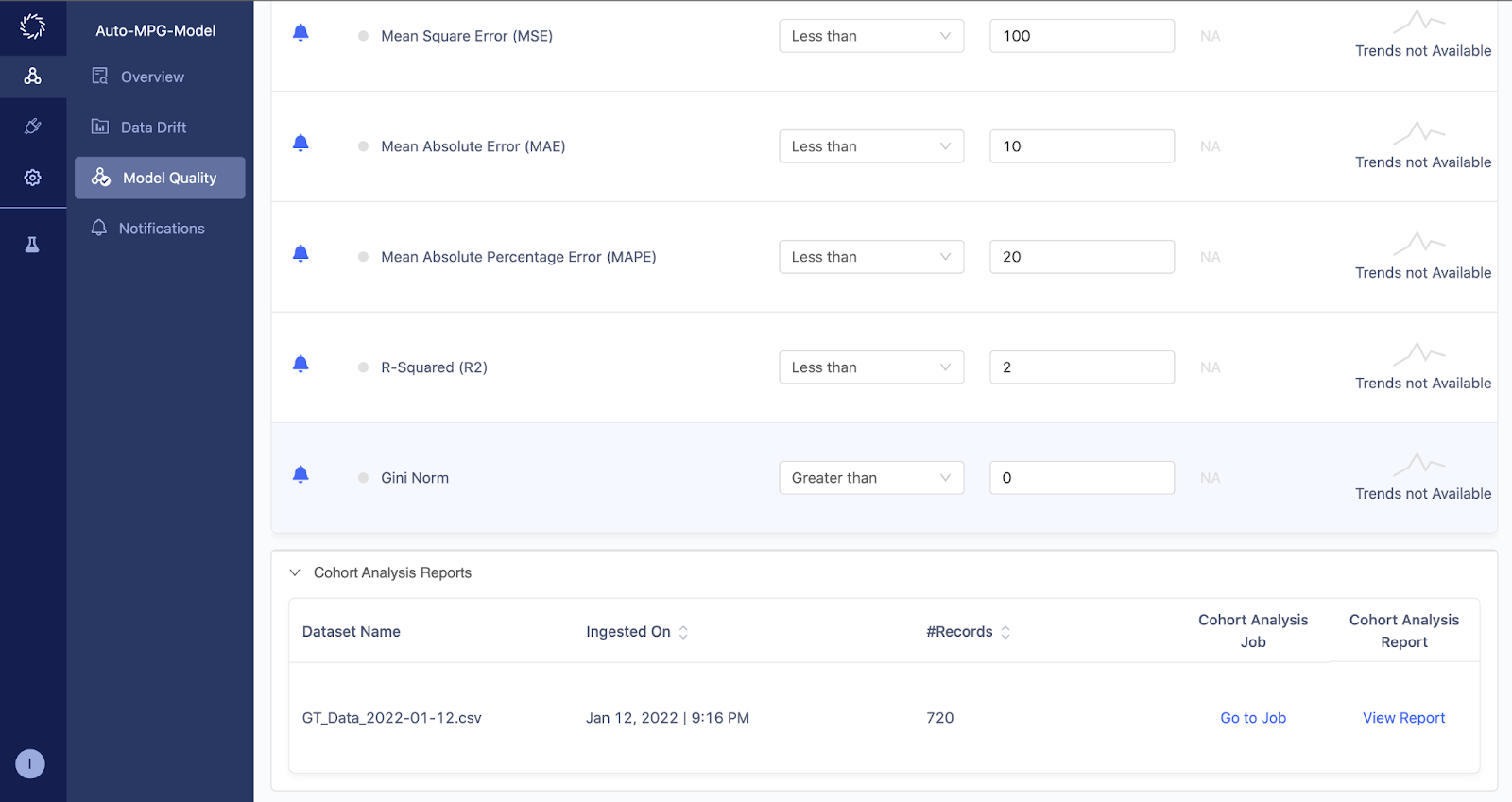
By clicking on the ‘View Report’ link, you can see a PDF that contains the detailed cohort analysis.
