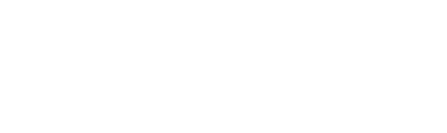
Applying leading-edge data science to push the bounds of rocket science
Watch on demand
Data science at Lockheed Martin
Lockheed Martin is at the forefront of innovation, applying sophisticated research methods to protect our world and explore new frontiers. Its missions are some of the most important and challenging in the world—from protecting citizens to advancing the boundaries of science. Its client list includes the US Department of Defense, US Department of Homeland Security, the National Aeronautics and Space Administration (NASA), and the US Defense Advanced Research Projects Agency (DARPA), among others.
The company uses the Domino enterprise data science platform and NVIDIA technologies to streamline development and deployment of advanced machine learning capabilities. These include an IIOT system that identifies potential equipment issues on the manufacturing floor, a predictive risk management system that helps supply chain professionals anticipate and take action on potential supplier issues to avoid part delays, and a AI fighter system that captured second place in DARPA’s AlphaDogFight competition, which pits AI technology against humans in aerial combat.
“We see Domino and NVIDIA as key components to our broader MLOps [Machine learning operations] pipeline, driving 10-100x efficiency gains for our data scientists and enabling Lockheed Martin to provide differentiating solutions across a broad set of challenges,” said Mike Johnson.
Webinar summary
Lockheed has committed to a digital transformation that makes it possible to both deliver cutting edge innovations faster, and to improve everyday efficiencies like optimizing the supply chain.
But, as Mike Johnson and Greg Forrest shared, bringing AI to scale is a huge task with:
- 110,000 employees worldwide operating in 54 countries (around 50 percent of employees work as scientists and engineers)
- 375-plus facilities
- Four divisions that deliver advanced solutions spanning land, air, sea, and space, and include everything from the F35 aircraft to space shuttles
During their talk, they shared how Domino and NVIDIA are helping them create an MLOps framework to bring development, security, and operations best practices to machine learning and achieve a 10 to 100X efficiency gain for data scientists.
Here are three examples they shared:
- Reducing supply chain risks. Lockheed Martin is in the process of building the Orion crew module for human spaceflight that is targeted to go to the moon in 2024. A project of this type requires many different unique components and there's significant risk if a part can not be supplied on time. To reduce risk of delays, data scientists are building models with Domino and NVIDIA that analyze open source unstructured data, such as information about a merger between two suppliers; classify and assess potential risks; and provide insight on what might happen next (for example, if a company may experience financial losses). These insights are combined with extensive internal knowledge of the company’s supply chain to give supply chain professionals a better sense of any risks so they can respond to them. With Domino and NVIDIA, the company has been able to train these models with effectively no training data—something that required a large amount of compute power--and rapidly deploy interfaces so they could create an active learning loop to obtain feedback from individuals on how the models are performing.
“The ability to kind of keep track of that data and assign it to these projects really made this project a success.”
—Mike Johnson, Lead Data Scientist, Lockheed Martin
- Building trust in AI systems through its participation in the AlphaDogFight competition, hosted by DARPA. Participants were tasked to develop an AI fighter system that could compete with humans in a Top Gun style aircraft fight. Lockheed was one of many teams, and it secured second place by taking a reinforcement learning approach and using Domino and NVIDIA to spin up multiple training jobs (each of which took on the order of 30 days) and track experiments.
“Before we had Domino, it took eight weeks to get access to a GPU. Now it's just a push button away. Those savings add up across thousands of employees at scale, and that really makes a difference.”
—Greg Forrest, Senior Manager of AI and AiMLabs, Lockheed Martin
- Predicting equipment failures before they affect operations. In his final example, Mike Johnson shared what it takes to build an F35 aircraft and the number of different operations that have to happen (all in one mile-long building) to turn raw materials into an aircraft. To minimize equipment downtime, the company is prototyping an AI-driven system that can continuously monitor every machine for potential issues and can notify staff when machines aren’t operating properly. So far, using Domino and NVIDIA, around three machine learning engineers were able to develop and train over 250 models and deploy 150 models at the same time.
About the speakers
Mike Johnson is the technical lead for a team of data scientists, machine learning engineers, and data engineers to deliver AI solutions across Lockheed Martin. He has built machine learning solutions in numerous fields including manufacturing optimization, semiconductor reliability, human resources, radar signal analysis, and time series search. While he has an undying love of natural language processing, he has recently been focused on applying deep learning at scale to the domain of unsupervised anomaly detection.
Greg Forrest is responsible for leading the development of Lockheed Martin’s corporate artificial intelligence strategy, creating an enterprise AI ecosystem and MLOps pipeline, and leading teams that develop transformative AI and machine learning capabilities for Lockheed Martin and its customers.