Subject archive for "reproducibility"
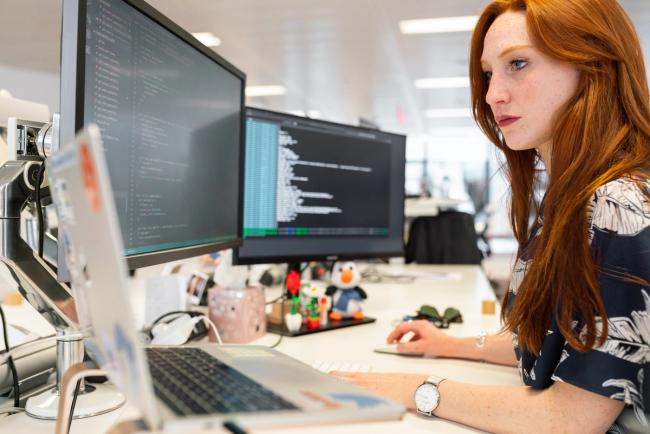
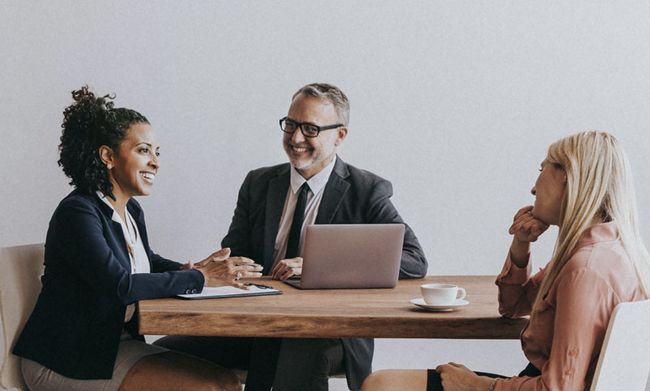
Responsible AI
Navigating the EU AI Act: Strategies for compliance and business growth
By Leila Nouri5 min read
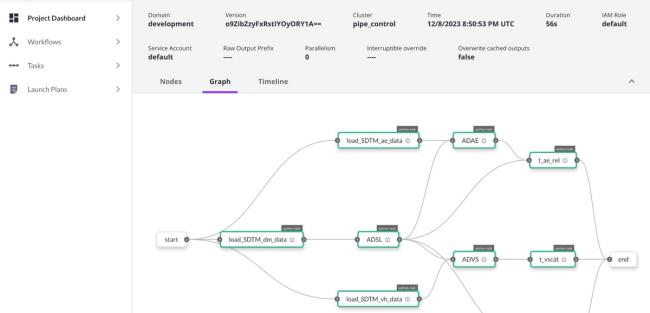
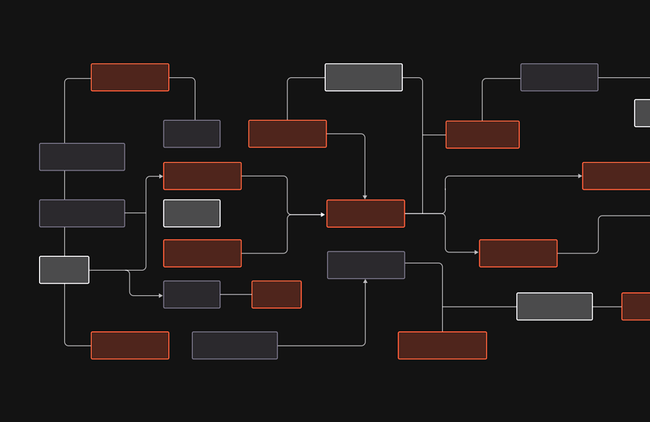
Announcement
Introducing Domino Flows: AI orchestration for life sciences made simple
By Brian Vogl7 min read
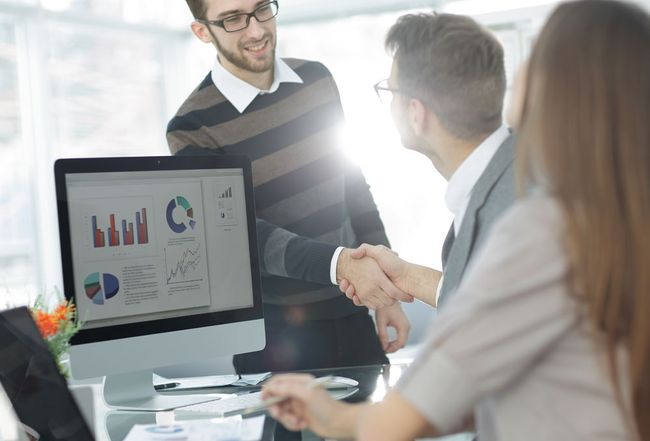
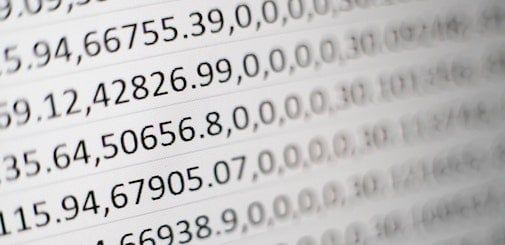
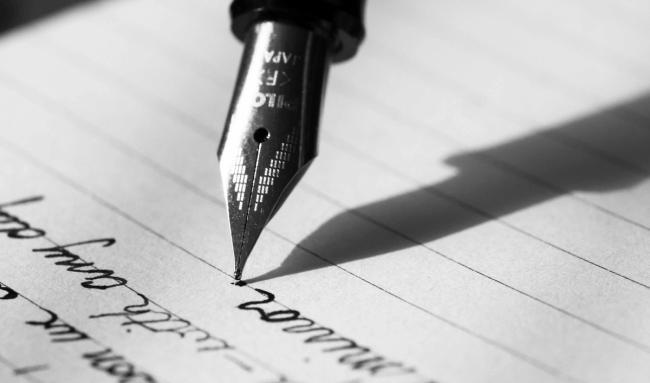
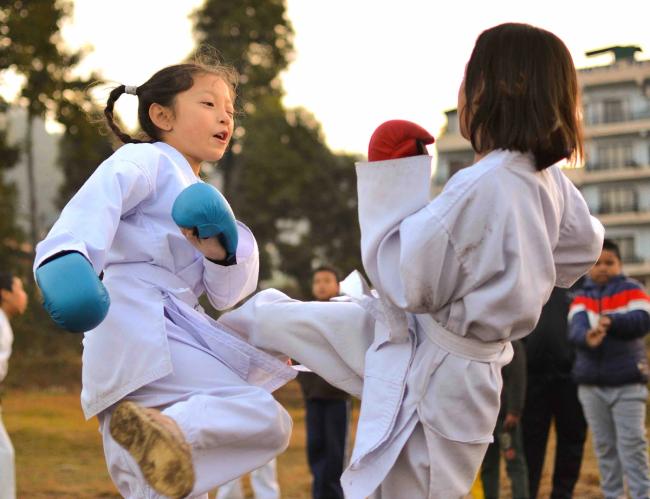