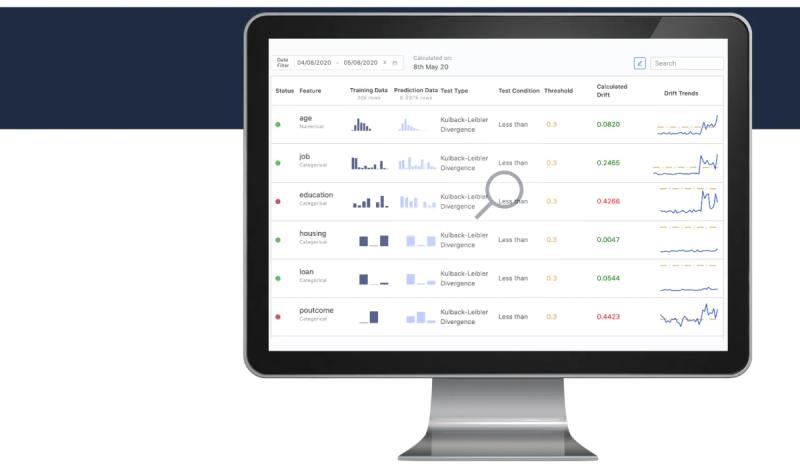
By Samit Thange, Senior Product Manager, Domino on July 31, 2020 in Product Updates
During our recent Rev Virtual webinar, Monitor the Health of All Your Models, we discussed the challenges many organizations have identifying when they need to retrain or even rebuild the models they depend on for critical business decisions. This is a problem that I hear quite often, and not surprisingly, the event attracted hundreds of attendees across numerous industries.
I also demonstrated our newest product, Domino Model Monitor (DMM) during the webinar. The interest and volume of questions from attendees was so high that we weren’t able to address all of them during the live event. This blog provides answers to some of the most commonly asked questions. We encourage you to read the whitepaper for best practices on model monitoring, or sign up to trial DMM first hand.
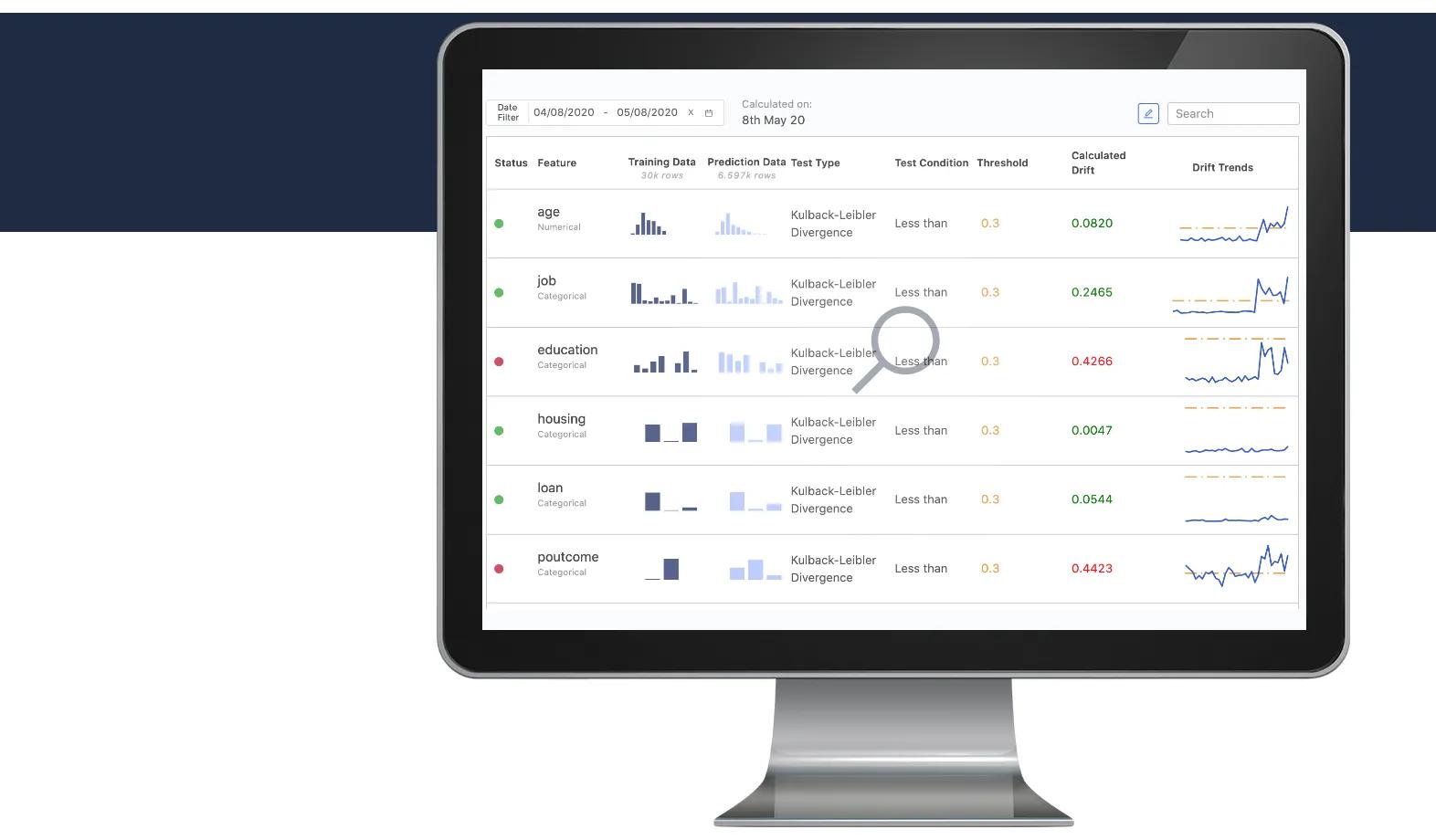
1) Can you register models in DMM that were created outside the Domino Data Science Platform?
