Become A Full Stack Data Science Company
Hoda Eydgahi2018-02-22 | 10 min read
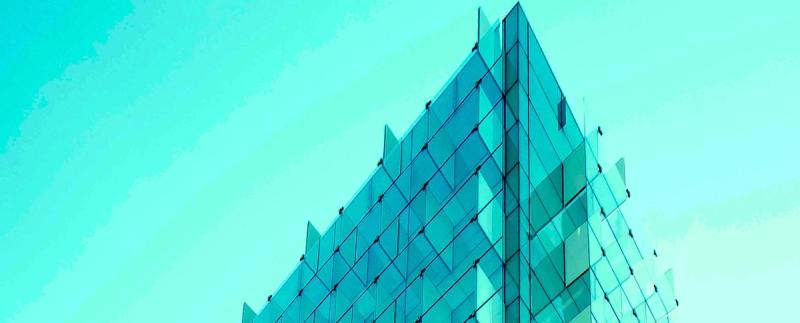
In this post, Hoda provides insight into how companies with a growing data science capability can structure their organization to ensure that data science provides them with a competitive advantage.
Become A Full Stack Data Science Company
"Deep learning to do X" is the new investor pitch trend.
The rise of big data and advancements in machine learning techniques are driving the next generation of tech companies. Data science and algorithms enable the most successful companies to build the moat they need to retain their competitive edge. Successful tech companies also adapt their organizational structure to empower those who bring the most unique and differentiated value to their company. If data science is such a critical piece of your company’s competitive strategy, then you should take the time to consider: how to structure your data science capability, how to define the role of your data scientists, and how to empower data science at all levels of your company.
The Rise of Software Engineering
Over the last decade, we’ve seen an explosion in tech companies and the rise in the ranks of software engineers. It is not uncommon to see companies, especially young ones, where most of the founders or the entire product and engineering organizations are engineers. Previously, the norm included hiring traditional MBAs with little to no domain expertise as managers over highly technical teams. Now engineers are encouraged and empowered to make lateral switches and take up managerial positions. Bringing in just a business-focused specialist CEO at a growing tech company is no longer a thing. CEOs either have a technical background or savvy business-oriented CEOs quickly bring in domain and technical expertise at the C-Level to support company strategy. Tech companies seek out, trust, and follow leaders who are committed to understanding the guts of what the company is doing.
The Rise of Data Science
We’re now seeing a similar trend in data science.
In its nascent days, data science was treated as the baby sibling of engineering: sitting as a sub-unit inside the engineering organization. As both data science and engineering share technical underpinnings, this was a reasonable starting point.
Yet they are not the same.
While both data scientists and engineers write software to get the job done, their approaches towards problem solving, the way in which their success is evaluated, and their needs to perform their roles well, are different. As data science grows in stature and companies start to build businesses around it, it's time to rethink how data science is placed in the organizational structure. I believe data science should be given appropriate attention and autonomy. It should not be put under the engineering or product organizational umbrella. Rather, it should be represented at every level of the company: from the top C-Level to the foundational individual contributor level.
Stitch Fix is Organized as a Full Stack Data Science Company
Stitch Fix is an online retailer that offers personal styling as a service. We use a combination of algorithms and expert human judgment to style our clients. Much of our competitive advantage over traditional retailers can be attributed to the strong focus we have on using data science in every level of the business. We use algorithms to empower our stylists to best serve our clients; to determine the optimal route for picking items in our warehouses; and to even suggest our own clothing designs to our merchandising team.
In order to realize our data science org’s full potential, we’ve made a commitment to data science representation at every level of the organization. Stitch Fix not only has one of the larger data science teams in the valley, it has one of the largest data science teams relative to its size: 1 in 7 HQ employees is a data scientist. We also have a Chief Algorithms Officer (CAO) who represents the data science organization at the executive level.
