Life Sciences Teams: Avoid These Common Pitfalls on Your Path to the Cloud
Tim Hughes2020-07-09 | 10 min read
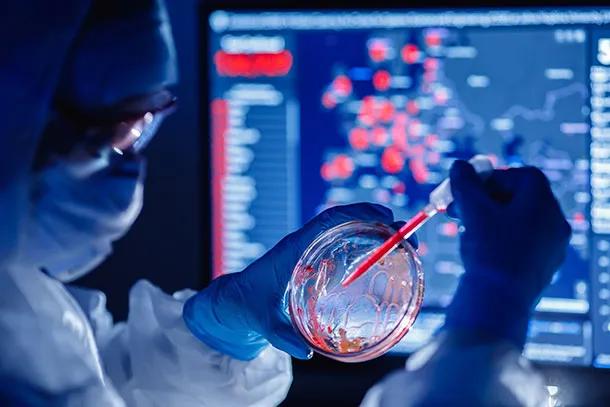
Many life sciences organizations are in the midst of digital transformation efforts. A key component of this is moving existing on-premises IT platforms, statistical computing environments (SCEs), and associated programming languages and tools into the cloud. Research and Data Science functions represent low-hanging fruit for these cloud migrations because their workflows necessitate dynamic access to compute infrastructure and centralized access to diverse toolsets.
The process of centralizing data science functions and their surrounding tools offers two important opportunities: addressing the challenges that have grown over time out of traditional data center and desktop-based analytics environments, and “hard coding” best practices into the process through automation and underlying technologies.
The benefits of running data science in the cloud are massive:
- Significant cost savings are possible when your organization has access to high-powered compute resources when they’re needed but only pays for what’s used.
- Standardizing and centralizing access to tools means that IT can effectively manage and govern the technology landscape across teams, providing improved security and compliance.
- Dramatic productivity gains associated with baking best practices into underlying technologies can be achieved on three levels: Automatic reproducibility of research and associated artifacts accelerates employee onboarding, alleviates manual documentation, and facilitates cross-team collaboration and accessibility of past work.
But there’s a reason migrating to the cloud remains on the “to do” list of many life sciences organizations. It’s a complicated process that takes time. SCEs usually consist of many different deployments of many different technologies, all of which have to satisfy internal and external requirements around security and compliance, such as HIPAA.
If your team is starting on the path to the cloud, here are some common pitfalls we’ve seen in the field. By bringing awareness to these challenges, we hope you can develop mitigation strategies in advance and get to the cloud faster. We also encourage you to read our whitepaper offering best practices for digital transformation in life sciences, based on our experience working with enterprises that have been successful on this journey.
1. Researchers can’t access the compute resources they need.
Because of the DevOps headaches involved in procuring access to large server environments, researchers end up doing most of their work on laptops that struggle to keep pace with exponentially growing data volumes.
Researchers are frustrated, wasting their precious time jumping through infrastructure hoops, and the organization’s research environment becomes a tangled web of “shadow IT” and fractured silos.
2. The technology landscape for data science tools is constantly changing, creating a latency between when new technologies are available and when researchers can access them.
Researchers want — and have every right — to use the latest and greatest tool for the task at hand. There’s a ton of innovation happening in the space. New and improved tools are introduced almost daily, many of them open source. Meanwhile, there are many users who want to use more traditional, proprietary technologies like SAS and MATLAB.
Every tool drives business value, but each has its limitations, especially if it isn’t integrated into an enterprise-wide data science strategy. SAS and MATLAB are powerful and trusted by large enterprises in regulated environments, but they’re limited in their ability to work with open source technologies. They also often replicate data which can require expensive data storage infrastructure.
On the other hand, open source tools such as R and Python streamline deployment and administration by leveraging containers and orchestration tools, which can reduce storage costs. They’re also accessible to a broader talent base. But open source tools struggle with relatively slow data access, challenges with recoding and version controls, and don’t offer the same governance capabilities over data, models and processes as their proprietary counterparts.