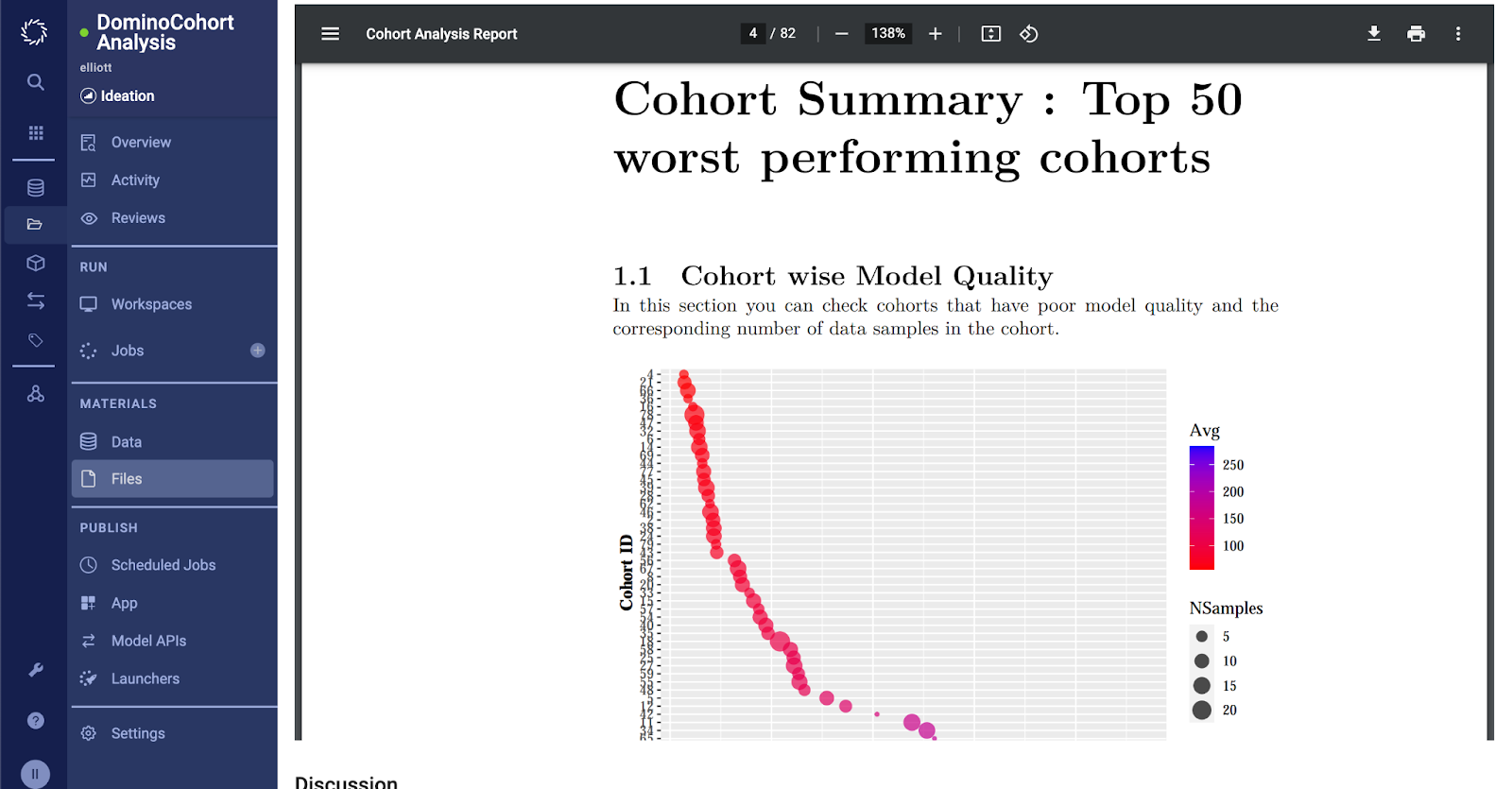
Within this report, you can drill down into each cohort identified in the summary page to understand the primary features that impact the model’s performance.
