Data Ethics: Contesting Truth and Rearranging Power
Ann Spencer2019-08-06 | 12 min read
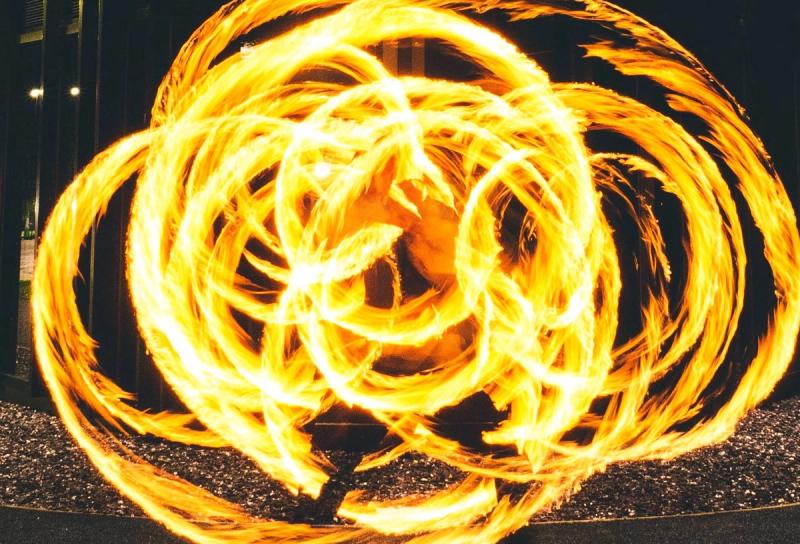
This Domino Data Science Field Note covers Chris Wiggins's recent data ethics seminar at Berkeley. The article focuses on 1) proposed frameworks for defining and designing for ethics and for understanding the forces that encourage industry to operationalize ethics, as well as 2) proposed ethical principles for data scientists to consider when developing data-empowered products. Many thanks to Chris for providing feedback on this post prior to publication and for the permission to excerpt his slides.
Data Scientists, Tradeoffs, and Data Ethics
As more companies become model-driven, data scientists are uniquely positioned to drive innovation and to help their companies remain economically strong. The IP, artifacts, and insights generated (i.e., the model context) when building and using models enable data scientists to directly impact business problems. Data scientists are continually making tradeoff decisions during the entire lifecycle of their models.
Recent questions about whether companies are taking data ethics into consideration have led to public discourse with contributions from prominent researchers (particularly with model interpretability), mainstream tech press, as well as data science leaders at established global institutions. Chris Wiggins, Chief Data Scientist at The New York Times as well as a professor at Columbia, recently presented the seminar “What should future statisticians, CEOs, and senators know about the history and ethics of data?” at Berkeley. In his talk, Chris proposed a framework, principles, and recommendations to help operationalize ethics in historical context. He also encouraged data scientists to understand how new data science algorithms rearrange power as well as how the history of data is a story of truth and power. The talk proposed that to understand the future, we need to understand how truth and power have always been contested. Even since the 19th century, when people collected data to establish capabilities, they often “use them to defend their own power rather than to empower the defenseless”.
I had just seen Chris speak in New York about his work building a team to develop and deploy machine learning in Data Science at The New York Times and I also attended his recent data ethics talk. While the data ethics presentation covered building and deploying a curriculum that paired technical and critical approaches to data, this blog post focuses on a proposed ethical framework, examples of modern data ethics problems (e.g., biases, harms, and re-identification), principles to consider, and recommendations. If interested in additional detail, the deck is available as well as a video of an earlier iteration of the talk. The class lecture notes and Jupyter notebooks are also distributed via GitHub. Many thanks to Chris for providing feedback on this post prior to publication as well as the permission to excerpt his slides.
Analytical Framework for Understanding Data Ethics: Data Science as Capability that Rearranges Power
Chris proposed that history provides a framework for “thinking about decisions to be made.” He recommended looking at prior problems, “historical dumpster fires,” or previously established “truths” that were contested. By reviewing how these truths were contested, history provides industry with a sense of “the different possible futures we may create.” For industry, the idea of inferring probabilistic outcomes is a familiar one. Yet, what may not be as familiar, is the argument that making a new data algorithm rearranges power. Chris argues that there is intent when building a capability and that only this capability --- not the intent -- becomes “portable to other people in the form of some technology. It has an impact on how people are able ‘to do what to whom’ which impacts the dynamics of power.” Chris indicated that he reached this epiphany because Ben Recht sent him the paper “The Moral Character of Cryptographic Work” by Philip Rogaway and recommended that Chris replace the word “cryptography” with “data science” when reading it. The paper’s abstract opens with “Cryptography rearranges power. It configures who can do what from what. This makes cryptography an inherently political tool”.
