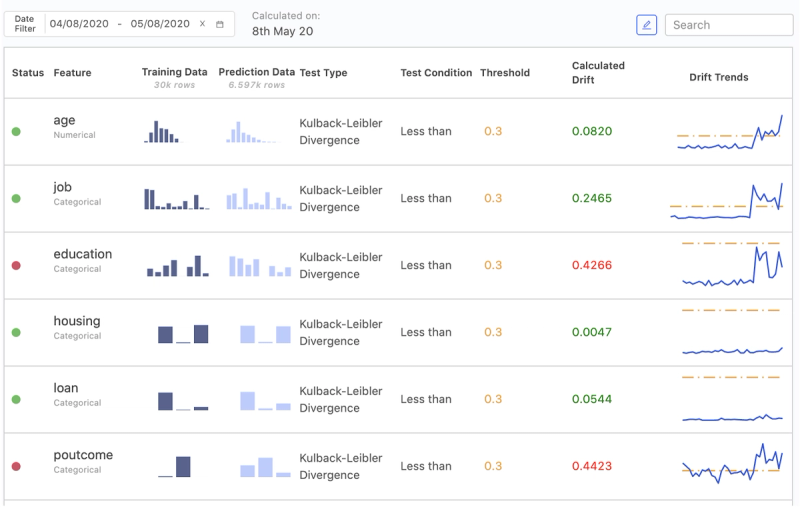
Last week, we announced the latest release of Domino’s data science platform which represents a big step forward for enterprise data science teams. We also announced the availability of an exciting new product – Domino Model Monitor (DMM) – which creates a “single pane of glass” to monitor the performance of all models across your entire organization. DMM gives companies the peace of mind to know that the models they’re basing strategic decisions on are healthy, so there is no negative impact on the business, customer satisfaction, and so on.
Model monitoring is especially critical during today’s unprecedented times; companies’ vital models were trained on data from a vastly different economic environment, when human behavior was “normal”. With DMM, you can identify production data that has changed from training data (i.e., data drift), missing information, and other issues, and take corrective action before bigger problems occur.
Domino Model Monitor unifies the end-to-end model management process with model ops and governance.
The value of data science teams’ work isn’t fully realized until the models they’ve built are operationalized so they can actually impact the business. And once they’re in production, it’s critical to monitor their performance so that they can be re-trained or re-built when needed. In many organizations, this responsibility falls to either the IT team, who has insufficient tools to assess model performance, or the data science team, taking time away from important, value-added projects. Domino is committed to helping this aspect of the data science lifecycle with a solution that helps both teams.
DMM offers complete insight and visibility into the health of all production models across multiple platforms. Gone are the days of worrying about production models left untracked; DMM prevents the financial loss and deprecated customer experience that can occur when production models aren’t properly looked after.
DMM allows companies to view all deployed models across their organization in a single portal, no matter the language, deployment infrastructure, or how they were created (e.g., using Domino or not). It establishes a consistent approach for monitoring across teams and models so you can break down departmental silos, eliminate inconsistent or infrequent monitoring practices, establish a standard for model health metrics across your organization, and facilitate your IT/Ops teams to take charge of monitoring in-production models.
It starts by monitoring production data that’s provided as an input to a model, and compares individual features to the counterparts that were used to originally train the model. This analysis of data drift is a great way to determine if customer preferences have changed, economic or competitive factors have impacted your business, or a data pipeline has broken and null values are feeding a model that was expecting more useful information.
