Introducing the Data Science Maturity Model
Mac Steele2016-11-22 | 2 min read
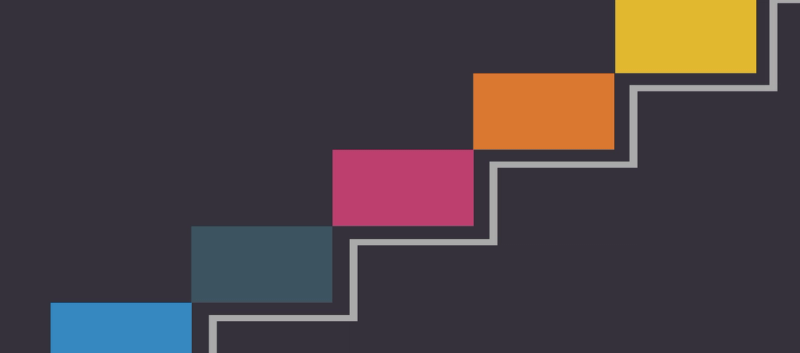
Many organizations have been underwhelmed by the return on their investment in data science. This is due to a narrow focus on tools, rather than a broader consideration of how data science teams work and how they fit within the larger organization. To help data science practitioners and leaders identify their existing gaps and direct future investment, Domino has developed a framework called the Data Science Maturity Model (DSMM).
The DSMM assesses how reliably and sustainably a data science team can deliver value for their organization. The model consists of four levels of maturity and is split along five dimensions that apply to all analytical organizations. By design, the model is not specific to any given industry — it applies as much to data science in insurance as it does to data science in manufacturing. The matrix below shows how we map the DSMM.
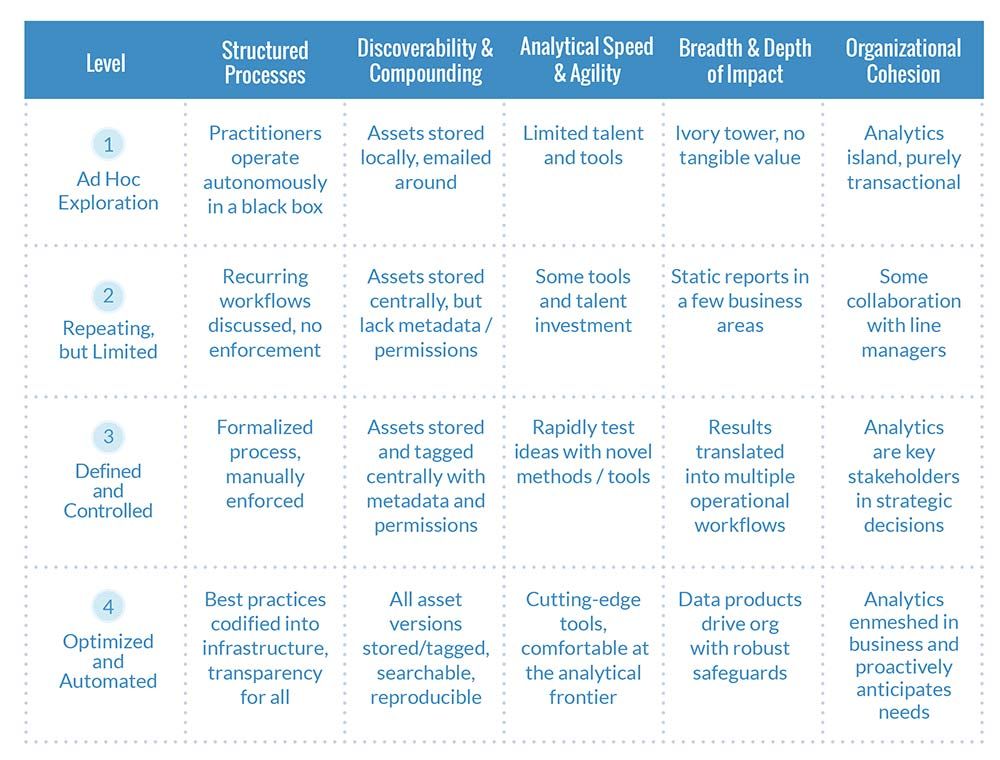
Alongside describing the maturity framework, the paper presents case study examples of teams at each of these four levels of maturity and offers actionable advice for improving the function of data science teams.
It is important to remember there is no turn-key solution to becoming a mature data science organization. Anyone pitching such a solution is naive or misleading. The teams we’ve observed operating at the highest level had visionary champions and took years of disciplined investment across people, process, and technology. Moreover, as the field evolves, it’s not enough to rest on one’s laurels.
Technology has reduced costs and barriers to entry in data science, eliminating a moat relied on by visionary firms. In the future, we believe the competitive edge in data science will derive from process and structure, more so than tools alone. Practitioners and executives alike should consider if their current strategy is building towards a mature organization.
If you have feedback or suggestions on how we can improve the framework, do not hesitate to contact us. We hope to iterate on this first draft and incorporate insight from the data science community.