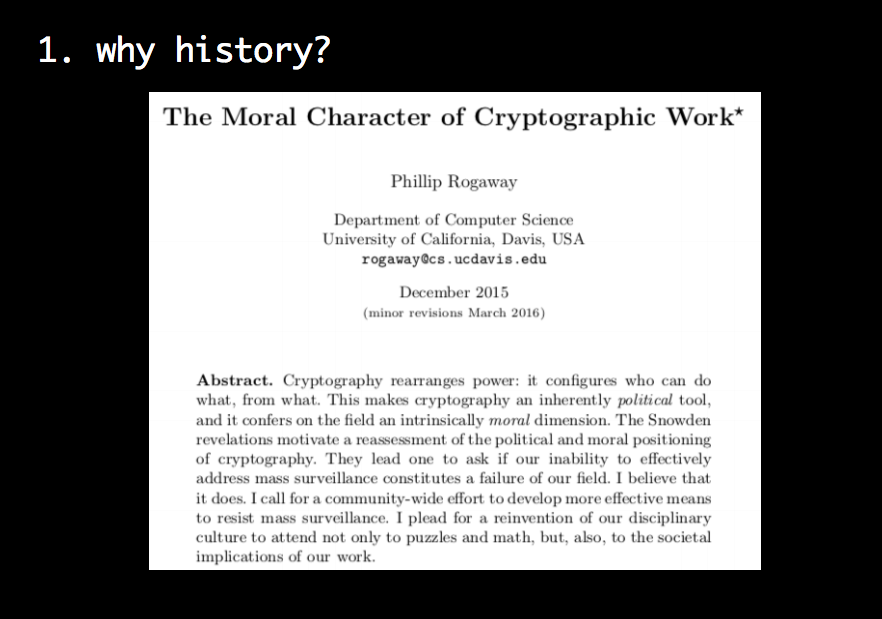
Then Chris advocated that data ethics should become a part of the public discourse, particularly when there are modern problematic implications including talent being reluctant to work for specific companies or when an engineer earns a prison sentence for his role in “defraud[ing] U.S. regulators and Volkswagen customers by implementing software specifically designed to cheat emissions tests.”
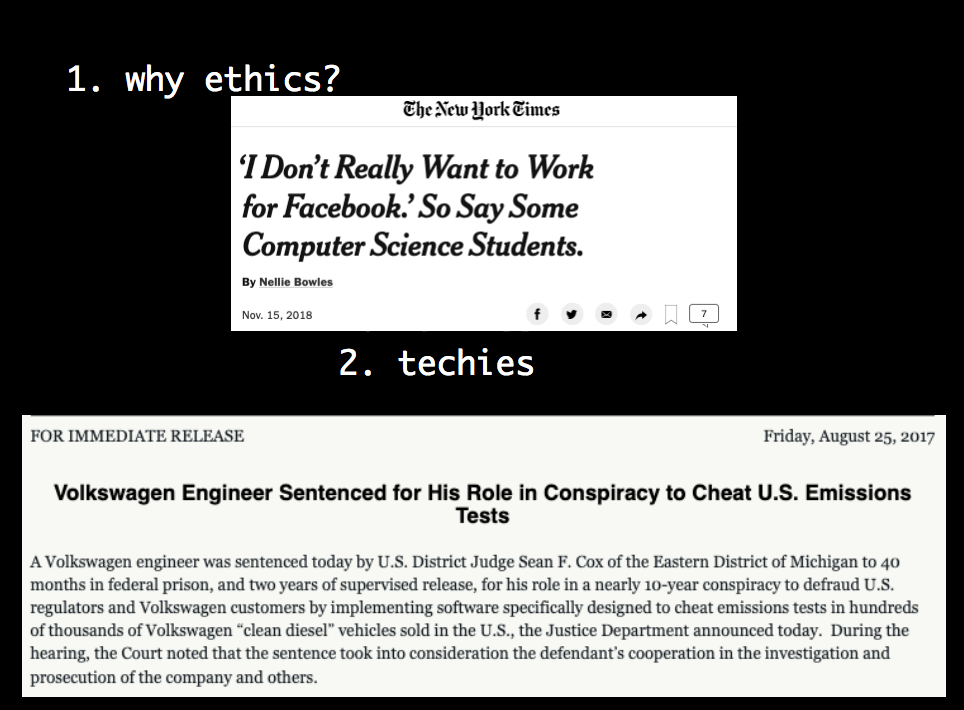
Chris encouraged discourse about various historical problems by asking people to answer the question “how did new capabilities rearrange power?” as well as consider the role of rights, harms, and justice.
