Considering a Job Change? Here’s Must-read Advice from 3 Top Data Science Leaders & Innovators
Lisa Stapleton2022-09-07 | 8 min read
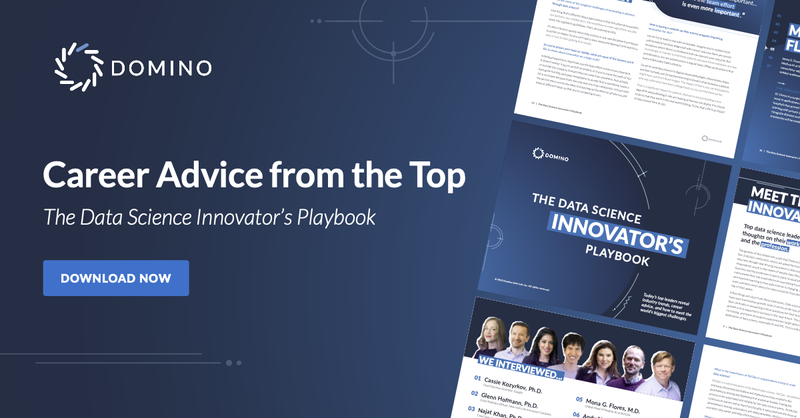
If you’re working in data science and are feeling like making a job change, here are some things top data science innovators say to consider as you look for a new opportunity or plan your next career move. Their career advice–and much more–is captured in the new ebook, The Data Science Innovator’s Playbook, from Domino Data Lab.
The good news is that you’re in a field that’s highly desirable, so odds are, you have several options for your next job, whether it’s within your current company or at a new employer. So what’s the best way to think about your data science career?
Career Advice for Job Hunts–and Your Entire Working Life
Google’s Chief Decision Scientist Cassie Kozyrkov offers career advice that holds not just for weeding out opportunities that might be a bad fit for your talents and aspirations, but for setting goals that will make you happy in the long run.
“My biggest success in life is the same as what I’d wish for every person on the planet: taking the time to understand oneself well enough to design personally-fulfilling goals rather than pursuing a life that would be a better fit for someone else,” she explains.
“And that also means saying no to some things that everyone around you seems to want,” she concludes. “Every single person is unique - we’re all weirdos in our own way, so real success has to start with designing our own lives instead of copying the lives of others.”
Don’t Work for Jerks–and Don’t Let Your Job Turn You Into One
John K. Thompson, whose career has included data science leadership at several large companies, has literally written the book on Building Analytics Teams, and he offers extensive career advice for data scientists in the Data Science Innovator’s Playbook and in an accompanying blog.
For everyone, at all career stages, he offers the following advice: “Don’t work for jerks.” And don’t be one, either, if you value your long-term job satisfaction and happiness.
“One of my MBA professors taught us that what you become at work, will have a direct and dramatic impact on your personal life,” says Thompson.
“The two cannot be separated. If you are rude and unbearable at work, you’ll be that way in your personal life,” he adds.
Thompson also offers specific advice for early-career, mid-career, and senior data scientists and their leaders:
Young data scientists
- Be curious.
- Learn every day.
- Take chances.
Mid-career professionals
- Strive to take on ever increasing levels of complexity, do not become complacent and solve the same problem over and over and over.
- Help younger staff members.
- Help your community.
- Be generous with your time and knowledge.
Seasoned data science leaders
- Be aware that you don’t know as much as you think you do.
- Learn from younger staff members.
- Give back to the data science community.
Look for Companies that Provide the Necessary Conditions for Professional Growth
Top data science leader Glenn Hofmann offers advice that’s insightful for both leaders looking to retain data scientists, and for data scientists looking to make a change.
He advises data science leaders who want to hire, grow, and retain data science talent to “Remember that data scientists and everyone else within a data science team–including the MLOps engineers, operations, and program managers–want to see that their work is making a difference. Ensuring that models are deployed and used by the business will help with both attracting talent to your team and retaining talent. Also, to attract talent, you need to provide the right resources, career paths, training programs, and learning stipends.”
