Bias: Breaking the Chain that Holds Us Back
Domino2018-04-05 | 17 min read
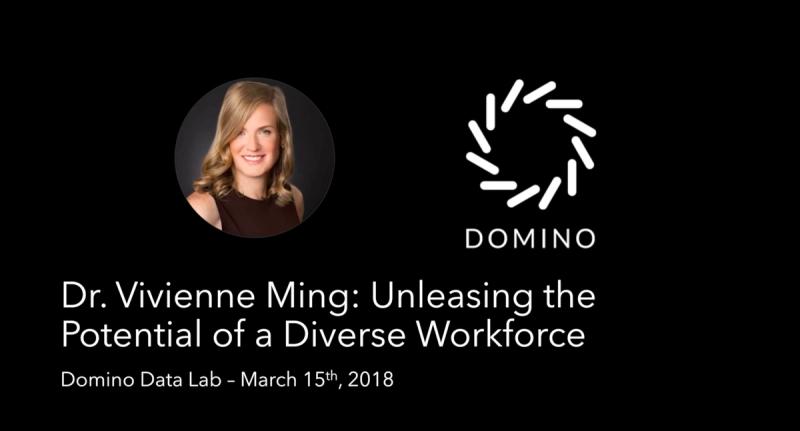
Speaker Bio: Dr. Vivienne Ming was named one of 10 Women to Watch in Tech by Inc. Magazine, she is a theoretical neuroscientist,entrepreneur, and author. She co-founded Socos Labs, her fifth company, an independent think tank exploring the future of human potential. Dr. Ming launched Socos Labs to combine her varied work with that of other creative experts and expand their impact on global policy issues, both inside companies and throughout our communities. Previously, Vivienne was a visiting scholar at UC Berkeley's Redwood Center for Theoretical Neuroscience, pursuing her research in cognitive neuroprosthetics. In her free time, Vivienne has invented AI systems to help treat her diabetic son, predict manic episodes in bipolar sufferers weeks in advance, and reunited orphan refugees with extended family members. She sits on boards of numerous companies and nonprofits including StartOut, The Palm Center, Cornerstone Capital, Platypus Institute, Shiftgig, Zoic Capital, and SmartStones. Dr. Ming also speaks frequently on her AI-driven research into inclusion and gender in business. For relaxation, she is a wife and mother of two.
Distilled Blog Post Summary: Dr. Vivienne Ming's talk at a recent Domino MeetUp delved into bias and its implications, including potential liabilities for algorithms, models, businesses, and humans. Dr. Ming’s evidence included first-hand knowledge fundraising for multiple startups, data analysis completed during her tenure as the Chief Scientist at Gild, as well as citing studies within data, economics, recruiting, and education. This blog post provides text and video clip highlights from the talk. The full video is available for viewing. If you are interested in viewing additional content from Domino’s past events, review the Data Science Popup Playlist. If you are interested in attending an event in-person, then consider the upcoming Rev.
Research, Experimentation, and Discovery: Core of Science
Research, experimentation, and discovery are at the core of all types of science, including data science. Dr. Ming kicked off the talk with indicating “one of the powers of doing a lot of rich data work, there's this whole range-- I mean, there's very little in this world that's not an entree into”. While Dr. Ming provided detailed insights and evidence that pointed to the potential of rich data work during the entire talk, this blog post focuses on the implications and liabilities of bias within gender, names, and ethnic demographics. It also covers how bias isn’t solely a data or algorithm problem, it is a human problem. The first step to address bias is acknowledging that it exists.
Do You See the Chameleon? The Roots of Bias
Each one of us has biases and makes assessments based on those biases. Dr. Ming uses Johannes Stotter’s Chameleon to point out that “the roots of bias are fundamental and unavoidable”. Many people when they see the image, see a chameleon. However, the chameleon image consists of two people covered in body paint and is strategically placed to look like a chameleon. In the video clip below, Dr. Ming indicates
“I cannot make an unbiased AI. There are no unbiased rats in the world. In a very basic sense, these systems are making decisions on their uncertainty, and the only rational way to do that is to act the best we can given the data. The problem is when you refuse to acknowledge there's a problem with our bias and actually do something about it. And we have this tremendous amount of evidence that there is a serious problem, and it's holding, not just small things back. But as I'm going to get to later, it's holding us back from a transformed world, one that I think anyone can selfishly celebrate.”
