How the US Navy is improving underwater mine detection with MLOps
Watch now
Accelerating ML model updates by 97% with best-in-breed AI infrastructure
The U.S. Navy uses machine learning (ML) models for underwater target threat detection by unmanned underwater vehicles (UUV). However, without a way to monitor and improve ML model performance at scale, models were slow to adapt to changing underwater conditions or enemy tactics — unable to “learn” based on recent field activity. The Navy partnered with the Defense Innovation Unit (DIU) as part of Project Automatic Target Recognition using Machine Learning Operations (MLOps) for Maritime Operations (AMMO).
The AMMO MLOps pipeline decreased the time needed for ML model updates from six months to a few days, enabling the Navy to quickly retrain and deploy automatic target recognition (ATR) models at the speed of operational relevance. Domino Data Lab’s modular, open, and extensible AI platform serves as the factory for integrating commercial technologies from Weights and Biases and Fiddler.ai — providing the governance and flexibility for distributed teams to deploy models faster with built-in observability and reduced time-to-impact.
Watch this on-demand webinar to learn how the US Navy and DIU:
- Improved AI-based target recognition reliant on underwater sensor data so threat intelligence is more trustworthy for decision-making.
- Accelerated time to train and deploy models against an expanded threat environment.
- Leveraged modern MLOPs practices with best-in-breed solutions to operationalize AI at scale in the cloud.
- Securely collaborates with multiple contractors and agencies.
Register now to see how your agency can benefit from these best-in-breed tools.
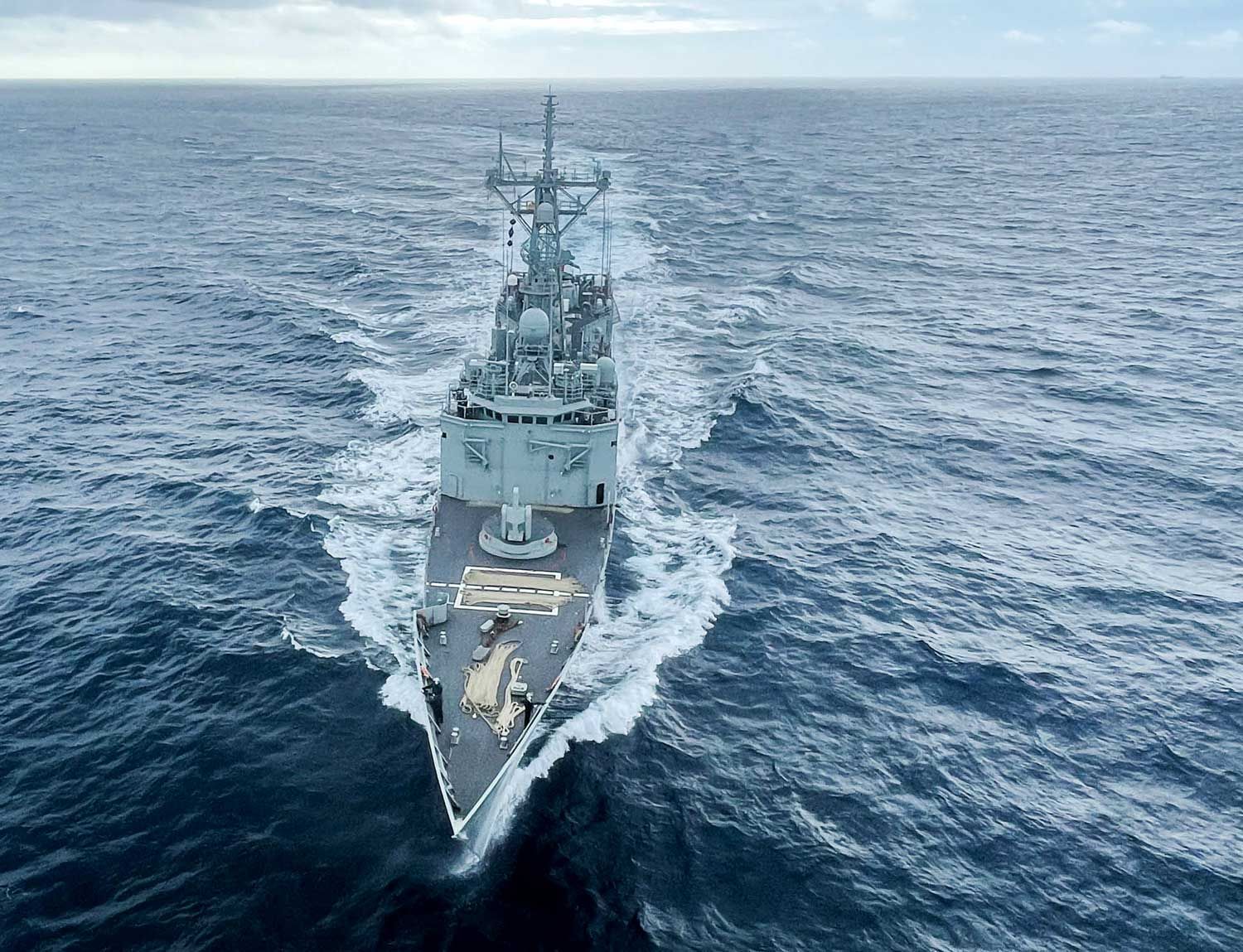
Featured speakers
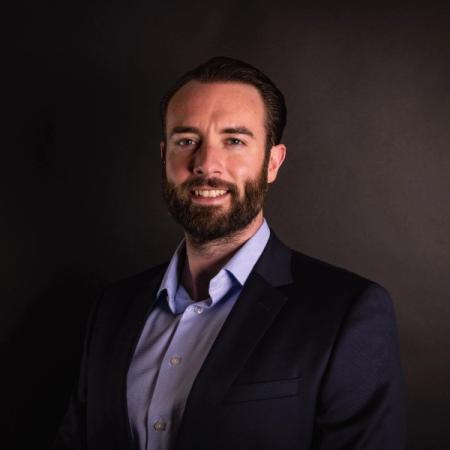
Christopher Elsins
Director of Public Sector GTM, Domino

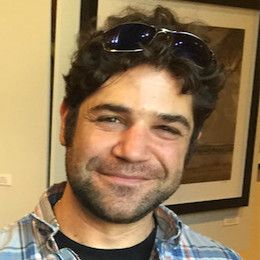
Josh Rubin
Principal AI Scientist, Fiddler.ai
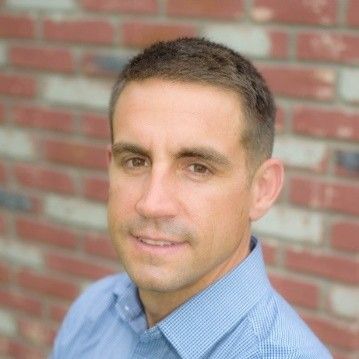
Kevin Stofan
Solutions Engineer, Weights & Biases
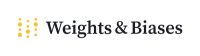