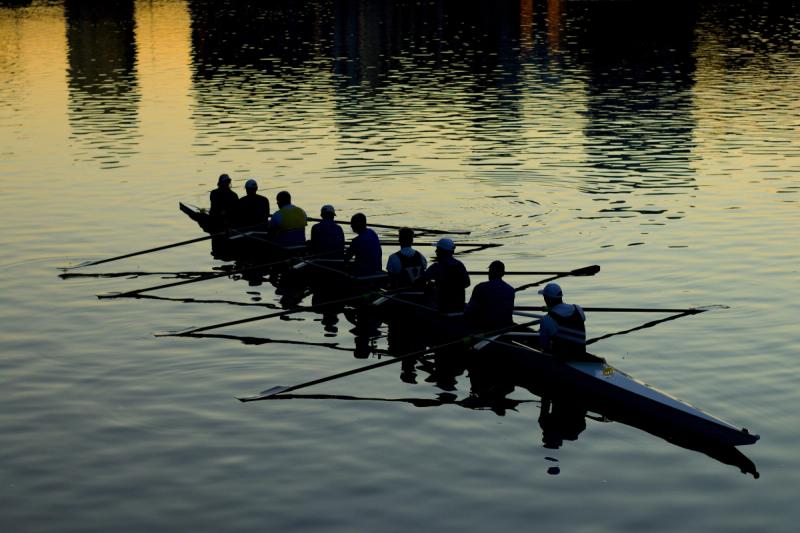
Most data science leaders can likely recall an instance where collaboration among a few data scientists ignited a new idea, accelerated the on-boarding of new team members, or helped speed up the development or deployment of new models.
They can also likely point to instances where lack of collaboration hurt their team’s productivity and progress with data scientists recreating code, experiments, and processes that others have already created.
It’s led some data science leaders to begin thinking programmatically about collaboration. This was one of several topics that data science leaders Matt Cornett (from a leading provider of insurance solutions), Patrick Harrison (from a global financial intelligence company), and Brian Loyal (from Bayer Crop Science) discussed in their webinar: Best Practices for Driving Outcomes with Data Science.
During their talk, they shared some best practices for enhancing collaborations among data scientists. These include:
- Developing a data science support network that facilitates collaboration among data scientists across business and software teams. Connecting data scientists from across these teams, Patrick Harrison explained, enables them to gain the support and mentorship they need, share tips and tricks, discuss common challenges, and advocate for shared interests. “Working with common tools and platforms really helps with this, and so if you are able to, even in a federated or hub and spoke model, to standardize on common ways to get things done and common tools to use that can really smooth out the path to an effective data science support network,” he said.
- Building with reusability in mind. At Bayer, data science leaders ask teams to start projects with reuse in mind. “We’ve seen a number of times how quickly a successful data science project can find applications in unexpected places,” said Brian Loyal. “By setting and reinforcing best practices early on, you can make life a lot easier for potential collaborators. And standardized tools like Domino can really help with baking in this reusability from the start.”
- Conducting regular peer reviews. One activity Matt Cornett likes to do with his team is to encourage data scientists to share work that’s in flight. “This is going to foster greater creativity and really help eliminate siloed efforts that can easily go off track,” he said. “The more that you’re able to share that work as it’s going, the more feedback you can get, and I think the stronger the end result is.” He facilitates this by scheduling time for these reviews in his weekly staff meeting—assigning one data scientist each week to talk through their project and get feedback from the rest of the team. Here too, Cornett says Domino has been a huge help. “There are so many different collaboration features within Domino,” he explained. “If you’re just running RStudio or Jupyter Notebook on your computer, if you want someone else to run your code, their computer has to be configured pretty much the same as yours. Domino helps to resolve that so people can jump into other people’s code and be able to interact with it as they’re collaborating, and as they’re thinking ‘what do I need to do next?’”
- Assigning multiple data scientists to a project. “One of the things that I’ve found in my last couple of roles is any time you have more than one data scientist working on a project, your results are really kind of multiplied,” Matt Cornett said. “It’s not necessarily a linear path. I think it’s an exponential kind of growth in the insights that you’re finding, in what you’re being able to put out and what you’re being able to develop.”
Listen to Matt Cornett, Patrick Harrison, and Brian Loyal discuss fostering great collaboration among data scientists.
Listen to the full discussion to hear more from Matt, Patrick, and Brian on best practices for increasing collaboration and driving outcomes. As these leaders show, collaboration at scale doesn’t just happen. Regardless of the type of organizational model in place—centralized, distributed, or using a hub-and-spoke model—data science leaders need to institute practices in the daily cadence of model development that foster sharing ideas and knowledge to innovate successfully.
Learn more
Watch the webinar, “Best Practices for Driving Outcomes with Best Science,” featuring data science leaders Matt Cornett, Patrick Harrison, and Brian Loyal.
Read the report, “Organizing Enterprise Data Science,” to learn more about the best practices data science leaders use to build an enterprise data science strategy.
Take our Model Velocity Assessment to determine where your organization is on the maturity path.
Domino powers model-driven businesses with its leading Enterprise MLOps platform that accelerates the development and deployment of data science work while increasing collaboration and governance. More than 20 percent of the Fortune 100 count on Domino to help scale data science, turning it into a competitive advantage. Founded in 2013, Domino is backed by Sequoia Capital and other leading investors.
RELATED TAGS
Subscribe to the Domino Newsletter
Receive data science tips and tutorials from leading Data Science leaders, right to your inbox.
By submitting this form you agree to receive communications from Domino related to products and services in accordance with Domino's privacy policy and may opt-out at anytime.