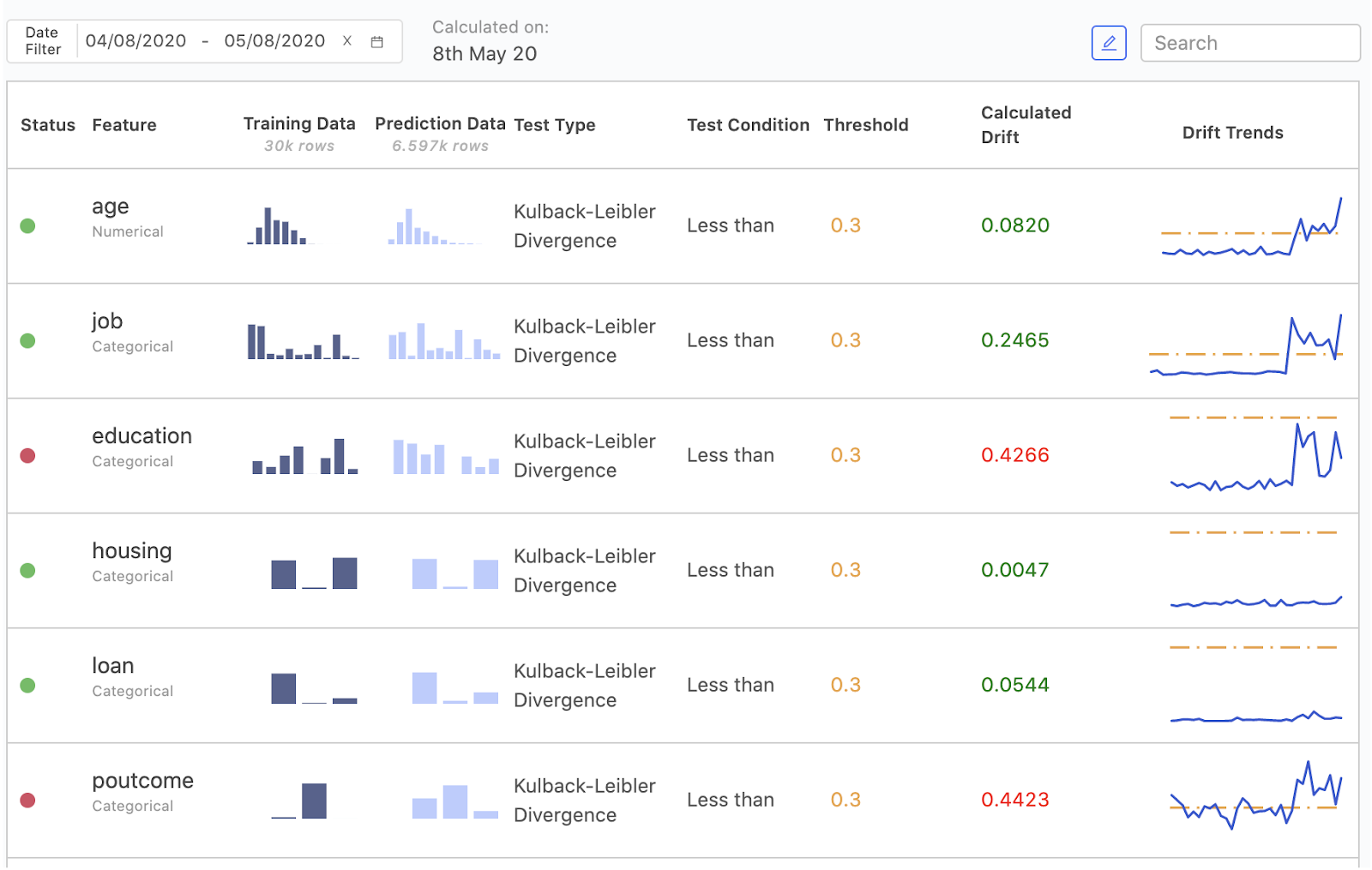
You can also upload live prediction data and, optionally, any ground truth data so that prediction accuracy can be analyzed. If DMM detects data drift or a decrease in model accuracy using a threshold you control, it can provide an alert so data scientists can assess the model and decide what the best corrective action should be. By allowing data science teams to focus on monitoring of potential “at-risk” models, they have more time for experimentation and problem-solving. And IT teams can sleep easier knowing that they have everything they need to deeply understand model performance in an easy-to-understand dashboard.
