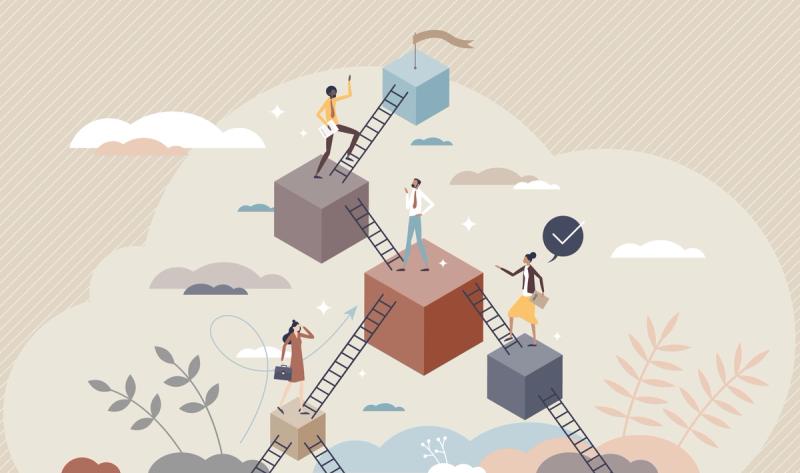
Co-written by Andy Lin, Vice President Strategy & Innovation, CTO at Mark III Systems and David Schulman, Head of Partner Marketing at Domino Data Lab.
The most innovative organizations gaining a competitive advantage with data science are creating a Center of Excellence (CoE) model for data science. McKinsey notes that 60% of top-performing companies in advanced analytics follow a CoE approach. Bayer Crop Science, for example, attributes many advantages to a CoE approach, such as greater knowledge sharing across teams, increased efficiency in data science initiatives, better alignment of data science and business strategy, and improved talent acquisition.
What is the path to building a thriving AI Center of Excellence (CoE)?
At Mark III, we’ve had the honor and privilege over the last few years to work in with NVIDIA and dozens of organizations in support of their journey to an AI CoE. At NVIDIA GTC, September 19-22, we announced new AI CoE solutions using the latest technologies from NVIDIA and Domino Data Lab.
Although every story and path is slightly different, we’ve noticed that most of them follow the same five general steps or phases as they mature. Domino Data Lab’s Enterprise MLOps platform integrates across NVIDIA’s product line of infrastructure like NVIDIA DGX systems, NVIDIA-Certified Systems from leading OEMs, and the NVIDIA AI Enterprise software suite. We have also helped organizations like Allstate, SCOR, and Topdanmark scale their CoEs. Although every story and path is slightly different, we’ve noticed that most of them follow the same five general steps or phases as they mature.
But before we describe what these steps we’ve observed are, we should probably define what an AI Center of Excellence is (at least our perspective of the AI CoE).
What is an AI Center of Excellence?
Quite simply, an AI Center of Excellence is a centralized compute resource for data science and AI/ML that's managed by one team at one point, but that can serve one, five, ten, a hundred, or even thousands of data scientists, each of whom has their own unique needs around IDEs, frameworks, toolsets, libraries, models, apps served, teams that they work within, and can all “vote with their feet” on where they build and run their models.
In short, even if you’re successful in building the technology stack to serve your data science community, there is a good chance that if you don’t maintain laser focus on the team, culture, human, user experience and education sides of the equation in parallel, they won’t like it and they’ll move to something else (or even refuse to use it), and your AI CoE won’t be successful.
So accordingly, the journey to the AI Center of Excellence is truly half technology, half non-technology (education/culture/teams). You’ll find this idea featured prominently below.