Savvy data scientists looking for new opportunities know this, and look for organizations that resource their teams properly, have good career paths for their best talent, sponsor interesting training programs, and provide ample budget for learning stipends.
Look for Firms that Minimize IT-Data Scientist Conflicts
Many data science job seekers are looking for a more collaborative environment than they’re experiencing in their current job. Data science is increasingly a collaboration not just within teams of data scientists themselves; rather, it's often a cross-departmental effort that is at times somewhat fraught.
For example, the relationship between data scientists and IT is surprisingly problematic. A DataIQ survey found that about one-third (33.7%) of the organizations they surveyed see 'conflict between data science and IT’ as one of their biggest challenges. Even companies that rate themselves as ‘Advanced’ or ‘Reaching Maturity’ in terms of their level of adoption of data and analytics are not immune to conflict. For both of these groups, ‘conflict between data science and IT’ is their biggest challenge (52.4% and 50% respectively).
“Conflict between data science and IT manifests itself in many ways, and it often starts because IT treats data science models like they do other software projects,” says Thompson. Part of easing conflict comes from analyzing the different pressures and requirements placed on IT and data science departments. IT pros don’t always understand how different modeling can be from software development.
“Models require re-training, are developed in an experimental fashion, and are made using many different software tools. There is no need to ‘retrain’ software code, but [data science] production models need to be retrained frequently,” he says.
Part of the remedy for the situation might be the realization that data science—and data scientists—don’t fit neatly into existing structures. For that reason, it’s necessary to rethink a host of related practices and established ways of thinking about what data scientists do and how they work.
“Companies that realize the most significant value from data science understand that models are a new type of digital life that calls for different people, processes, and platforms [than those that IT typically supports],” says Thompson.
For more on hiring and retaining data scientists in 2022, check out this helpful page, "Managing Data Science Teams."
Other Featured Innovators Weigh in on Data Science Career Strategies
Download the free The Data Science Innovator’s Playbook to read more career advice and insights from Thompson, Kozyrkov, and Hofmann–as well as from many others. The complete list of top innovators featured in the book includes:
- Cassie Kozyrkov—Chief Decision Scientist, Google, who describes how the jobs of data scientists, data engineers, and others in the data science ecosystem, are changing.
- Andy Nicholls—Senior Director, Head of Statistical Data Sciences, GSK plc, who describes opportunities for data scientists, MLOps engineers, and others, to streamline many parts of the drug approval and clinical trial processes.
- Mona G. Flores—Global Head of Medical AI at NVIDIA, who talks about opportunities to apply ML and AI to transform the business operations of medicine and pharma.
- Najat Khan—Chief Data Science Officer and Global Head, Strategy & Operations for Research & Development at the Janssen Pharmaceutical Companies of Johnson & Johnson, who talks about what makes data science teams successful.
- Robert Nishihara—Co-creator of Ray, and Co-founder & CEO, Anyscale, who talks about the skills data scientists will need as distributed resources become easier to use.
- John K. Thompson—Analytics Thought Leader, Best-selling Author, Innovator in Data & Analytics, offers more insight on growing innovative teams.
- Glenn Hofmann—Chief Analytics Officer, New York Life Insurance Co., talks about building a successful data science team.
Lisa Stapleton is a technology writer and editor in San Jose, CA. She has written and edited for Infoworld, InformationWEEK, LinuxInsider.com, and many other business and technical publications. She is now Domino's Content Director.
Summary
- Career Advice for Job Hunts–and Your Entire Working Life
- Don’t Work for Jerks–and Don’t Let Your Job Turn You Into One
- Look for Companies that Provide the Necessary Conditions for Professional Growth
- Look for Firms that Minimize IT-Data Scientist Conflicts
- Other Featured Innovators Weigh in on Data Science Career Strategies