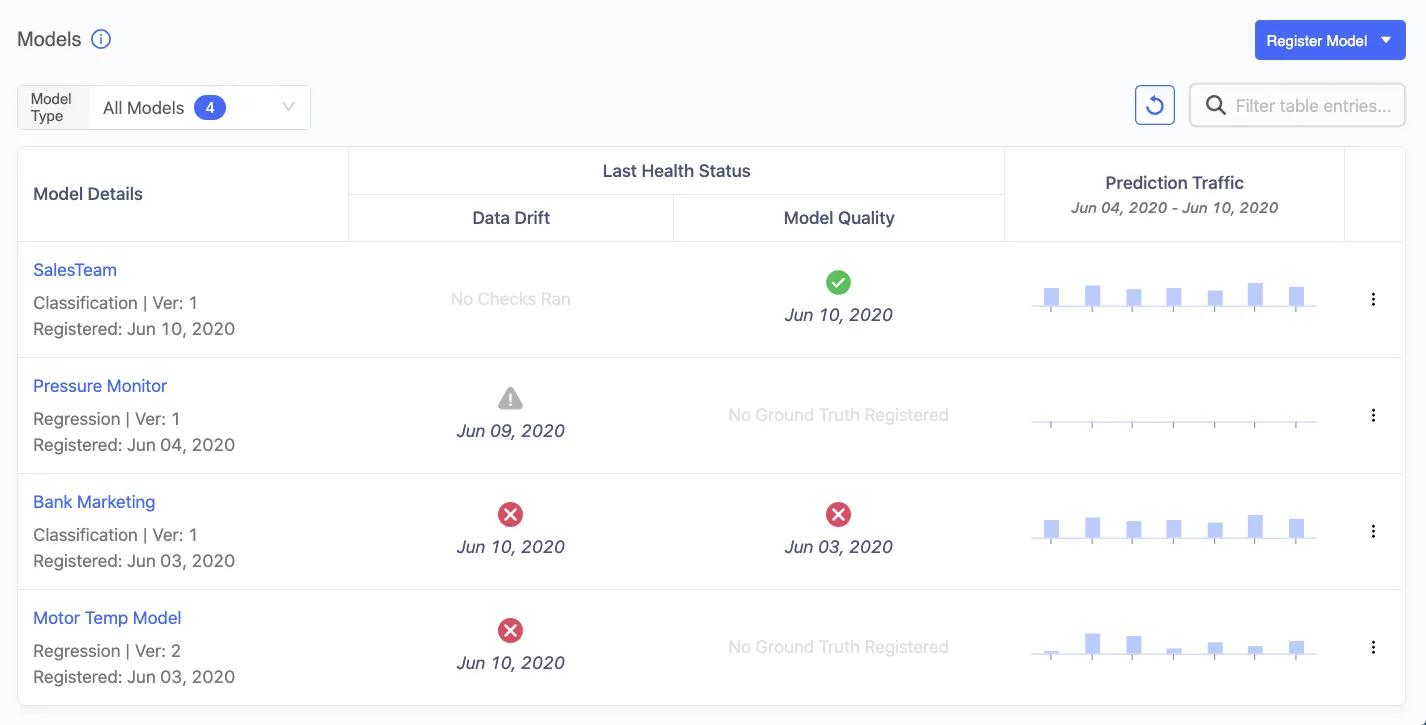
How DMM works
To monitor any model making batch predictions or deployed as an API endpoint, you need to register the model on DMM, configure checks and set up the system for data ingestion. You can schedule the checks for each model to run daily, weekly or monthly, and configure who should receive email alerts when checks fail. DMM ingests production data, and compares them to the original training data to see if any features have significantly drifted. Ground-truth, labeled data can also be ingested and assessed for prediction quality on a regular basis.
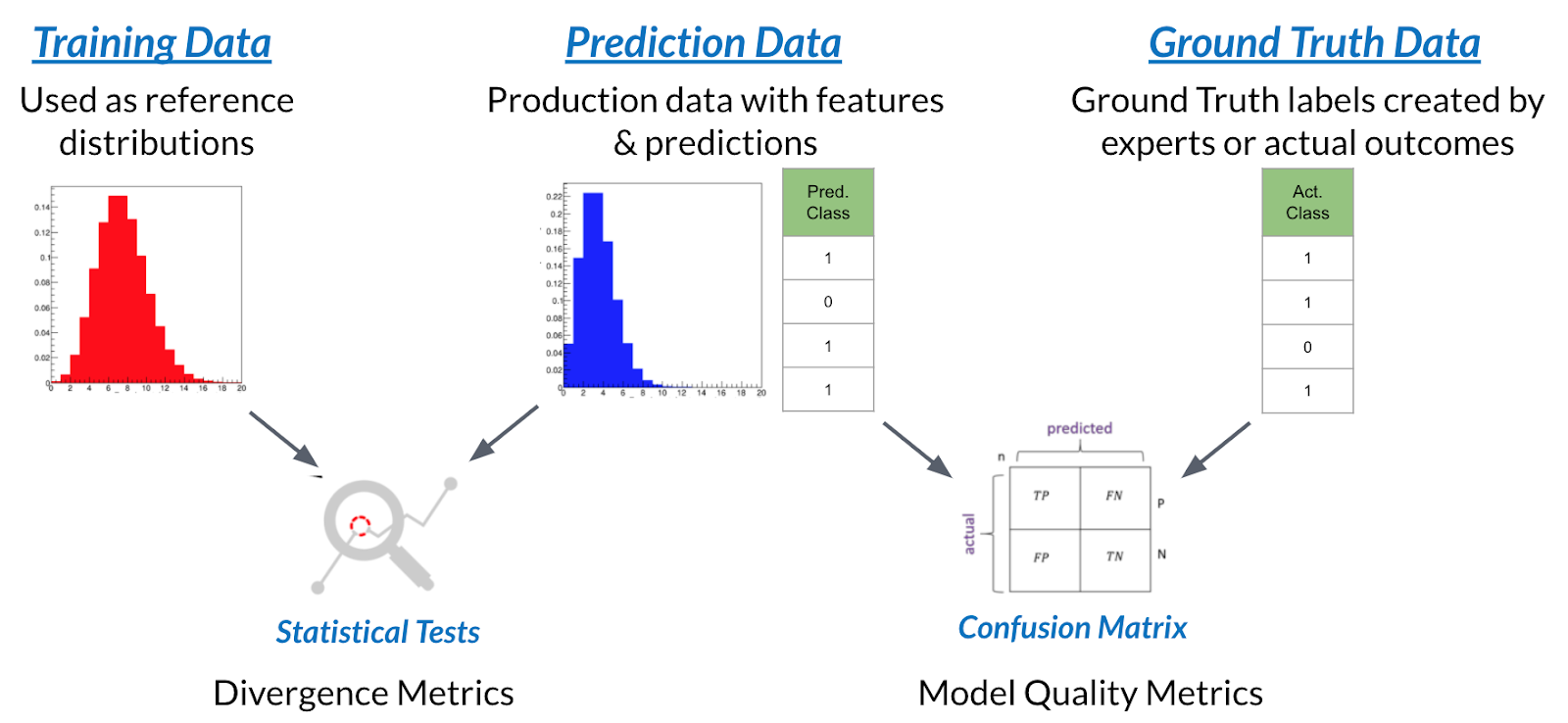
With Domino Model Monitor you can monitor:
- Changes to input features.
- Changes to output predictions.
- Model quality metrics tracking for classification and regression models.
- Historical trends in a production model.
Prevent financial loss and degraded customer experience
The world around us is constantly changing, and the historical data used to train a model may not be reflective of the world we live in today. This is especially true in times like this. Are models built using data from 2018-2019 good predictors of what will happen during a pandemic in 2020? Now more than ever, companies need to be aware of shifts in buyer preferences, economic changes, and other external factors outside their control that render their models obsolete.
Internal changes can also cause models to degrade, in some cases quite significantly. For example, at a global insurance company, inadvertent changes in an upstream data pipeline caused a fraud detection model to make suboptimal predictions and increase claims payments for weeks. DMM helped them detect the data drift so they could update the model with the latest pipeline changes, and prevent further overpayment of claims.
DMM gives companies the ability to detect changes without having to dedicate expensive and scarce data science resources to constantly check each model. They can have a dashboard with details about the health of all models in production, and drill in to get details. And even if they don’t want to monitor the dashboard, they can set thresholds so that proactive notifications can be sent out to notify key people (including the data scientist who originally built the model) that it’s time to either retrain it using updated data from today’s world, or completely rebuild it using a new algorithm. DMM gives companies the peace of mind to know that the models they’re basing strategic decisions on are reflective of today’s world, not the world when the model was built – minimizing economic impact to their business, keeping their customers happy and engaged, etc.
Next steps
The team here at Domino has put a lot of energy into designing and developing DMM, and we’re excited to bring this new product to data science teams who are working to optimize health and processes across their models. We appreciate the ongoing feedback received from customers and friends throughout the development process and are inspired to keep improving Domino to solidify its place as the best-in-class system of record for data science in the enterprise.
