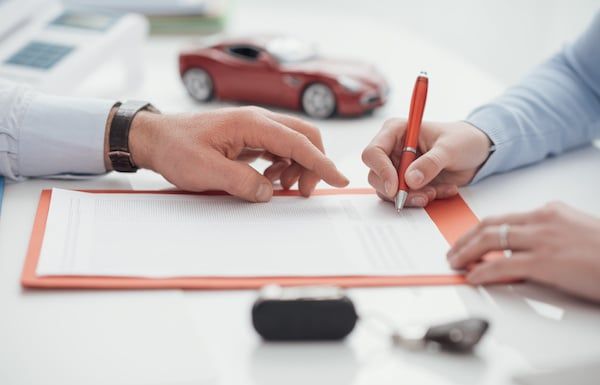
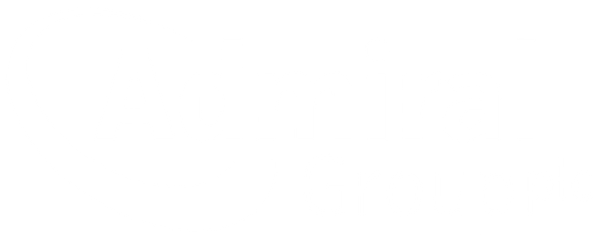
Delivering €2 Million in Incremental Annual Profit from Model-driven Insurance Product Recommendations
Data Science at ConTe.it and Admiral Group Europe
Worldwide, insurers face increasing consumer expectations for convenience, speed, and service. Many are turning to data science and real-time analytics to power innovative services and capabilities.
Take ConTe.it, for example. Headquartered in Rome, ConTe.it is the Italian brand for the Admiral Group, a direct insurance company specializing in auto and motorcycle insurance. With more than five million customers worldwide, the Admiral Group and its brands have made their mark in innovation, convenience, and customer satisfaction in the highly competitive insurance industry.
Francesco Maggina, ConTe.it’s Head of Data Science, expects within the next five years to make machine learning part of the company’s DNA and transform everything from how they price products for customers to how quickly they process claims. To this end, he and data science leaders from the company’s brands in Spain (under the Admiral Seguros brand) and France (under the L’olivier brand) have adopted the Domino data science platform to accelerate the development and deployment of algorithmic models that will help them better understand and serve their customers. Already, a new recommendation engine built and deployed on Domino enables ConTe.it to more quickly direct customers to the products they need. It has increased customer satisfaction and is on track to generate an incremental €2 million in profit, with more than €500,000 in additional revenue already captured through these targeted customer recommendations.
Challenge
For Francesco Maggina, one of his team’s biggest priorities is delivering real-time services for customers — whether providing real-time pricing and policies, or processing claims and providing customer payments within minutes of them submitting the request. “I expect that in three years we will be able to pay 70 percent of our claims within minutes,” said Maggina.
It will require a broad range of complex algorithmic models working in concert. For example, in the case of claims processing, models provide a real-time assessment of payments based on accident photos, predicted repair costs, policy information, and more. But to create innovative products and services like this, the company’s data science team needed a platform that would facilitate research, development, deployment, and monitoring of new models.
Data scientists previously used personal workstations to build models, which created a host of challenges that impeded innovation. These included:
- Process inefficiencies such as difficulties iterating on projects due to data and tool versioning issues or accessing compute resources to train more complex models.
- Lengthy deployment times as data scientists had to manually recode models for the production environment. In one case, it took six months for the team to convert a model from Python to Java for deployment.
- Inability to easily collaborate, including sharing ideas and code, and keeping a pulse on other projects within their team and across Europe with contractors and data scientists in other Admiral Group brands.
“Our first challenge at the European level was to select our data science platform that would help us improve data scientist productivity and performance so we could bring new services into production more quickly,” explained Maggina.
Solution
Together with his counterparts at Admiral Seguros and L’olivier and IT staff, Maggina launched the effort to identify a data science platform that could foster collaboration and accelerate data science efforts.
“We started with a list of 50 data science platforms and other solutions, and an extensive list of requirements covering DevOps, ModelOps, platform administration, privacy, security, training, support, and documentation. We narrowed the field down to three finalists—Domino, Amazon SageMaker, and Databricks—and then conducted in-person evaluations. In the end, we found that Domino was the best platform due to its security, ability to go live with models quickly, and comprehensive features.”
ConTe.it, Admiral Seguros, and L’olivier today all use the Domino data science platform to facilitate research, development, and deployment of machine learning models. The companies also plan to use Domino’s model monitoring capabilities to provide a single view of the health of all models. ConTe.it has deployed more than seven models on Domino since its adoption in 2018, and many others are under development.
With Domino, the teams can:
- Accelerate research and development of complex models. For example, one of ConTe.it’s first use cases on Domino is a recommendation system for both its digital channel and call center to provide each customer with customized information about optional products. Previously, the system generated a static pop-up with the same recommendations for everyone. “With Domino, we’re better able to build machine learning models to better understand and support customer needs,” said Maggina.
- Gain greater visibility into use cases in development. “We have many use cases in the pipeline using telematics data, GPS data, accident photos, and more to improve customer satisfaction and service, and we can see what’s working and what’s not across brands,” said Maggina.
- Rapidly deploy new models. Domino makes it easy for teams to export models to their production environment using application programming interfaces (APIs). “Prior to using Domino, we found that trying to deploy machine learning algorithms was a very manual, bespoke process. We could do it, but it was quite clear that an enabler like Domino could enable us to bring our work to production much faster,” said Maggina. “Our ultimate goal is to deploy models in less than ten days, and Domino makes this possible.”