Bias as the Pat on the Head (or the Chain) that Holds Us Back
While history is filled with moments when bias is not acknowledged as a problem, there are also moments when people addressed societal-reinforced gender bias. Women have assumed male nom de plumes to write epic novels, fight in wars, win Judo championships, run marathons, and even, as Dr. Ming pointed out, create an all-women software company called Freelance Programmers in the 1960s. During the meetup, Dr. Ming indicated that Dame Stephane “Steve” Shirley’s TedTalk, “Why do ambitious women have flat heads?”, helped her parse two distinctly different startup fundraising experiences that were grounded in gender bias.
Prior to Dr. Ming co-founding her current education technology company and obtaining her academic credentials, she dropped out of college and started a film company. When
“we started this company, and the funny thing is, despite having nothing, nothing that anyone should invest in-- we didn't have a script. We didn't have talent. Literally, we didn't even have talent. We didn't have experience. We had nothing. We essentially raised what you might in the tech industry called seed round after a few phone calls.“
However, raising funding was more difficult the second time, for her current company, despite having substantially more academic, technology, and business credentials. During one of the funding meetings with a small firm with 5 partners, Dr. Ming relayed how the last partner said “‘you should feel so proud of what you've built’. And at the time, I thought, oh, Jesus, at least one of these people is on our side. In fact, as we were leaving the room, he literally patted me on the head, which seemed a little strange.” This prompted Dr. Ming to consider how
“my credentials are transformed that second time. No one questioned us about the technology. They loved it. They questioned whether we know how to run a business. The product itself people loved versus a film. Everything the second time around should have been dramatically easier. Except the only real difference that I can see is that the first time I was a man and the second time I was a woman.“
This led Dr. Ming to conclude and understand what Stephanie Shirley meant by ambitious women having flat heads from all of the times they have been pat on the head. Dr. Ming relays that
“I've learned ever since as an entrepreneur is, as soon as it feels like they're dealing with their favorite niece rather than me as a business person, then I know, I know that they simply are not taking me seriously. And all the Ph.D.'s in the world doesn't matter, all the past successes in my other companies doesn't matter. You are just that thing to me. And what I've learned is, figure that out ahead of time. Don't bother wasting days and hours, and prepping to pitch to people that simply are not capable of understanding who you are, but of course, in a lot of context, that's all you've got.“
Dr. Ming also pointed out that the bias due to gender also manifested at an organization where she worked before and after her gender transition. She noted when she went into work after her gender transition,
“That's the last day anyone ever asked me a math question, which is kind of funny. I do happen to also have a PhD in psychology. But somehow one day to the next, I didn't forget how to do convergence proofs. I didn't forget what it meant to invent algorithms. And yet that was how people dealt with it, people who knew before. You see how powerful the change is to see someone in a different skin.”
This experience is similar to Dame Shirley’s, who, in order to start what would become a multi-billion dollar software company in the 1960s, "started to challenge the conventions of the time, even to the extent of changing my name from "Stephanie" to "Steve" in my business development letters, so as to get through the door before anyone realized that he was a she". Dame Shirley subverted bias during a time when she, as a female, was prevented from working on the stock exchange, driving a bus, or, “Indeed, I couldn't open a bank account without my husband's permission”. Yet, despite the bias, Dame Shirley remarked
"who would have guessed that the programming of the black box flight recorder of Supersonic Concord would have been done by a bunch of women working in their own homes” ….”And later, when it was a company valued at over three billion dollars, and I'd made 70 of the staff into millionaires, they sort of said, "Well done, Steve!"
While it is no longer the 1960s, bias implications and liabilities are still present. Yet, we in data science are able to access data to have open conversations about bias as the first step avoiding inaccuracies, training data liabilities, and model liabilities within our data science projects and analysis. What if, in 2018, people built and trained models based on the assumption that humans with XY chromosomes lacked the ability to code because they only reviewed and used data from Dame Shirley’s company in the 1960s? Consider that a moment, as that is what happened to Dame Shirley, Dr. Ming, and many others. Bias implications and liabilities have real-world consequences. Being aware of the bias and then addressing it, moves the industry forward towards breaking the chain that holds research, data science, and us, back.
