Seeking Reproducibility within Social Science: Search and Discovery
Ann Spencer2019-07-22 | 25 min read
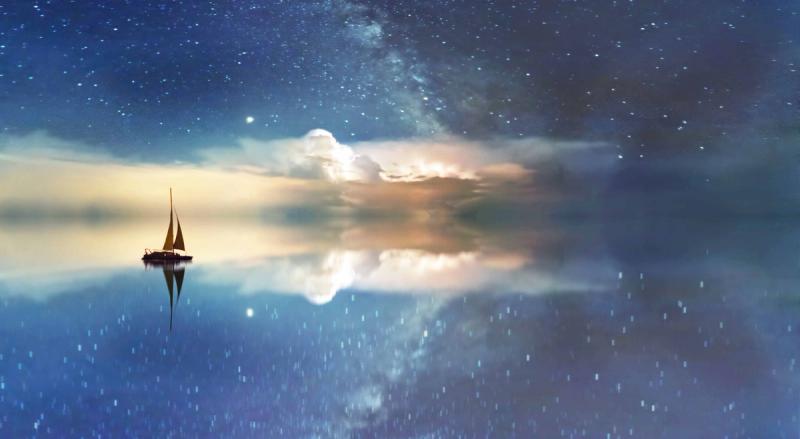
Julia Lane, NYU Professor, Economist and cofounder of the Coleridge Initiative, presented “Where’s the Data: A New Approach to Social Science Search & Discovery” at Rev. Lane described the approach that the Coleridge Initiative is taking to address the science reproducibility challenge. The approach is to provide remote access for government analysts and researchers to confidential data in a secure data facility and to build analytical capacity and collaborations through an Applied Data Analytics training program. This article provides a distilled summary and a written transcript of Lane’s talk at Rev. Many thanks to Julia Lane for providing feedback on this post prior to publication.
Session Summary
Science is facing a research reproducibility challenge that hampers data scientists’ and researchers’ ability to accelerate their work and provide insights that impact their organizations. Since Domino’s inception, we have tackled the reproducibility problem to support our customers via continued updates to the platform's collaboration functionality as well as contributing to the overall public discourse on this blog and at industry events including Rev. In the Rev session, “Where’s the Data: A New Approach to Social Science Search & Discovery”, Julia Lane provided insights into how the Coleridge Initiative is addressing the reproducibility challenge by providing secure remote access to confidential data for government analysts and researchers and to build analytical capacity and collaborations through an Applied Data Analytics training program. The goal is to enable government agencies to make better evidence-based policy decisions with better quality data. Lane, staying true to the ethos of reproducibility, covered how the approach could be used to allow approved analysts to reuse and ingest insights to accelerate their work. Lane discussed the questions Coleridge sought to answer; to improve the analytical rigor associated with working with linked data; how Coleridge built and tested a set of tools that could be applied to identify what data have been used to address different research questions; and how the approach can be used to inform new researchers as they access the secure environment with new projects. Lane closed the session with how the intention of initiative “is to build evidence-based policy making whereby we get better knowledge, get better policy, get resources allocated better, and reduce the cost and burden of collecting information.”
A few highlights from the session include
- how modern approaches can be used to obtain better quality data, and at a lower cost, to help support evidence-based policy decisions.
- unique challenges of sharing confidential government microdata and the importance of access in generating high quality inference
- pragmatic evaluation of risk-utility tradeoffs, or the tradeoff in risk resulting from greater data usage versus the risk of disclosure or reidentification
- a discussion of how Coleridge used the training classes to build the capacity of government agency staff to address data quality challenges
- the importance of pairing training for researchers with access in a secure environment.
Additional insights are available in the written transcript.
Transcript
Julia Lane:
I'm an economist by training and that is where my focus is going to be. Let me tell you the story. I'm at NYU, as you can tell by my strong New York accent, and I've spent most of my career working with federal statistical agencies, the agencies that bring you the Decennial Census, the unemployment rate, GDP, and so on. A major challenge that you face with using those kinds of data for decision making is data is very expensive to collect and the quality of the data is going down.
How many of you are familiar with the fact that the Decennial Census is going to be fielded next year? Okay. Roughly how many people do you think there are in the United States? 350, 360 million, we'll find out more maybe next year. How much do you think it costs to collect…count that number of people? $360 million. Try north of that. $2 billion, do I hear two? Okay. Anyone want to go higher? Higher, more, more. $17 billion, okay, to count the number of people and ask them 10, maybe 11 questions. Right? The challenge that we have is that the quality of the data is going down. People aren't responding, they're giving bad responses, and so on.