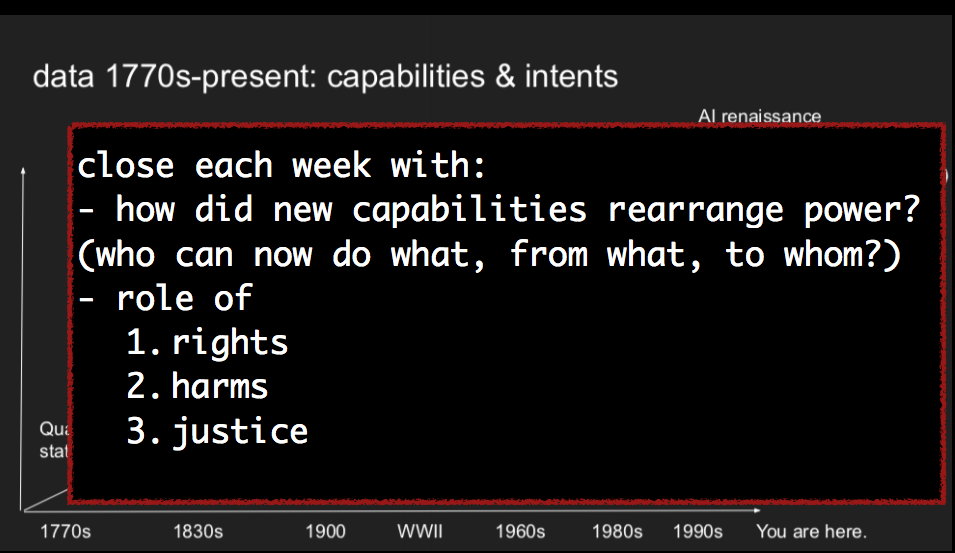
Chris also covered how recent modern literature was pointing to how “something was wrong on the internet”, with citations to analysis from Cathy O’Neil, Safiya Noble, Virgina Eubanks, and Shoshana Zuboff. Chris advocated that modern analysis from researchers contesting preconceived “truths” is influencing and shaping industry awareness about data ethics. (When an audience member noted “All women!” at the Berkeley talk regarding his citations, his response was “Exactly. And to that I could add papers by Zeynep Tufekci, Hanna Wallach, and many more. This is not a complete list”).
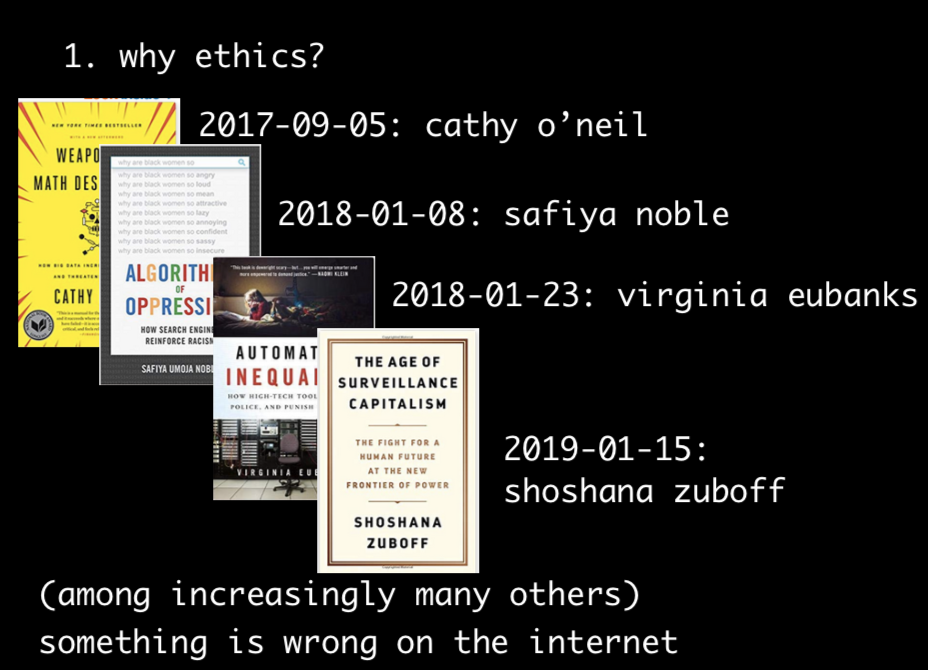
Data Ethics: Re-Identification and Contesting Truths (with Swagger)
Chris pointed out numerous examples of consumer protection protests in his seminar as well as how industry has contested and addressed them. Yet, there were two re-identification examples he covered in his talk that may especially resonate with data scientists, particularly when seeking out examples with business implications. In 2010, Netflix cancelled their second recommendation contest after a privacy lawsuit. The associated paper, “Robust De-anonymization of Large Sparse Datasets” by Avrind Narayanan and Vitaly Shmatikov
“demonstrate[s] that an adversary who knows only a little bit about an individual subscriber can easily identify this subscriber’s record in the dataset. Using the Internet Movie Database as the source of background knowledge, we successfully identified the Netflix records of known users, uncovering their apparent political preferences and other potentially sensitive information.”
