Unleash enterprise AI: BARC report points the way for IT
Yuval Zukerman2024-03-27 | 7 min read
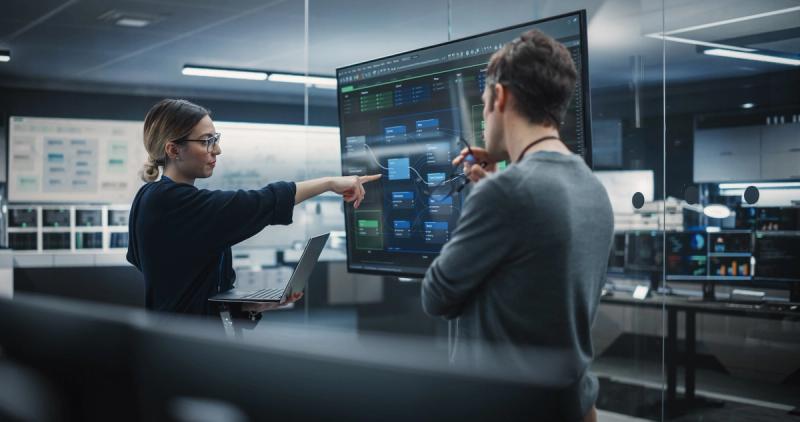
As an IT professional, you are the enterprise's gatekeeper. You guard valuable data assets and secure ongoing operations. AI is happening everywhere around you. Some colleagues are using ChatGPT. Others use Midjourney. Now, the CEO wants you to make AI happen for everyone. And yes, why not the cafeteria staff, too? It's a big task, but luckily, you're not alone.
A recent BARC survey report titled, “Optimizing Your Architecture for AI Innovation,” will give you some ideas on where to start. The report offers the clear, realistic AI answers you need from veteran IT analysts, Shawn Rogers and Merv Adrian. To cut to the chase, almost everyone (87%) wants IT to drive this revolution. 95% want your help with strategy and some budget, too. So, what is slowing AI adoption down?
Enterprise AI adoption barriers
AI’s introduction to business follows a well-known pattern seen with other new technologies. Few have deep expertise, and the path to competence is complicated. 85% of those surveyed encountered challenges while trying to implement AI solutions. These obstacles spanned from the technical through the structural, highlighting the complex nature of successful AI adoption. What barriers, then, should you expect to deal with?
- AI skill shortage: 39% of companies face skill shortages, and this gap worsens as AI adoption grows. Staying on top of the latest technology is never easy. AI is a fast-changing space, so even capable teams can fall behind.
- Costs: 45% of companies with live AI deployments were concerned about costs. AI infrastructure, model development, and maintenance can be expensive, especially if generative AI (GenAI) is involved. Many companies use cloud services for AI. If you follow this route, you will likely need to sign pricey long-term contracts, too.
- Collaboration: AI is a team sport. Many groups need to contribute, from domain experts to data experts, security specialists, legal advisors, and more. As you know, getting them all in the same room is not easy. Such cross-functional collaboration is difficult enough for 24% of respondents to flag. 20% also expressed worries about leadership and strategy.
- Data challenges: AI models are only as good as the data you train them on, and one-fifth of respondents said their data quality was lacking. Another 20% were worried about insufficient data or had problems accessing the data.
- Responsible AI and governance: The survey also covered responsible AI practices and how respondents prioritized tasks. Security, privacy, compliance, and risk topped the group's to-do list.
With these obstacles in mind, what are your peers doing to overcome them and accelerate AI adoption?
Design a successful AI blueprint
The survey reveals several key strategies for AI’s integration into the business. Many are looking to adopt a ModelOps platform. This approach does not merely simplify AI initiative management and monitoring. It will also help you take on areas such as skill development, teamwork, and efficient resource use. Moreover, the findings encourage organizations to recognize the value of their existing infrastructure and complement it with targeted technological enhancements. Let’s dig deeper.
One AI platform to connect the dots
83% of respondents are in various stages of ModelOps platform adoption. Such a platform provides enablement, management, and monitoring of all AI activities. Of respondents working for companies with AI in production, 78% already use such a platform. Why did they choose this path?
- Upskilling and collaboration: Upskilling and collaboration are tightly related. A platform can help you easily share knowledge from existing work for reuse and training. Collaboration tools allow your data science expert to support and lead novice teams across projects. Furthermore, a platform can support upskilling with AutoML and coding assistants like Jupyter AI and GitHub Copilot.
- ROI and cost management: 83% of that group also invested already in AI-optimized computing. This hardware is in high demand inside any company. A platform can help you share access based on project urgency, budgets, or whether the hardware is just unused. That maximizes utilization and increases your ROI. You can also track usage and spending and back-charge individual business units for their work.
- Data — because AI is a data science: Many advanced organizations suffer from data and application sprawl. A platform can consolidate access to a single point. Enable projects to use multiple data sources with a centralized credential store. You can also set up a feature store, which 75% of companies in production adopted. And the platform will even accelerate data processing work with compute clusters.
- Security and governance: With a platform, you never need to worry about data lost on missing laptops. You can control roles and permissions for data and models from a single pane of glass. A platform can log access, data changes, and model executions. Such logs help you create an audit trail for regulators. The platform can facilitate model review cycles across teams. It can monitor your models for you, so if something goes wrong, you receive an alert. You prevent a problem from spiraling out of control and get to keep your job, too.
A ModelOps platform gives you control over processes, security, and infrastructure. It is a critical foundation for building AI capability across the company, and you should include it in your AI budget plan.
What about infrastructure? The survey had valuable guidance here, too.
Your infrastructure is good enough for AI.
AI is all the rage in the media. Hardware and cloud vendors enjoy the buzz as companies fear missing out on promised benefits. You may instinctively think you need to buy new hardware and overhaul your infrastructure for AI. Yet, a good majority of survey respondents (61%) rejected that notion. They believe their existing capabilities will provide a solid foundation for AI. They do, however, look to add AI-specific technology to that foundation. This approach is especially relevant if you invested in an on-premises data center. But even if you have a virtual private cloud (VPC), you should start with the infrastructure you have and augment it.
Discover more
If you got here, you clearly want to read the report. But, before you go there, we hope you give Domino a close look. As the enterprise AI platform of choice for many of the world's largest companies, Domino helped them scale AI. To learn more about the benefits of enterprise AI – and why you need an AI platform – check out the BARC report.
As Domino's content lead, Yuval makes AI technology concepts more human-friendly. Throughout his career, Yuval worked with companies of all sizes and across industries. His unique perspective comes from holding roles ranging from software engineer and project manager to technology consultant, sales leader, and partner manager.