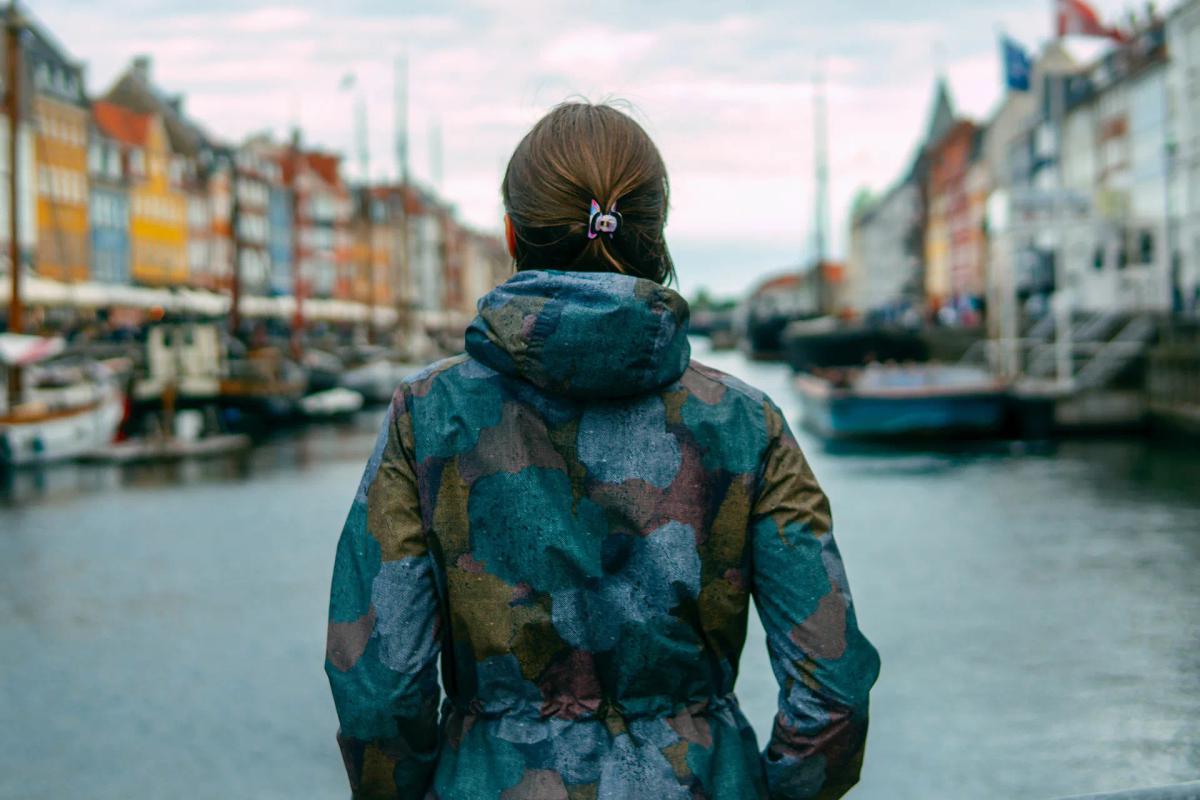
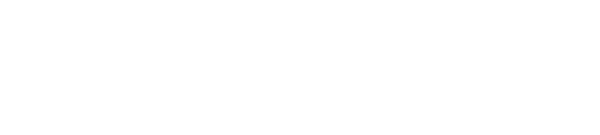
Giving Homeowners Answers About Insurance Coverage in Seconds with Model-driven Policy Approvals
Data Science at Topdanmark
Topdanmark, a large European insurance company, is infusing data science across its operations to provide consumers with a better, faster insurance experience. The company's model-driven workflows, built and deployed on the Domino data science platform, have automated 65 percent of cases for approving new coverage and processing claims. Customers can now learn whether they have coverage in one to two seconds versus four days, and Topdanmark experts can focus their effort where their expertise is most needed. What's more, if model drift occurs once a model is in production, the Domino platform can immediately notify Topdanmark data scientists so they can take proactive action and ensure the business isn't negatively impacted.
Challenge
Several years ago, executives at Topdanmark set out to dramatically change how quickly they could respond to customers—whether the decision entails accepting risk to insure a client's home or processing a claim. While the company has always worked to make policy decisions and process claims quickly, traditional decision-making processes were time-consuming and laborious. Staff had to manually pore through pages and pages of reports on a building's condition and make educated guesses as to whether the building might have hidden damage that assessors couldn't see. As a result, it could take nearly a week for customers to learn whether their claim was covered.