Say My Name: Biased Perceptions Uncovered
When Dr. Ming was the Chief Scientist at Gild, a reporter called her for a quote on the Jose Zamora story. This also led to Dr. Ming’s research on her upcoming book. “The Tax of Being Different”, Dr. Ming relayed anecdotes during the meetup (see video clip) and has also written about this research for the Financial Times:
"To calculate the tax on being different I made use of a data set of 122m professional profiles collected by Gild, a company specializing in tech for hiring and HR, where I worked as chief scientist. From that data, I was able to compare the career trajectories of specific populations by examining the actual individuals. For example, our data set had 151,604 people called “Joe” and 103,011 named “José”. After selecting only for software developers we still had 7,105 and 4,896 respectively, real people writing code for a living. Analyzing their career trajectories I found that José typically needs a master's degree or higher compared to Joe with no degree at all to be equally likely to get a promotion for the same quality of work. The tax on being different is largely implicit. People need not act maliciously for it to be levied. This means that José needs six additional years of education and all of the tuition and opportunity costs that education entails. This is the tax on being different, and for José that tax costs $500,000-$1m over his lifetime.” (Financial Times)
While this particular example focuses on ethnicity-oriented demographic bias, during the meetup discussion, Dr. Ming referenced quite a few research studies regarding name bias. In case Domino Data Science Blog readers do not have some of research she cites on hand, a sample of studies have published around bias with names include: names that suggest male gender, “noble-sounding” surnames in Europe, names that are perceived as “easy-to-pronounce” which also has implications for how organizations choose their names. Yet, Dr. Ming did not limit the discussion to bias within gender and naming, she also dived right into how demographic bias impacts image classification, particularly with ethnicity.
Bias within Image Classification: Missing Uhura and Not Unlocking your iPhone X
Before Dr. Ming was the Chief Data Scientist at Gild, she was able to see Paul Viola’s face recognition algorithm demo. In that demo, she noticed that the algorithm didn’t detect Uhura. Viola indicated that this was a problem and it would be addressed. Fast forward years later to when Dr. Ming was the Chief Scientist at Gild, she relayed how she received “a call from The Wall Street Journal [and WSJ asked her] ‘So Google's face recognition system just labeled a black couple as gorillas. Is AI racist?’ And I said, ‘Well, it's the same as the rest of us. It depends on how you raise it.’“
For background context, in 2015, Google released a new photo app and a software developer discovered that the app labeled two people of color as “gorillas” and Yonatan Zunger was the Chief Architect for Social at Google at the time. Since Yonatan Zunger is no longer at Google, he has since provided candid commentary about bias. Then, in January 2018, Wired ran a follow-up story regarding the 2015 event. In the article, Wired tested Google Photos and found that the labels for gorillas, chimpanzees, chimp, and monkey “were censored from searches and image tags after the 2015 incident”. This was confirmed by Google. Wired also ran a test to assess view of people by conducting searches for “African American”, “black man”, “black woman”, or “black person” which resulted in “an image of a grazing antelope” (on the search “African American”) as well as “black-and-white images of people, correctly sorted by gender but not filtered by race”. This points to the continued challenges involved with addressing bias in machine learning and models. Bias also has implications beyond social justice.
As Dr. Ming pointed out in the meetup video clip below, facial recognition is also built into the iPhone X. The face recognition feature has potential challenges in recognizing global faces of color. Yet, despite all of this, Dr. Ming indicates “but what you have to recognize, none of these are algorithm problems. These are human problems.” Humans made decisions to build algorithms, build models, train models, and roll out products that include bias that has wide implications.
Conclusion
Introducing liability into an algorithm or model via bias isn’t solely a data or algorithm problem, it is a human problem. Understanding that it is a problem is the first step in addressing it. In the recent Domino Meetup, Dr. Ming relayed how
“AI is an amazing tool, but it's just a tool. It will never solve your problems for you. You have to solve them. And particularly in the work I do, there are only ever messy human problems, and they only ever have messy human solutions. What's amazing about machine learning is that once we found some of those issues, we can actually use it to reach as many people as possible, to make this essentially cost-effective, to scale that solution to everyone. But if you think some deep neural network is going to somehow magically figure out who you want to hire when you have not been hiring the right people in the first place, what is it you think is happening in that data set?”
Domino continually curates and amplifies ideas, perspectives, and research to contribute to discussions that accelerate data science work. The full video of Dr. Ming’s talk at the recent Domino MeetUp is available. There is also an additional technical talk that Dr. Ming gave at the Berkeley Institute of Data Science on “Maximizing Human Potential Using Machine Learning-Driven Applications”. If you are interested in similar content to these talks, please feel free to visit the Domino Data Science Popup Playlist or attend the upcoming Rev.
Domino Data Lab empowers the largest AI-driven enterprises to build and operate AI at scale. Domino’s Enterprise AI Platform provides an integrated experience encompassing model development, MLOps, collaboration, and governance. With Domino, global enterprises can develop better medicines, grow more productive crops, develop more competitive products, and more. Founded in 2013, Domino is backed by Sequoia Capital, Coatue Management, NVIDIA, Snowflake, and other leading investors.