Yes, you can register and monitor models built outside the Domino platform. A key value proposition of DMM is that it enables you to monitor business-critical models regardless of the technology or platform used to build them or where they are deployed. So, for example, you could have teams of data scientists in different departments building models using R Studio, SAS Studio, MATLAB, AWS SageMaker, and other tools, and all of the models they produce can be monitored in one dashboard within DMM.
2) Is this a containerized solution that can be extended as needed in an orchestrated environment?
Yes, DMM is a Kubernetes-native app. That makes it easy to add more infrastructure in order to run more containers if needed for your workloads.
3) What types of models can DMM monitor?
DMM can be used to monitor regression models, as well as binary and multi-class classification models. In the case of regression models, instead of accuracy or precision, metrics such as root mean square error, mean absolute error, etc. are used to track the model’s prediction quality. DMM can also be used to monitor output prediction drift and the model’s prediction quality for text and image classification models.
4) Is there a limit on the number of models that can be monitored?
No. The number of models and data that DMM can handle depends on the deployment sizing.
5) What does the drift trend chart measure? How can it be interpreted side by side with the drift measure?
When you are analyzing drift in DMM, the drift trend chart shows how drift for a feature has changed on each day of the selected time period. The aggregate drift number captures the overall drift for all the data of that time period.
6) How is drift calculated – is it aggregated or just a comparison of the start and end times?
DMM calculates drift on the aggregated data. For example, if you are looking at data for the last seven days, it will construct aggregated histograms for all input features and output predictions made in all seven days, and compare them with training data to calculate the drift.
7) How can user input on correctness of predictions be hooked with DMM?
In DMM, you can ingest ground truth labels in the same way as prediction data. Say you have a process of generating these labels once every week (either using human experts or system generated). You can put them in a CSV file (you need 2 columns – GT label and Prediction ID) in your AWS S3 bucket and make an API call to DMM with the path of this file. DMM will do the rest.
8) For data ingestion, can we link to an on-premise data source directly, for instance, any SQL Server environment?
Not out of the box today. Depending on the particular on-prem SQL database you use, Domino will add a data connector that would allow you to ingest directly. Ask your Domino Customer Success Manager to have your particular database connector prioritized in the DMM roadmap.
9) Our dataset sizes are in the terabytes (TBs). Will DMM be able to support them?
Data size limits depend on the size of the cluster provisioned for DMM. If your data sizes are larger than the cluster can support, you can down-sample the data and ingest it into DMM. For example, if a raw data stream has 10M+ predictions, our observation has been that ingesting 1M predictions per day will be sufficient to provide a reliable drift signal for the model. As part of the deployment, Domino will work with you to estimate a good baseline cluster size, and more compute resources can be added later if required.
10) How does the data source get connected to DMM?
There is a dedicated UI in DMM through which you can add a new data source and manage existing ones. While adding a new data source, DMM will ask for the necessary metadata and authentication details as part of the data source addition. Whenever data is to be read from this source, the provided information will be used to establish a connection and read the dataset.
11) Can the data for inputs and outputs be read in from an Apache Kafka stream for real-time models?
Today data can only be ingested using the REST APIs. However, ingesting data through Kafka is something we are looking at. If the Kafka use case is important for you, please connect with your Domino Customer Success Manager to register that feedback.
Additional resources
For more information about DMM or model monitoring in general, I encourage you to read our new whitepaper for best practices on model monitoring. If you missed the webinar you can watch the on-demand session: Monitor the Health of All Your Models. And, if you like what you see you can sign up to trial DMM first hand.
Summary
- 1) Can you register models in DMM that were created outside the Domino Data Science Platform?
- 2) Is this a containerized solution that can be extended as needed in an orchestrated environment?
- 3) What types of models can DMM monitor?
- 4) Is there a limit on the number of models that can be monitored?
- 5) What does the drift trend chart measure? How can it be interpreted side by side with the drift measure?
- 6) How is drift calculated – is it aggregated or just a comparison of the start and end times?
- 7) How can user input on correctness of predictions be hooked with DMM?
- 8) For data ingestion, can we link to an on-premise data source directly, for instance, any SQL Server environment?
- 9) Our dataset sizes are in the terabytes (TBs). Will DMM be able to support them?
- 10) How does the data source get connected to DMM?
- 11) Can the data for inputs and outputs be read in from an Apache Kafka stream for real-time models?
- Additional resources
RELATED TAGS
Subscribe to the Domino Newsletter
Receive data science tips and tutorials from leading Data Science leaders, right to your inbox.
By submitting this form you agree to receive communications from Domino related to products and services in accordance with Domino's privacy policy and may opt-out at anytime.