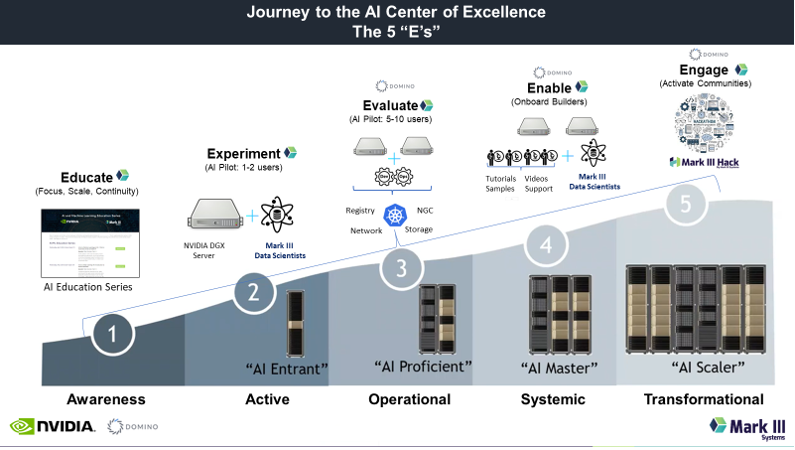
What are the five steps to building an AI CoE (as observed in working with dozens of institutions and organizations) and how do we work together to ensure that it is successful?
Educate
Even before there’s any discussion about technology and certainly any thoughts about a centralized platform or MLOps, we’ve found that the most successful organizations are already educating their data science community in preparation for a centralized AI CoE technology stack someday. At any given point in time, we’ve noticed that at least 70% of attendees are either starting out or trying to reinforce basic AI/ML fundamentals, so continuing practical education is always important both in the beginning and on an ongoing basis.
Mark III has helped to fill this gap by educating communities within organizations at an early stage and offering continuing education throughout all phases via its AI Education Series, a set of live, virtual tutorials/labs designed for diverse, geo-dispersed audiences and designed specifically with the idea of practical AI Education in mind. The focus is entirely on terms, practical concepts, and the labs — and can be immediately “taken home” by attendees for them to plug in their own numbers, data, and use cases and start iterating immediately.
Domino has seen similar examples from organizations, not just for data scientists but for business leaders as well. Allstate uses education programs focused on educating business leaders about data science, facilitating cross-functional communication by equipping business leaders and data scientists with “a common language” to solve difficult business problems (learn more at 43:00 in this on-demand panel).
Other examples of education we’ve seen foster this culture and “set the table” for the AI CoE include interactive user-driven workshops, cross-functional team building, and “flash builds” around shared challenges.
Experiment
The next phase that we generally see, and this often overlaps with the "Educate" phase (which really is always ongoing once it starts), is the "Experiment" phase, where data scientists and “builders” emerge from the shadows and tell you what they’re working on and how they’re using or thinking about using data science and AI models to do game-changing things all around the organization.
Domino has seen organizations carve out intentional time for data scientist experimentation and innovation — potentially outside of “top-down” business objectives. Allstate allocates a portion of data scientist time to work on any project of interest, fostering cross-team experimentation and collaboration to drive innovation. For additional details, please view this on-demand panel).
The incredible thing about the open-source nature and history of AI over the last 5-10 years is that literally anyone can get started with just their laptop or workstation, inside or outside of the office. An official effort to formally educate the community around data science is almost like a green light for these builders to come out and show off what they’ve been working on. At this point, organizations have an opportunity to encourage the community to continue and accelerate their work in their respective groups by giving them access to better platforms and tooling within the workflows that they’re used to.
Evaluate
With enough education and encouragement, there comes a point where it becomes difficult for an organization to keep track of all the models being built and all the data science activity happening across all its siloes and groups. Conversely, data scientists start running into real challenges, such as model drift, inability to track dataset versions, inability to obtain optimal datasets that they need for their models, sluggish velocity at taking a model from training to inferencing in production, and much more. The good thing is that there is a lot of activity and buzz across the organization, but these are real problems that can derail all momentum, if they aren’t addressed.
This inflection point is the perfect time to "Evaluate" and look at an MLOps platform, which when done right, can serve as the technology core of the AI Center of Excellence moving forward and allow the organization to manage the CoE at one point, without infringing on the unique needs and preferences of each individual data scientist as the organization scales.