About three years ago, Evidence-Based Policymaking commission was established, which brought together experts from around the country to figure out how can you develop data to make decisions at better quality and lower cost,. They came up with a set of recommendations, that got passed into the Evidence-Based Policymaking Act this year.
They asked NYU to build a s to build an environment to inform the deliberations of the Commission, and show how you can bring together data from multiple sources and make sense of them at the level of quality that you need to be able to allocate resources to make decisions. That's why it's called evidence-based policy.
Of course, the challenge with bringing data together is that the data, particularly on human beings, is quite complicated. When it is generated from multiple different sources, it's not just a matter of mashing them together. You need to understand how they were generated, what the issues are and so on. Together with that, we built training classes to train government agency staff and researchers and analysts who want to work with the data what the issues…how to work with them.
Here's the challenge. When you're working with complicated data and when it is confidential, because it is data on human beings, it's very difficult to find out what work has been done on them before. Every time someone starts working with the data, it's a tabula rasa. You can't figure out what's going on. The challenge that we had to solve is the challenge I'm going to talk to you about today, which is how do you find, when you land on a dataset that is newly generated, that isn't curated in any way .... how do I figure out what's in there and how do I figure out who else has worked with it? And if you think about it, this is an amazon.com problem, right?
The reason Jeff Bezos made so much money is that he solved the problem of figuring out what was in books, figuring out by information that was generated by the way other people who've use the book rather than just the way that people had produced the book. What we wanted to do was to build an amazon.com for data, and that's the story I'm going to talk about today, and give you some sense about the platform that we're trying to build.
What am I talking about with the new types of data? Back in the day, data used to be generated by someone filling out a survey form, right? A statistical agency collected it, curated it, documented it, sent it out. Or it could be administrative records, records that are generated from the administration of a government program like tax data. Nowadays, we're also looking at the new types of data, dataset generated by sensors, generated by your card swipes, like retail trade data, Mastercard or IRI data or your DNA. These are complicated datasets. They don't come nicely curated, but they can add a lot of understanding about how to allocate policy.
That data needs to be shared. The challenge that we face is when it's confidential data on human beings, there are, quite rightly, many prohibitions on sharing the knowledge. Everything is much more siloed than in the open data world that you're used to dealing with. For example, as this slide shows, the commissioners in the city of Baltimore will get together every time a child dies to share information about all the government programs that they've touched. It might be housing, education, welfare, foster care and so on. But the only time they share the knowledge is when the kid is dead. What we're trying to do here is to build a knowledge infrastructure that enables us to share the knowledge before children die in ways that can improve policy.
But the challenge is, and this is a risk-utility tradeoff, that the value of working with confidential data is that the more people and the more use it gets, the better off the policy is, but also the greater the risk of disclosure. What you have to do is you have to try and manage that disclosure. If you can, you really can build better policy. New Zealand is a good exampleAs these slides, developed by the former prime minister, Bill English, show. Like most countries or cities, you know, there's three big areas of expenditure: education, health, pensions. And what you want to do, if you want to allocate resources a little bit better, is to use the integrated data generated from multiple government programs a little bit better.
What do I mean by integrated data, for example? Here's a kid, and the age of the kid is along here, down the bottom here is the cost to the taxpayer, not just other things. You look at how this kid hits Children, Youth, Family Services, Abuse, Foster Care, Education, Youth Justice, Income Support or Welfare, right? Kid gets born, by age about two and a half showing up with Children, Youth, Family Services and notifications of abuse. You start seeing the kid here, more abuse, more visits from Family Services. Starts education, by about 9, 10, 11, spotty education, gets taken into care then by 17, he hits into the Youth Justice, and then goes into income support. Pretty predictable if you put that information together. I don't need to belabor the issues that are associated with it here.
That kind of information, if you put it together and understand it, can help allocate resources. This is getting a school certificate, education qualification. If the kid gets it by age 18, now, in pretty good shape for the future. If they don't get it, it's a pretty bad indicator. You can rank, based on the data, you can rank the likelihood of a kid achieving, not achieving this school certificate by age 18, and you can figure out who are the kids who are at the highest risk of not getting the qualification. If you allocate resources away from, you know, kids like my kids, they don't need interventions. They don't need the kind of services that these kids need. You can reallocate funding and you can tremendously reduce the cost to both the taxpayer and transform those children's lives.
It is this type of vision that led to the Evidence-Based Policymaking Act. The big thing is, is how do you put the data together securely, in a clearinghouse? That the kind of work that I'm talking about can be implemented by government analysts and by researchers in a secure way so that the risk of re-identification is minimized.
We built a clearinghouse. which we called the Administrative Data Research Facility. I don't like the term clearinghouse for any number of reasons. It has to be program…mission specific, so I prefer the term facility. But the key thing was not just building the clearinghouse but also building a training program that worked with it.