"We saw an opportunity to use machine learning to automate or augment decision making in real time," said Stig Pedersen, the company's head of Machine Learning.
Solution
Initially, the data science team believed they could automate between 30 and 50 percent of the company’s new policies with machine learning. The company has a market share in Denmark just below 50 percent in this product line. The team surpassed its goal using the Domino data science platform to develop and deploy model-driven workflows faster. The company now also uses model monitoring in Domino to get insights into how models are performing in real-time, and to detect data and model drift once models are in production.
The model is 800 times faster than humans, so customers can learn whether they're covered in one to two seconds.
With Domino, data scientists can:
- Easily test new ideas and share their work using natural language and image processing techniques. Through their collaboration, the team has been able to stand up minimum viable product models in just 12 weeks that can predict on the fly whether a claim is valid or requires expert investigation. The final automation level was reached during the first year in production by retraining and adjusting the models. The work has enabled the company to automate 65 percent of all cases so it can effectively balance its need for speed with human expertise and judgment.
- Expedite deployment of new models using APIs. For example, inspection reports saved to the company's data lake can easily be anonymized and retrieved via API by a machine learning framework on Domino that assesses the level of risk based on both the reports and model predictions of potential hidden risks. From there, the model determines whether to approve the policy outright, approve with certain conditions, deny coverage, or, if an unusual pattern in the data emerges, send for manual investigation by an investigator.
- Detect data and model drift in real time. "Data drift can have a critical impact on predictions and ultimately, our business," said Pedersen. "Model monitoring in Domino saves us significant time previously spent on maintenance and investigation, and enables us to monitor model performance in real-time and compare it to our expectations."
The Domino Effect
Through its model-driven workflows built on Domino, Topdanmark has dramatically improved the customer experience, while reducing costs. This includes:
- Reducing approval times from four days to one to two seconds. "The model is 800 times faster than humans, so customers can learn whether they're covered in one to two seconds," said Pedersen. "Even customers whose applications aren't approved are happy because they receive an answer right away rather than having to wait days."
- Innovating faster with 65 percent of cases automated. "When we initially started, data scientists estimated that we could reach between 30 percent and 50 percent automation," said Pedersen. "We've reached 65 percent. Even our data scientists were surprised how fast and far we could push this automation level."
- Rapidly uncovering model drift to minimize the potential business impact of suboptimal predictions. In one case, Domino was able to automatically detect drift that had previously taken three months to identify manually.
Industry
Insurance
Location
Headquarters: Ballerup, Denmark
Use Cases
Fraud detection
Policy approvals
Risk management
Impact
Policy approvals completed in 1-2 seconds versus 4 days
65% automation of all cases (up from an initial target of 30%-50%)
Model drift detected in real time as opposed to 3 months with manual monitoring methods
Solution Components
Data Science Platform: Domino
Cloud infrastructure: Amazon Web Services
Data Science Tools: PyTorch, TensorFlow, Keras, Spark, Python, Pycharm

Resources
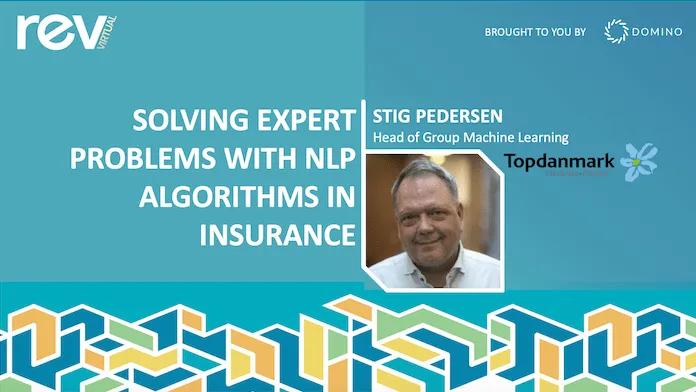
Related Event
Solving Expert Problems with NLP in Insurance
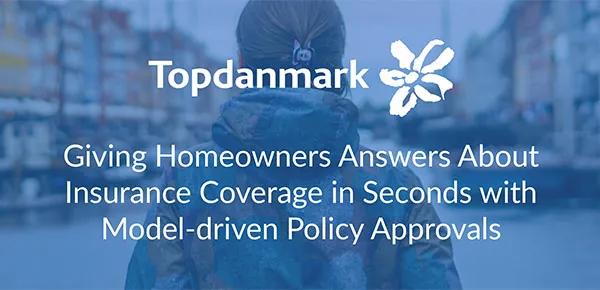
Case Study | PDF