Data scientists can’t afford to lose time waiting for third parties or system admins to make changes or get access to new technologies, but IT needs to make sure new technologies are introduced to the organization safely and with necessary governance.
How can IT provide access to the wide range of technologies while ensuring they meet corporate mandates and regulatory requirements? How can they provide an environment where researchers can combine a string of job executions across different tools without causing issues? How can teams maintain a reproducible chain of workflows spanning SAS, Python, R and other libraries to support a variety of stakeholders and business cases?
A backlog inevitably builds up, leads to frustration between researchers and IT, and contributes to the “shadow IT” situation where researchers are not only limited to the compute resources on their laptops, but they’re also running multiple SAS, R, Python and MATLAB silos.
3. IT can’t keep pace with the modern delivery model for cloud-based software releases.
Especially in life sciences environments dealing with sensitive data, IT has traditionally spent four to eight months validating software upgrades for each new release of on-premises systems. They’re used to this predictable upgrade cycle; they can plan for it. But cloud-based software vendors aren’t bound to the old ways of operating, and it’s now commonplace to deploy new software versions several times per year.
IT teams haven’t yet figured out how to adjust to the new cadence, and every upgrade consumes multiple support staff, admin resources or capabilities that would otherwise be spent on valuable research workflows.
This is exacerbated by the fact that IT doesn’t have a good handle on what version of each tool is in use across the many siloed systems. Trying to keep up and provide support for distributed teams leads to a huge support overhead. We commonly hear IT leaders comment that they have one team that’s dedicated solely to supporting rolling upgrades across all of the commercial tool installations throughout the organization.
4. Unnecessary friction and duplicated work result from siloes of activity and inconsistent version controls.
Especially with teams geographically distributed, people are recreating the same work in pockets across every organization without realizing it. Without being able to see what has been created in the past, researchers start every project from scratch or ask others about past work in one-off settings.
When researchers ask others to engage in peer reviews, it’s nearly impossible to know what versions of R, Python, and/or SAS were used for a given analysis. This also makes reproducibility of past work a complex task.
5. Semi-manual version control wastes a lot of time.
Time spent on manual or semi-manual processes translates into less time spent furthering the analysis or moving onto the next project. And even when researchers implement manual methods for version control, it exists at the source code level but not at the experiment level; software versions, etc., are not captured. Satisfying requirements for model provenance is painful, demanding a lot of ad hoc efforts to manually audit work documents and the specific versions of tools that led to a particular analysis and outcome.
6. Data science leaders struggle to allocate resources efficiently to projects with limited visibility.
Data science leaders are responsible for allocating the right data scientists to projects, but this is often done on a whim or based on anecdotal, ad hoc inputs from researchers and line of business stakeholders. Data science leaders have a hard time gaining transparency and understanding the state of work across their team and/or the broader organization.
Further, if they determine a need to reassign resources to different projects, the work it takes to get new contributors up to speed is seldom worth the effort. It ultimately takes longer to deliver high-quality outcomes and becomes hard to measure the efficiency of data science teams.
The light at the end of the tunnel.
Many industry-leading organizations are finding success navigating these challenges while they work their way to the cloud. It’s important to be aware of these challenges and account for them as part of a bigger strategy to centralize research and data science functions, leveraging technologies that can empower researchers to use their preferred tools, access compute when they need it, and automate manual work that can lead to inconsistencies and inefficiencies at scale.
For an in-depth look at best practices adopted by successful life sciences enterprises that implement a future-proof data science practice in the cloud, read the whitepaper:
Summary
- 1. Researchers can’t access the compute resources they need.
- 2. The technology landscape for data science tools is constantly changing, creating a latency between when new technologies are available and when researchers can access them.
- 3. IT can’t keep pace with the modern delivery model for cloud-based software releases.
- 4. Unnecessary friction and duplicated work result from siloes of activity and inconsistent version controls.
- 5. Semi-manual version control wastes a lot of time.
- 6. Data science leaders struggle to allocate resources efficiently to projects with limited visibility.
- The light at the end of the tunnel.
RELATED TAGS