I'm not going to go into too much detail about it, but the basic idea is you're going to have a secure environment. And then of course, a major challenge is that you have to have telemetry to figure out who's accessing it. But you also need to have metadata around the data. You need to have a rich context because if it's just zeros and ones, I have no clue what it is. And many of you who worked with open data will have observed that the open data can be a bit dodgy in terms of quality. Part of the challenge is the way in which the data was generated, it was just kind of…there's vomit of data that was put together and summarized and doesn't really have any cables going back to the microdata engine. And what you really need is you want the data users to be to be involved in the metadata documentation.
In other words, again, drawing the analogy with the statistical system, the way in which the agencies generate data for use as human beings who create metadata documentation very painstakingly, and you get a report on what all the variables mean, how they were generated and so on. Highly manual process. What we want to do is we wanted to generate an automated way of finding out what information is in the data and what's the quality of that information. Just let me give you a flavor of what those is. In one of the classes we have data on ex-offenders. The programmatic question is, is what's the impact of access to jobs and neighborhood characteristics on the earnings and employment outcomes of ex-offenders, tand their subsequent recidivism of.
Here's where we're in…why this is all on [inaudible 00:14:09], They're going back and forth. Wouldn't it be great to have that tacit knowledge codified so that as people start working with the data, the metadata documentation is generated automatically, like amazon.com. Right? That's the basic idea. You know, instead of you saying, "Where's the data coming from and how was it documented just based on the way it was produced," you've got the community telling you something about what the data is about.
Okay. Here's my challenge. I want to figure out, when I land on a dataset, who else has worked with the data on what topics and for what results. And then I want to generate a community that's going to contribute knowledge. It's kind of an amazon.com for data.
Okay. How am I going to build a machine that's going to do that? Remember the statistical agencies, and I slammed them at the beginning, but, you know, these are great people. These are hardworking, wonderful human beings who have great motivation, but they're like a pre-Industrial Revolution data factory. Now what we're trying to do is we're trying to build a modern data factory, a modern approach to automate the generation of the metadata.
Essentially, what we're going to try and figure out how to do is we are going to scope the question, pose it to the computer science community, natural language processing, machine learning community, and say, "Can you figure out how you can learn from it, automate it, rinse and repeat?" And here's the core insight. We're interested in who has worked with this data before, and identify them and then figure out what they did with it. If you think about it, all of that knowledge is embedded in publications, either published work or working papers or government reports. It's in a document somewhere. In that document, if it's empirical, someone has said, "Here's my question, here's what I'm going to do with it, and here's the section that describes the data."
What I want to do is I want to tee the computer scientists up to help me figure out where is the dataset and where the semantic context that's going to point me to that. Okay. And then I'm going to get the community at large to tell me whether they've done it right or wrong, and then fix it from there.
Essentially, one of the communities we're working with U.S. Department of Agriculture. But anyway, USDA, you'll see one of the things they look at is NHANES, the nutrition education dataset. You'll see here, here they have something that says analytical sample. They say something about the data. What we want is for the computer scientists to go figure out where that data is.
That's what we did. We ran a competition. We took a hand-curated corpus. Social science data repositories, public use ones, sit in different places across the country. One is at University of Michigan, you have ICPSR. There are three people who, every day, their job is to read papers and say which one of the ICPSR datasets is in that corpus, manually. Then they write it down and then they put it up to say what's been done. We took that corpus and we ran a competition. We had 20 teams from around the world compete. Twelve of them submitted code, four very kind people here helped advertise it, thank you for that. Then we had four finalists. The model that actually enable was…that we were amazed was they could actually do this.
Think about it. If I hold up a publication and it… Can you tell me what the dataset is that's referenced in there? And the answer is, of course, no. The baseline is zero. What the winning algorithm did, it correctly identified the dataset that was being cited in the publication 54% of the time. And that's amazing, right?
Now there's a lot of work to be done yet. I've skipped over all the bugs and problems and on, but it's still super encouraging, right? Because now, once I get that dataset to publication link, that gives me the rich context, that gives me the potential to find out everything else because there's a lot of work that's done on publications by my colleagues at Digital Science, UberResearch. They have linked to publications over the past 10 years: grants, policy documents, patents, clinical trials, and so on. Once I get that dyad, I'm off to the races, right? What that enables me to do is to figure out, for a particular publication, everything around it. That was my goal.
Now there's a lot of work that needs to be done around that. But, for example, again, this is a Dimensions website, and I'm not going to have time to go live because I'm going to get yelled at, but I could show you live, you can type in the dataset, and you get lots of related information. You find out who the researchers are, what the related topics are and so on. And that is going to give me the knowledge that I was looking for. It was a pretty buggy model, even though it was amazing. There's, you know, whole amount of work that needs to be done on it.