Openness and flexibility is key to data science innovation, which is why it’s a core pillar of Domino’s platform. The landscape of data science tools and technologies is heterogeneous and constantly evolving, and the platform at the core of the CoE must provide flexibility, agility, and scalability. Bayer saw this as a key factor in evaluating Domino, with data scientists using many tools such as RStudio, Jupyter, Flask, etc. Naveen Signla, Bayer’s Data Science Center of Excellence Lead, notes, ““Domino has made it easier for users across the global enterprise, using different tools and with varied backgrounds and skill sets, to work with each other, leverage past work, and collaborate quickly.”
The word we use for this phase is "Evaluate" because it requires not only the ability to pilot and “roll” the MLOps technology stack, powered by Domino’s Enterprise MLOps platform with a Kubernetes foundation, but also ensure that data scientists in any pilot have a terrific experience training, deploying, and running their models. In short, it is a true evaluation. If your early-adopter data science users don’t have a great experience, your AI CoE may not make it to the next phase.
Enable
Should the organization be successful, the fourth step in the journey to the AI Center of Excellence is the "Enable" phase, which centers around rolling a successful pilot for the AI CoE into early production, ensuring that data science users get onboarded in greater numbers and working with the IT operations team to make sure that the environment stays up and running.
Mark III has found that treating and supporting the AI CoE almost like a software-as-a-service product — and treating the team managing it as a product management team — is an effective lens to build out a team and strategy. Domino has seen similar approaches focused on enabling both data scientists and the business, with S&P Global using Domino as the “glue” underpinning a program to educate 17,000 globally distributed employees on data science. SCOR embeds data scientists within the business to better foster ideas, practices, technology, and documentation to drive data science adoption. Additionally, their data science experts use Domino as a tool to share best practices and templates.
Mark III has assisted organizations with everything from building knowledge bases and tutorials for onboarding to “co-piloting” with IT operations teams to keep the overall AI CoE environment up and optimal.
Engage
The fifth and final step in the journey to the AI Center of Excellence is the "Engage" phase, which is all about activating the remainder of the data science community to onboard the AI CoE. If you’re thinking in terms of the technology adoption curve, this would include early majority and late majority users.
By this time, the organization’s AI CoE and MLOps platform are humming, most groups are fully aware, and the onboarding and user experience should be running smoothly, even for non-expert users.
At this point, the AI CoE should be running like a well-oiled machine. The focus is driving up engagement for the masses, to encourage them to do more with and lean into the AI CoE. At Mark III, we often co-run hackathons at this "Engage" phase to not only drive awareness throughout the organizations, but also to help build partnerships with external organizations, both in industry and the public sector.
Similarly, Domino is often the MLOps platform used in hackathons to provide the infrastructure, data, and tooling required by participants. Johnson & Johnson held an internal hackathon to improve forecasting in its vision care business, using Domino’s platform to engage dozens of teams to come up with the best model. BNP Paribas Cardif held a Virtual Data Science Hackathon with more than 100 data scientists from universities and partner companies, using Domino Data Lab to enable each team to access the infrastructure resources they needed.
How to Build an AI CoE: New Solutions Announced at NVIDIA GTC
The journey to the AI Center of Excellence is different for every organization, but we think that if these five steps are planned for and followed, it will likely lead to success, no matter the exact path. Like mentioned above, this journey is based on half technology, half-culture/team/education and is something our collective teams can assist with via our shared learnings.
Enterprise MLOps is critical on the journey to the AI CoE, as it forms the technology core of the CoE, but please don’t neglect the other key steps and pieces on the road there.
After all, AI Center of Excellence success really is all about the journey, not the destination. Learn more about our AI CoE solutions, announced at NVIDIA's GTC September 19-22.
Andy is Vice President of Strategy & Innovation, CTA at Mark III Systems. He is leading multi-year strategic initiatives, architecting Mark III's "Enterprise Full Stack Partner" solutions strategy across all functional areas. He joined Mark III Systems in 2009 and has served in various roles as a Vice President, Sales Executive, Solutions Architect, and Marketing Strategist in Mark III’s Austin office.
RELATED TAGS
Subscribe to the Domino Newsletter
Receive data science tips and tutorials from leading Data Science leaders, right to your inbox.
By submitting this form you agree to receive communications from Domino related to products and services in accordance with Domino's privacy policy and may opt-out at anytime.