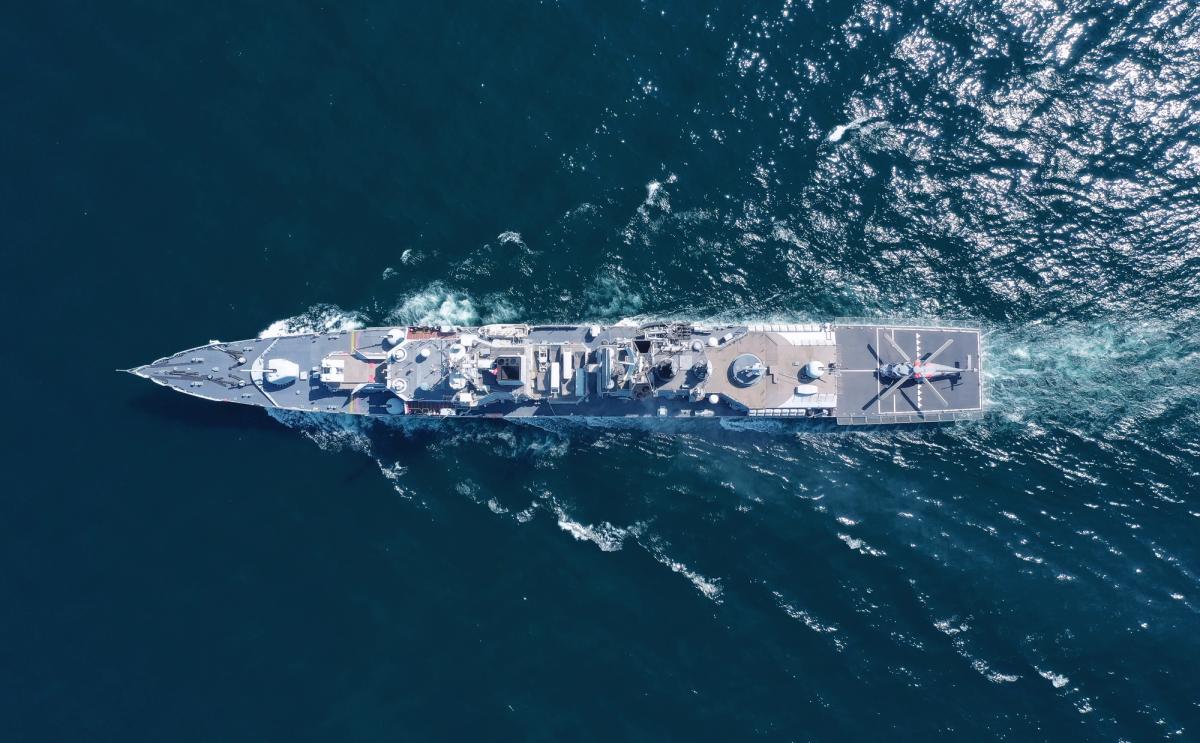
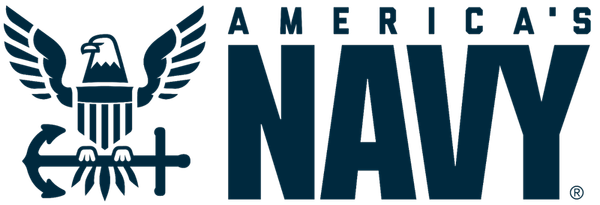
U.S. Navy makes mine detection intelligence more trustworthy with Domino
Situation
For years, the U.S. Navy relied on machine learning (ML) models to support underwater target threat detection by unmanned underwater vehicles (UUV). However, it lacked a way to monitor and improve ML model performance at scale post-deployment because models were slow to adapt to changing underwater conditions or enemy tactics. Without the ability to monitor or maintain ML models, mission-critical systems risk producing inaccurate or unreliable intelligence that drives decision-making.
“One of the things that we discovered through that process was that as these unmanned vessels were out there doing what they did, [when] they came back, we needed to be able to update their software based on what they just learned,” said Commander Eli Ford, Principal Assistant Program Manager, Underwater Explosive Ordnance Disposal, PMS 408 (Expeditionary Missions).
In 2022, the Navy partnered with the Defense Innovation Unit (DIU) to improve ML model performance gaps by leveraging commercial technology that could be integrated into the Navy’s existing systems. Using DIU’s Commercial Solutions Opening (CSO) process, the team identified commercial machine learning operations (MLOps) vendors who could collectively provide a pipeline to track, modify, and redeploy underwater target threat detection ML algorithms.
“The partnership between the U.S. Navy and DIU has opened the pathways to commercial innovation and has produced a MLOps toolset that ensures our solutions adapt and evolve at the speed of tactical relevance, placing an enduring capability in the hands of the warfighter. Nowhere was this more apparent than at an Integrated Battle Problem in the Fall of 2023 where sensor data was collected, new models were trained against an expanded threat environment, and updated detection models were deployed through the AMMO ecosystem back into the hands of the operator,” said Ford.