Now what we want to do is to go and get that dyad clean up. The biggest problem is, and you probably already figured this out, but the search that we had was on titled datasets. It's things that were called American Community Survey or NHANES or PSID or something like that. Where there's a lot of datasets that human beings work with, they'll say, "Oh, we were working with LinkedIn data, we were working with Twitter data," something that's not labeled or with retail IRI, retail scanner data, it doesn't actually have a title that you could go and find. We need much better knowledge from the semantic context. That means we need to develop a corpus, a tagged corpus that the machine learning algorithms can be trained on.
We're working with Dimensions in Digital Science, and we're also trying to get human-curated input in a number of different ways. One is working with publishers where the [inaudible 00:22:01], when an author submits a publication and they say, "Can you give us some keywords," we can tell them, "Give us some…tell us what datasets are in there." Right? If they just tell us what datasets are in there, I've got a dyad right away. Right? And then I can train.
When researchers are getting onboarded into a secure environment and then they are asking about what datasets are available, you could get them to contribute their knowledge as well. In the classes, we run 300 government analysts through who are subject matter experts, we could get them to tell us what they know about datasets that are available for the common good. If any of you are social scientists, please go to this and we're asking our colleagues to just fill it. It turns out, you know, if we get a thousand well curated document with public datasets, that's going to be enough to see the next iteration.
This is where we go next. The Digital Science guys of the guys who brought us Altmetrics. It turns out that people really like…they go to that shiny little badge, the Altmetrics badge, and click on it. What we're designing is if you type in now and look for a dataset, and we're working with the Deutsche Bundesbank as well, it will then pull up the related publications. Then the idea here is every publication that's pulled up, you get the dataset context that's in there and it's going to say how many experts, how many papers, how many code books, how many annotations there are associated with it. Then when you click on that, up pops more rich context, I can find all the papers, the other papers, experts, code books, annotations and related datasets. And then up here is a call for action, right? We don't have to have everyone responding, but we'll trial this out to see how well we do on that. And then of course, feed that into this approach.
Then the last step, and this is work with Brian Granger and Fernando Perez, is to build it into Jupyter notebooks. One of the things that Fernando and Brian have been doing is they're trying to make the workbooks more collaborative. Because right now, it's just a single computational narrative. And they're also trying to work with them, to be able to work with confidential microdata for all the reasons that I talked about.
Here's the basic idea. Currently, now, when you land in on a dataset, if you're lucky, all you get is data that is generated by the way the dataset was produced. The analogy example is Jeff Bezos again. When you look for a book, what do you find? The ISBN number, the author, the title, the publisher, right? That's the metadata, by the way, the book was produced. What you really want is knowledge about the data itself. I don't have to go into a bookstore and find out, I can just find out who else like me has used the information. And again, we're building this into Jupyter notebooks, we, the Jupyter team are, in conjunction with our team, and the notion here is remember the Slack communications that we had, build that in to annotations. Here's Brian and Fernando just putting stuff in, but build that into the annotation that tacit knowledge gets codified and built into the graph model that underlies the data infrastructure.
That's the sweep of the story. We want to be able to build evidence-based policymaking whereby we get better knowledge, get better policy, get resources allocated better, and reduce the cost and the burden of collecting information. We started off with building a secure environment and building workforce capacity around it. We're kind of at this stage right now. Where we want to head is build a platform.
If you want more information, we're hiring here in scenic New York, at NYU. A lot of the information is here and also on our website. You may wonder why it's called the Coleridge Initiative. How many of you have heard of Samuel Taylor Coleridge? Great. Okay. Very famous for "The Rime of the Ancient Mariner," right? We were trying to figure out what to call this thing, and we thought, "Data Science for the Public Good," [blech] "Evidence-Based Policy," [blech]. Coleridge Initiative seemed obvious, right? Rime of the Ancient Mariner, "Water, water everywhere, nor any drop to drink," right? Here, it's "Data, data everywhere, we have to stop and think." That's why it's called the Coleridge Initiative.
This transcript has been edited for readability.
Ann Spencer is the former Head of Content for Domino where she provided a high degree of value, density, and analytical rigor that sparks respectful candid public discourse from multiple perspectives, discourse that’s anchored in the intention of helping accelerate data science work. Previously, she was the data editor at O’Reilly, focusing on data science and data engineering.
Summary
Subscribe to the Domino Newsletter
Receive data science tips and tutorials from leading Data Science leaders, right to your inbox.
By submitting this form you agree to receive communications from Domino related to products and services in accordance with Domino's privacy policy and may opt-out at anytime.