50x Faster Performance with Ray and NVIDIA GPU-Accelerated Compute
ON DEMAND | Originally Aired March 8, 2022
Watch On Demand
Harness NVIDIA GPU-Accelerated Parallelization with Ray
Apache Spark has been the incumbent distributed compute framework for the past 10+ years. But the overhead and complexity of Spark has led the longtime leader to become eclipsed by new frameworks like Ray.
In this technical talk, we will provide an introductory overview of Ray, its origin, strengths, weaknesses, and best practices for using it. You'll learn reasons why you should choose Ray based on available compute infrastructure, data volumes, workload complexity, and more.
We'll show Ray in action, demonstrating performance gains from NVIDIA GPU-acceleration.
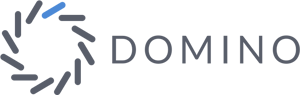
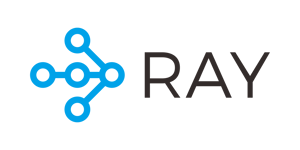
What's in store for you
The what and why of Ray
Discover Ray's history and learn its intended use cases in data science work
Getting started with Ray & MLOps
Learn strategies to start taking advantage of Ray's benefits quickly and easily using MLOps
See Ray in action for ML model tuning
See a demo of an NVIDIA GPU-accelerated hyperparameter optimization workflow
Meet the speaker
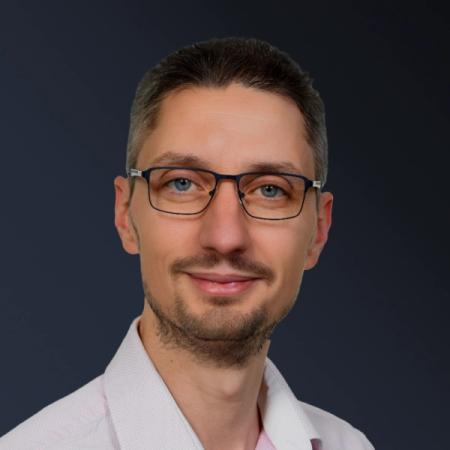
Nikolay Manchev is the Principal Data Scientist for EMEA at Domino Data Lab.
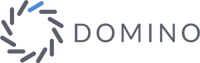
In this role, Nikolay helps clients from a wide range of industries tackle challenging machine learning use-cases and successfully integrate predictive analytics in their domain-specific workflows. He holds an MSc in Software Technologies, an MSc in Data Science, and is currently undertaking postgraduate research at King's College London. His area of expertise is Machine Learning and Data Science, and his research interests are in neural networks and computational neurobiology.