Why Data Science at the C-Level Matters
When data science is your company’s competitive edge, having a CAO represent data science at the executive level is more effective than being represented by an engineering head, like a CTO. The CAO has a deeper and more nuanced understanding of data science --- from strategy to execution, than someone, like a CTO, whose priorities may lie elsewhere outside of data science. For example, engineering specializes in end-user interaction, transaction processing, and the like. While important, data scientists are not particularly great at these capabilities. Therefore, having a separation between data science and engineering empowers each team to focus on what they are great at.
Our CAO helps the data science organization manage expectations and prioritize the data science hierarchy of needs appropriately. These needs include data collection, ETL, pipelines, and understanding that infrastructure is needed long before we get to the sexy deep learning or machine learning algorithms. Having C-Level support helps us set the proper foundation and enables the data science org to scale seamlessly like the rest of the business. It also helps manage cross-functional stakeholder expectations and remove administrative overhead so that our individual contributors can focus on being full stack data scientists.
The Full Stack Data Scientist Responsibilities
At Stitch Fix, we don’t abide by the data-scientist-in-the-corner model where data scientists are isolated from the rest of the company. We don’t overload our data scientists with running SQL queries for the rest of the organization. We don’t have data scientists who focus solely on developing mathematical models that eventually get passed on to someone in engineering to be put in production. We don’t even have a single project manager in our data science organization. Rather, we believe in the “full stack” data scientist.
Our full stack data scientists do it all: engineering, machine learning, and project management. We combine all of these domains into one role because there is simply too much value in the process of iteration. For non-full stack data scientists, the workflow happens something like this:
- a data scientist builds mathematical models
- then hands the models to engineering to productionize
- then every time model coefficients need to be updated or a new feature needs to be added, the data scientist has to wait for the engineer (who is part of an organization with different priorities) to implement the changes.
This slower iteration process can be demotivating to the data scientist because something he or she cares about isn’t as important to someone on a different organization (and likewise for the engineer). The full stack model enables faster iteration process and enables our data scientists to become the domain experts of a particular capability and invaluable partners to their counterparts in other parts of the business.
Empowering Data Science at Every Level as Company Culture
Company culture is typically set within the early days of a startup. If data science is your company’s competitive differentiator, then having a CAO in the executive suite is a signal that your company values data science as a stand-alone capability. It also signals how important having a data-centric approach to solving key strategic business problems is to your company. While Stitch Fix has significant investment in data science at all levels of the company, if you are just starting out, you do not need a lot of hierarchy. Stitch Fix’s data science organization started with a CEO and a single data scientist, then grew from there.
Over the years, Stitch Fix’s data science org has evolved to have more layers. At the C-Level we have a CAO. The VP of Data Science and VP of Data Platform report to the CAO since both orgs work hand in hand and need to be closely aligned. The data engineers on the Platform team build the tools, frameworks, and services that the data scientists, in turn, leverage to build ETLs, construct models, and deploy in production. Our directors and managers help manage stakeholder expectations and remove administrative overhead so that individual contributors can focus on being full stack data scientists. As Stitch Fix grew, so did our data science organization.
Takeaway
If data science is truly your competitive advantage, treat it as such. If data science is worth highlighting in your investor pitch deck, then at the very least give the data science organization its own voice in the C-suite. Having data science present not only ties business value to what the overall data science team is doing, but also enables data-driven strategic decision making. Give data scientists end to end ownership and put them on the same plane as engineers. Only such proactive measures to support your data science org will let reach its full potential.
Hoda Eydgahi is a Data Science Manager at Stitch Fix and a Scout for Sequoia Capital. Previously, Hoda was the first Data Scientist at Color Genomics as well as a Co-founder and CTO of Bluelight Global. An entrepreneur at heart, she is an advisor to and investor in numerous startups. She holds a BS in Biomedical Engineering from Virginia Commonwealth University and an MS and PhD in Electrical Engineering and Computer Science from the Massachusetts Institute of Technology.
Summary