- Deliver fast application response times. As data scientists develop pricing models that can provide customized policy rates and offers in just a few seconds, they must ensure that the Domino platform can answer “calls” from its digital systems in near real time. “We found in our tests that more than 99 percent of the time, the Domino platform can answer within 0.7 seconds, which will enable us to confidently embed machine learning models throughout our processes,” said Maggina.
The Domino Effect
- Increased customer satisfaction and expected €2 million in incremental profit annually. ConTe.it’s new recommendation engine, built on Domino, has helped the company understand customer needs more effectively. These customized customer interactions have led to more satisfied customers and a substantial bottom-line impact that includes increased revenue (from the sale of additional insurance products) and cost savings (from reducing call duration within its call center). “Already, in less than a year, we’ve identified €500,000 in additional revenue using A/B testing to measure the success of this new recommendation system,” said Maggina. “We are on track to deliver an estimated €2 million in incremental profit. This would not be possible without Domino to help us accelerate the work.”
- Reduced end-to-end model lifecycle by 83 percent. For example, the model that once took six months to deploy because data scientists had to translate it from Python to Java code to run in the production environment can now be deployed in just one month using Domino to create APIs with a few clicks. And for Maggina, this is only the beginning. “We are not taking advantage of all of Domino’s capabilities yet,” he explained. “As we expand our use, our time savings will increase.”
- Faster innovation cycles. As data scientists seek to update models, such as adding new data sources or expanding capabilities, they can now do it rapidly. “One of our priorities is also how quickly we can update models,” said Maggina. “With Domino, we can make any necessary changes in just a day, compared to a month previously, which will enable faster innovation as well.”
INDUSTRY
Insurance
Financial Services
LOCATION
Headquarters: Rome, Italy
USE CASES
Recommendation System
IMPACT
Expected €2 million in incremental annual profit with €500,00 in additional revenue realized to date
Reduced model deployment from 6 months to 1 month
Reduced time to refresh models from 1 month to 1 day
DATA SCIENCE SCALE
7 models in production, and rapidly growing
USERS
5 data scientists within ConTe.it
11 data scientists across Admiral’s European brands
External data science consultants
SOLUTION COMPONENTS
Data Science Platform: Domino
Data Science Tool(s): R, Python
Server / Cloud Infrastructure: Amazon Web Services
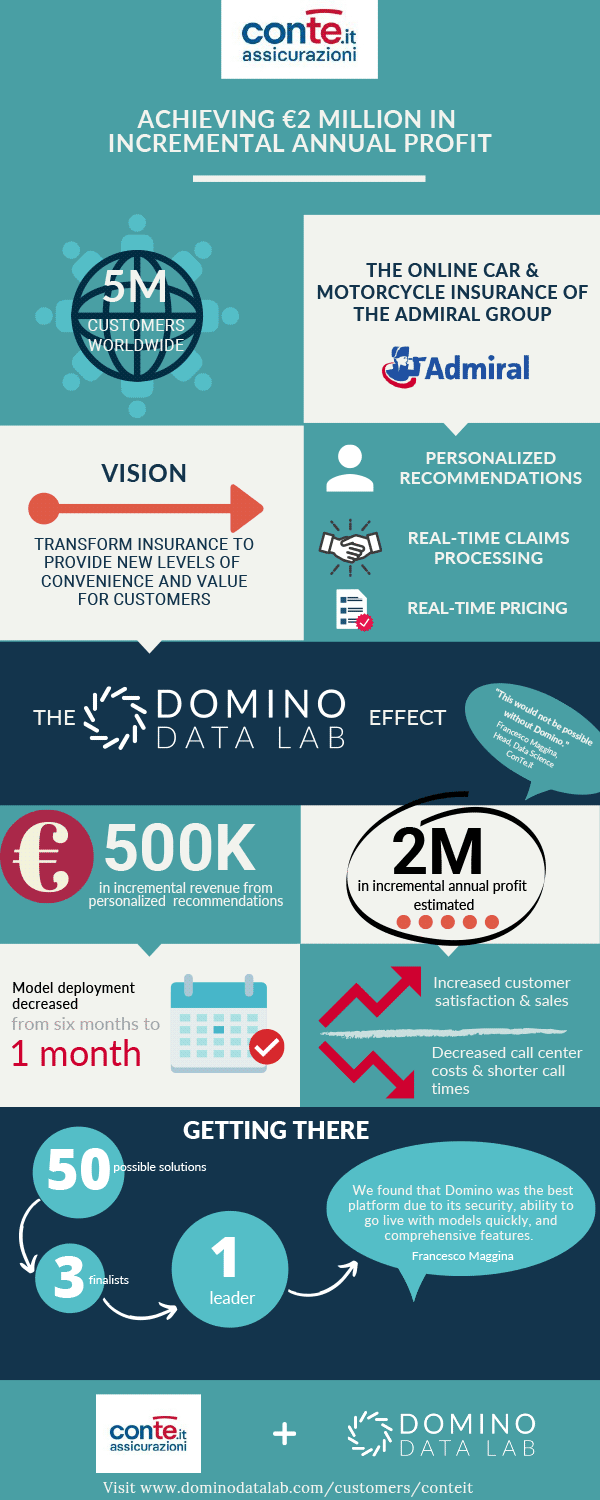