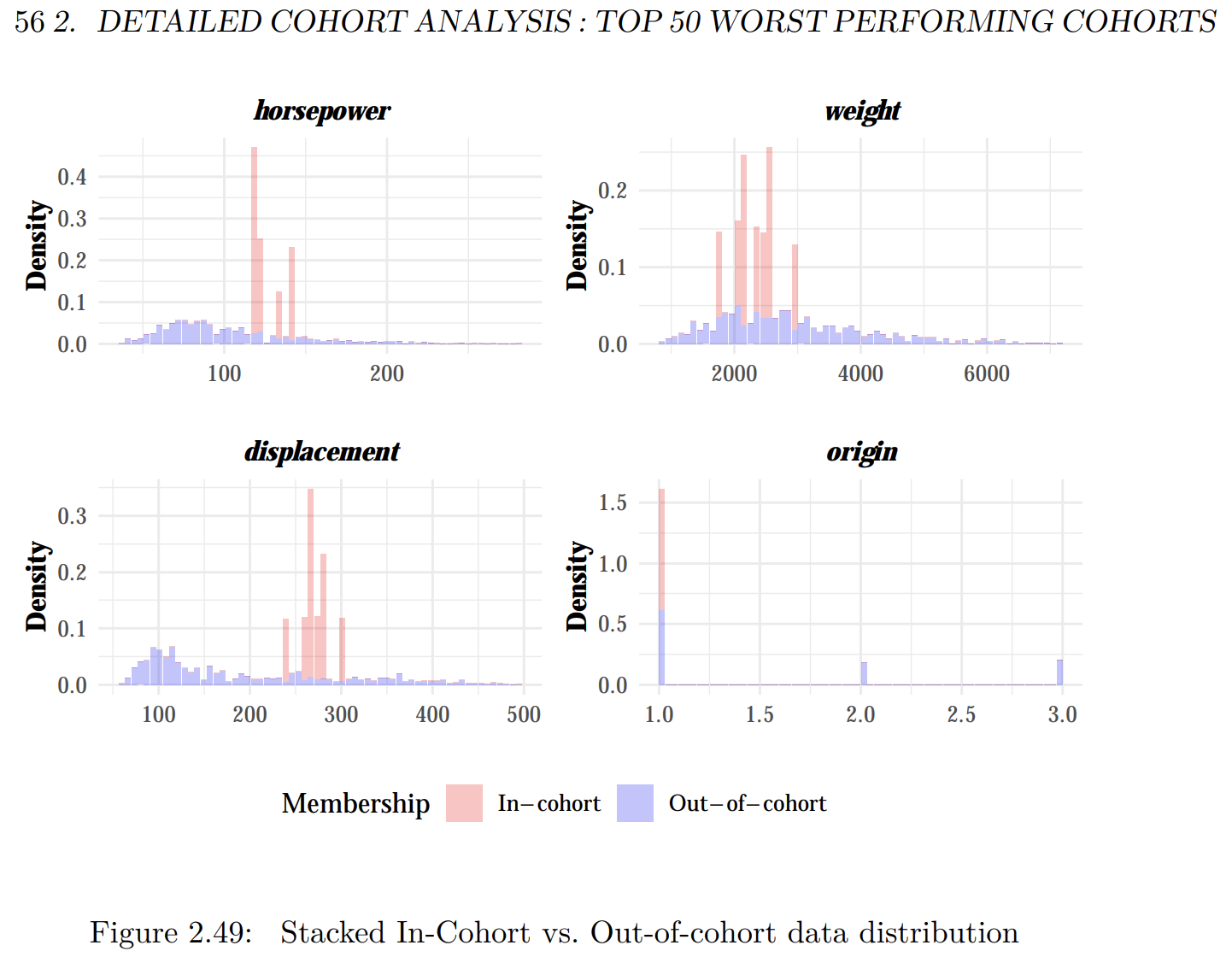
Custom Reports And Analysis
In addition to the easily accessible and downloadable report, Domino also gives you access to the code used to perform the analysis as well as cohort statistics in the form of JSON files. You can make a copy of the code and accompanying statistics to create a custom report or as part of your model remediation efforts.
