Should You Build Your Own Data Science Platform?
Desmond Chan2018-07-10 | 4 min read
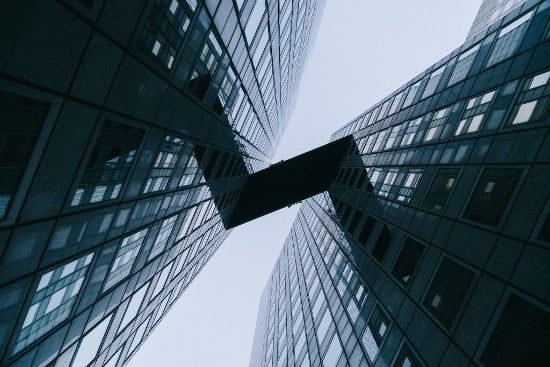
As organizations increasingly strive to become model-driven, they recognize the necessity of a data science platform. According to a recent survey report, “Key Factors on the Journey to Become Model-Driven”, 86% of model-driven companies differentiate themselves by using a data science platform. And yet the question of whether to build or buy still remains.
For most organizations, purchasing a data science platform is the right choice from both a business strategy and project cost efficiency perspective. However, many organizations confuse the criticality of models to their long-term success with the need to build the underlying platform themselves. In a few select situations, the platform itself is the differentiator. These organizations have highly specialized workflows (e.g., Uber), a stellar track record of internal software development (e.g., Airbnb), and deep data science expertise that recognizes the unique traits of models. For the vast majority of organizations, the competitive differentiator is not the platform, but the entire organizational capability — what we call Model Management — encompassing many different technologies, stakeholders, and business processes. Buying the platform is the logical choice for most.
You’re probably thinking, “Of course Domino, the data science platform vendor, believes everyone should buy a data science platform.” We at Domino do admittedly have our opinion on the topic, but this opinion stems from thousands of interactions with organizations of all shapes and sizes around the world that have faced common struggles and obstacles on their journey to become model-driven. Most that have opted to build one on their own have stalled or failed. Those who have purchased a platform are operationalizing data science at scale. These interactions and experiences working with organizations trying to decide whether they should build or buy led us to develop a rigorous and objective framework to facilitate the decision process. In this framework, we examine three major factors:
- Total cost of ownership The scope of building, managing and operating a data science platform needs to be carefully examined. Many organizations underestimate the total cost of ownership in the build approach. In a four-year scenario where an organization builds a data science platform supporting 30 data scientists at first (and growing at 20% annual rate in subsequent years), we estimated the TCO of building to be over $30 million while the TCO of buying is only a fraction of that. See Figure 1 below for a yearly side-by-side comparison of the TCOs of the two approaches.
Figure 1. Four-year projection of TCO of build vs. buy
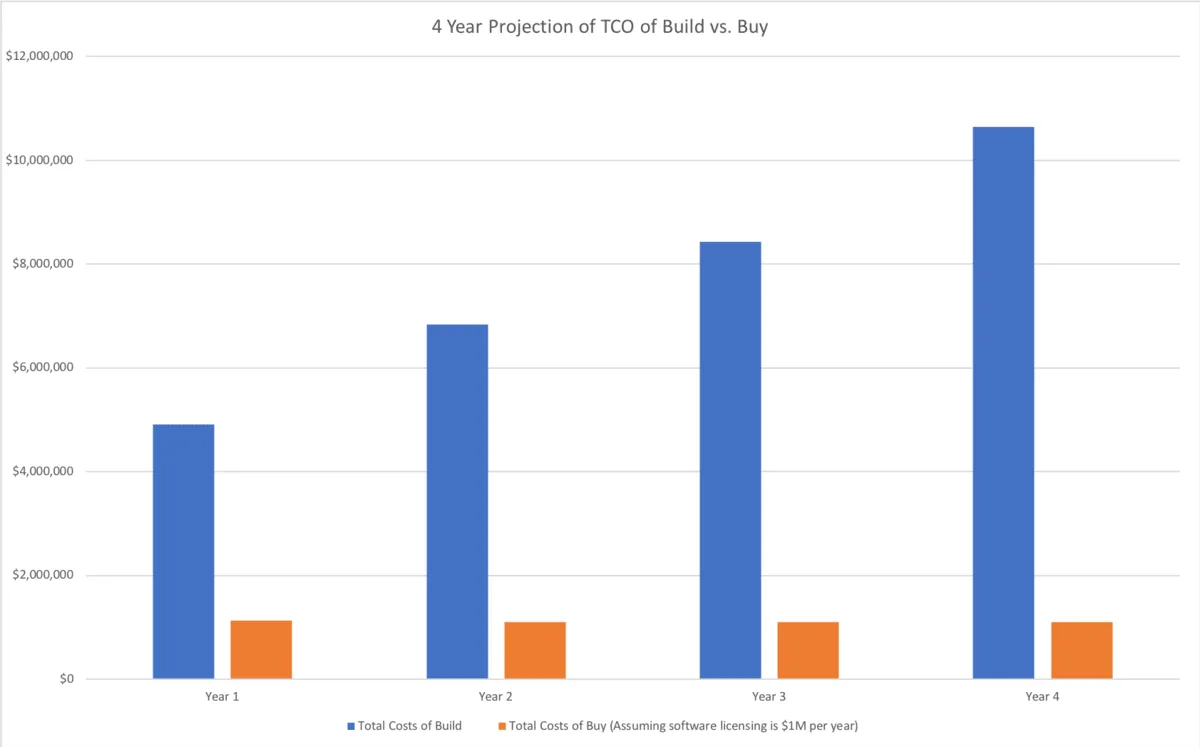
- Opportunity costs By devoting resources to building a data science platform, an organization is inevitably choosing to divest from other projects. This choice can be unwise especially if the organization sacrifices its core competency, which will eventually hurt the organization’s revenue.
- Risk factors Data science is not an easy endeavor to take on, and it is wise to de-risk as much as possible. Risk factors such as talent acquisition and retention, skill requirement changes, and platform feature requirement changes need to be considered carefully before deciding to build. On the flip side, an organization should also be very careful with choosing which vendor to purchase from if they so decide.
If you are inclined to dive into more details of this framework, you can peruse the Domino whitepaper: “Should You Build Your Own Data Science Platform?”. Ultimately, organizations need to decide where their differentiation lies with data science: in the models they build and overall organizational capability, or in the underlying infrastructure? For most, it is the former, so a “buy” approach likely offers the lowest TCO and most aligned strategic choice.
RELATED TAGS