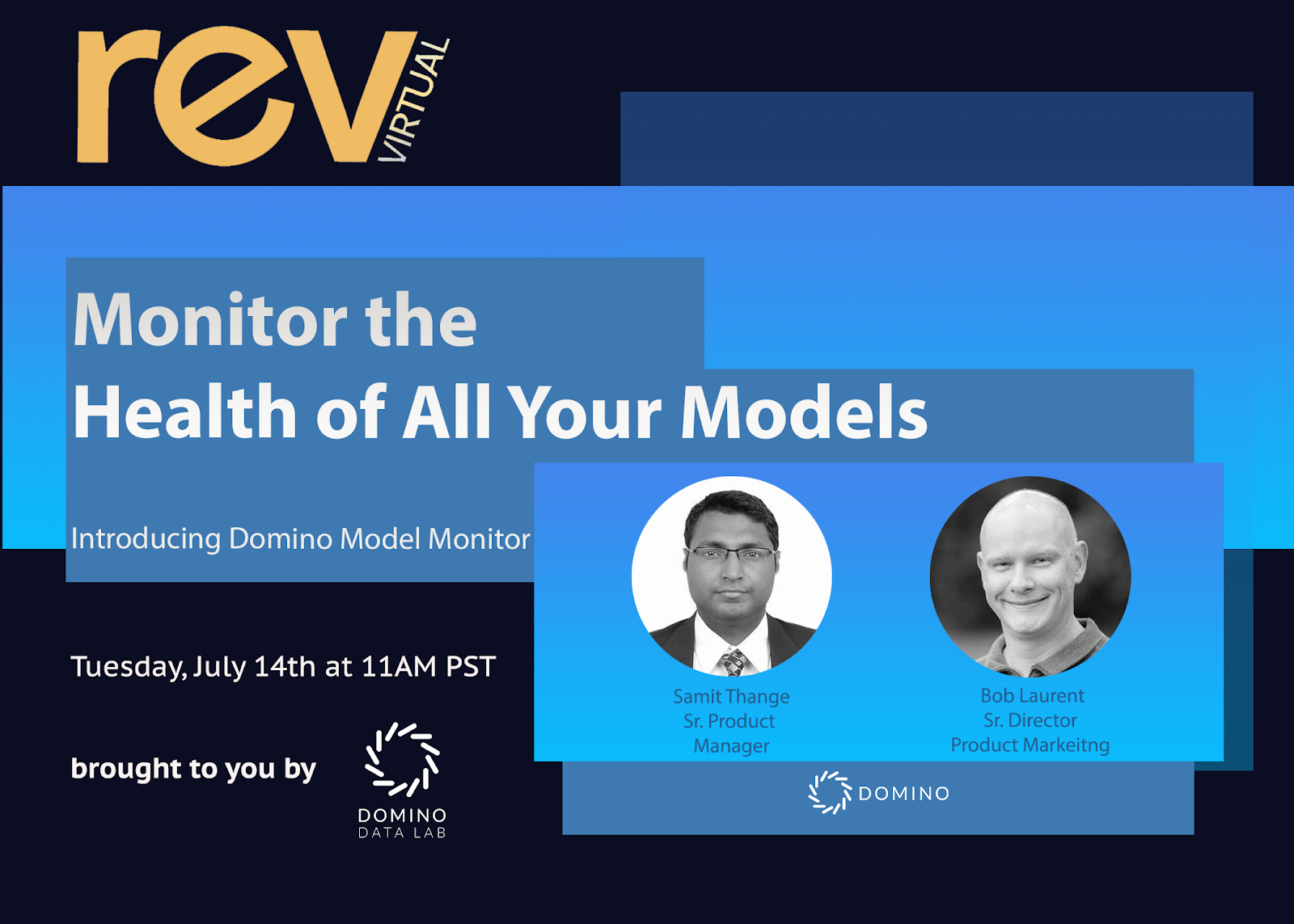
Bob Laurent is the Head of Product Marketing at Domino Data Lab where he is responsible for driving product awareness and adoption, and growing a loyal customer base of expert data science teams. Prior to Domino, he held similar leadership roles at Alteryx and DataRobot. He has more than 30 years of product marketing, media/analyst relations, competitive intelligence, and telecom network experience.
RELATED TAGS
Subscribe to the Domino Newsletter
Receive data science tips and tutorials from leading Data Science leaders, right to your inbox.
By submitting this form you agree to receive communications from Domino related to products and services in accordance with Domino's privacy policy and may opt-out at anytime.