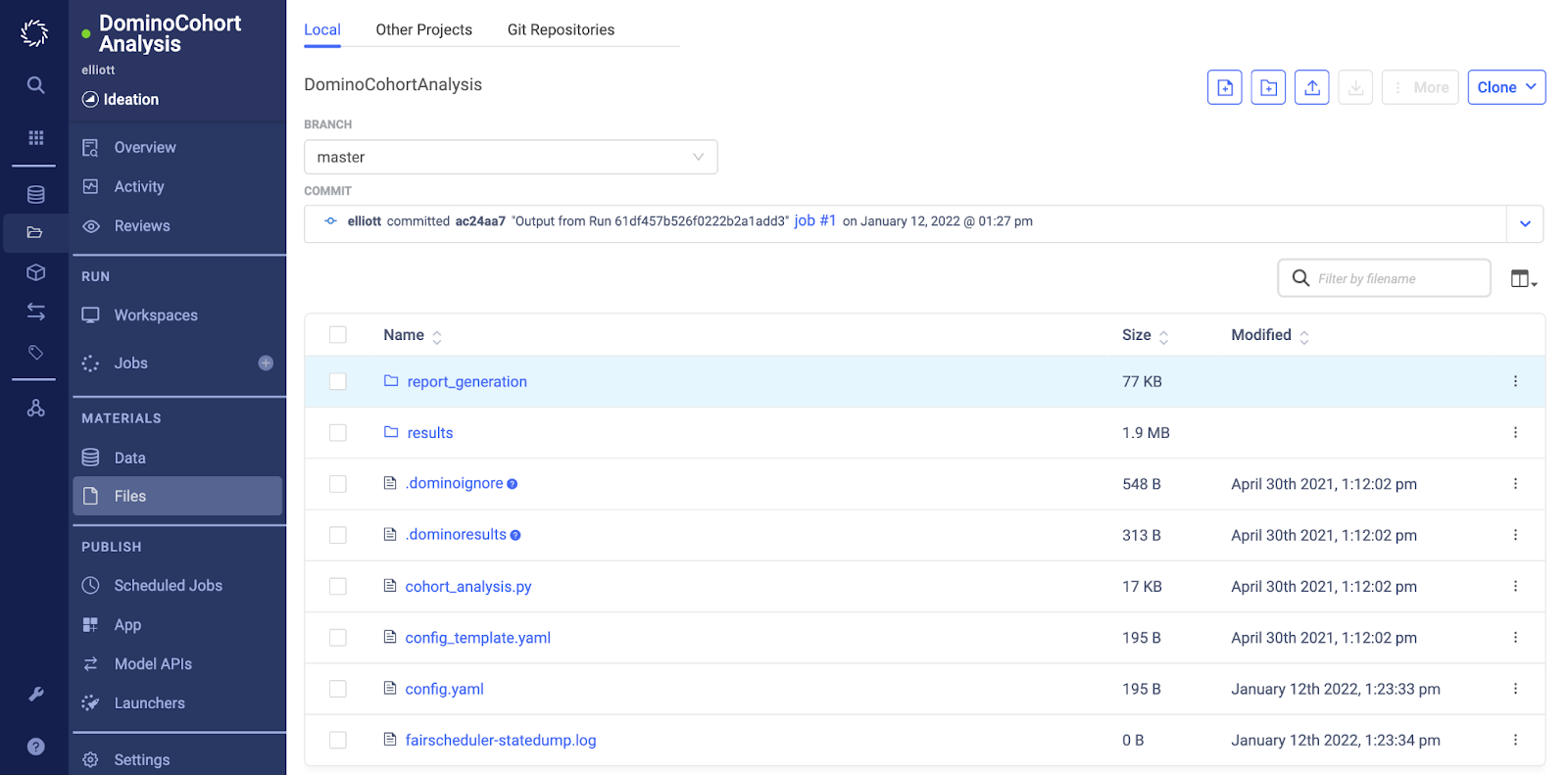
Conclusion
These new capabilities continue to make it easy for experienced data scientists to build high-quality models by providing actionable insights. With the aid of an automated model quality analysis, data scientists are made aware of segments in the data and features within those segments to investigate further as they continue to optimize their model. With this key information, they can choose to retrain their models with updated datasets or adjust the model itself.
Vinay Sridhar is a Principal Product Manager at Domino. He focuses on model productionization and monitoring with an aim to help Domino customers derive business value from their ML research efforts. He has years of experience building products that leverage ML and cloud technologies.
RELATED TAGS