To solve this, the U.S. Navy's Expeditionary Missions team partnered with the Defense Innovation Unit to identify commercial technologies, including Domino, that deliver a potentially transformative effect in these maritime scenarios carried out by Automated Machine Learning for Mine Countermeasures Operations (AMMO). AMMO capabilities helped address the subsea/seabed warfare (SSW) mission by improving the Navy’s ability to detect targets of interest in undersea environments. AMMO also allows the Navy’s ML developers to focus on model creation and refinement tasks, allowing the tools to handle the critical aspects of deployment, monitoring, and governance.
Solution
The U.S. Department of Defense (DoD) built a successful AI model prototype to close a critical gap in underwater mine intelligence. The Domino Enterprise AI Platform helped quickly deploy and retrain ML models reliant on data collected from automated underwater mine detection vehicles, improving undersea warfare essential to U.S. success in the Pacific and other contested environments.
The Domino Enterprise AI Platform is now an integral component of a modern MLOps pipeline for mine countermeasures (MCM) AI-based model development and deployment in the cloud. The Domino Enterprise AI Platform helps the U.S. Navy deploy and retrain automatic target recognition (ATR) models dozens of times faster than before — enabling naval decision-makers to have higher confidence in field-generated insights from unmanned underwater vehicles. Automatic target recognition is the ability of an algorithm or device to recognize targets, such as mines, based on data obtained from sensors.
As a modular, open, and extensible platform hosted in AWS GovCloud (US), Domino enables the U.S. Navy’s Project AMMO’s distributed teams to deploy models faster with built-in observability and reduced time-to-impact. Users can leverage the best open source and commercial tools of their choice in a highly governed way, on one platform, to generate and analyze ATR field-generated insights. In addition, Domino’s built-in model portability allows these teams to easily convert and export models into external formats to far-edge platforms. It also allows users to securely access and share structured and unstructured data from anywhere.
“It's highly encouraging to see Domino, the Navy, and DIU partnering to rapidly field commercial tech sector capabilities in order to deter conflict. This type of teaming, with Domino’s core MLOps technology at its center, is an example of a rapid, repeatable, and scalable template that will significantly improve our nation's defenses,” said Brigadier General Bobby Kinney.
Kinney is deeply familiar with both the needs of the DoD and its AI-driven missions, having previously served as the Missions Director at the Joint Artificial Intelligence Center (JAIC) under the Office of the Secretary of Defense (OSD). During his time at JAIC, he was responsible for leading AI coordinating activities and accelerating the delivery of $250M a year of high-impact AI projects across the Department of Defense (DoD).
Impact
Autonomy is a key feature of next-generation warfighting, with trusted AI enabling those systems. Commercial AI solutions like Domino are critical to accelerating the DoD’s ability to field autonomous capabilities across domains, particularly underwater. As an open, scalable, yet highly governable platform, Domino helps the U.S. Navy’s Project AMMO deploy cutting-edge commercial tech to enable the fleet with rapidly updated models that deliver a higher level of trust in intelligence to power their missions.
With Domino, the U.S. Navy’s AMMO team was able to decouple hardware and software updates to dramatically accelerate updates to ATR models at the edge. This results in more accurate imagery and sonar-based intelligence that can be trusted and actioned by naval decision-makers for next-generation warfare advantage.
Using Domino as the factory for integrating four other commercial technologies, three different contracted teams were able to accelerate their work, reducing the time to deploy AI models at the edge — to two weeks down from six months — and retraining the models in two weeks rather than 12 months.
“Working with multiple international partners, we demonstrated the ability to deploy and update our automatic target recognition models at the speed of operational relevance, while simultaneously remaining confident in their performance. This embodiment of human-machine teaming gives our sailors a powerful tool in their arsenal and increases confidence in their systems,” said Commodore Shaun Lieb, Commander, Task Force (CTF) 75.
The U.S. Navy’s Project AMMO also sets the stage for the DoD to deploy and manage ML algorithms effectively at scale, through MLOPs practices that ensure the safe, reliable, and effective operation of all ML models that advance trust in new AI technologies.
“I'm proud our warfare community could be the pathfinder and exemplar for machine learning ops, tooling, and processes that will improve many other Navy and joint service warfighting capabilities in the age of artificial intelligence,” said Commodore Shaun Lieb, Commander, Task Force (CTF) 75.
INDUSTRY
Government
LOCATION
Headquarters: Washington, D.C.
USE CASES
Improve AI-based target recognition reliant on underwater sensor data so threat intelligence is more trustworthy for decision-making
Accelerate time to train and deploy models against an expanded threat environment
Leverage modern MLOPs practices to operationalize AI at scale in the cloud
Securely collaborate with multiple contractors and agencies
IMPACT
ML model deployment time went from 6 months to 2 weeks
ML model retraining time went from 12 months to 2 weeks
Securely operated in an AWS GovCloud (US) DoD IL5 environment at the edge