An earlier re-identification example Chris covered was from Latanya Sweeney, in her paper “Only You, Your Doctor, and Many Others May Know”. Latanya pointed out that while one data set may be considered anonymous because a person's “name” is not included with demographic attributes, other data columns may exist somewhere with the same demographic attributes in columns paired with the person’s name. The similar columns become a join key and re-identification occurs. Chris also noted that Latanya emailed the governor of Massachusetts his own health records in 1997, when she was still a graduate student in CS at MIT!
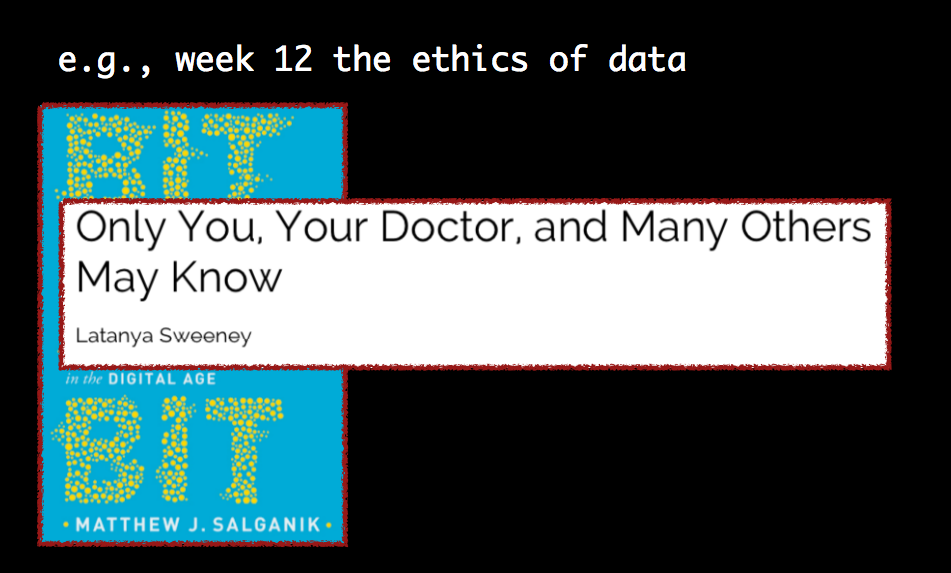
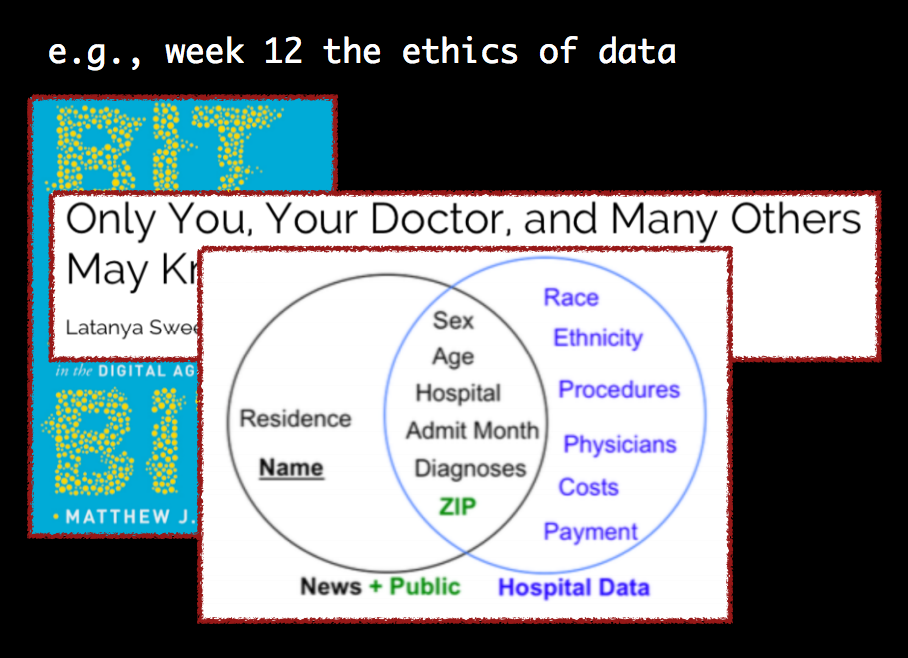
Data Ethics: Consider Adapting Belmont Principles
In his seminar, Chris noted that ethics is not a simple topic. Operationalizing data ethics, in particular, includes a union of technical, philosophical, and sociological components. This is not a surprise as data science requires critical literacies (e.g., awareness of subjective design choices) as well as functional technical literacy (e.g., data munging, building models, etc.). Also, data science work is experimental and probabilistic in nature. As a result, applying deterministic rules for ethics may challenge execution.
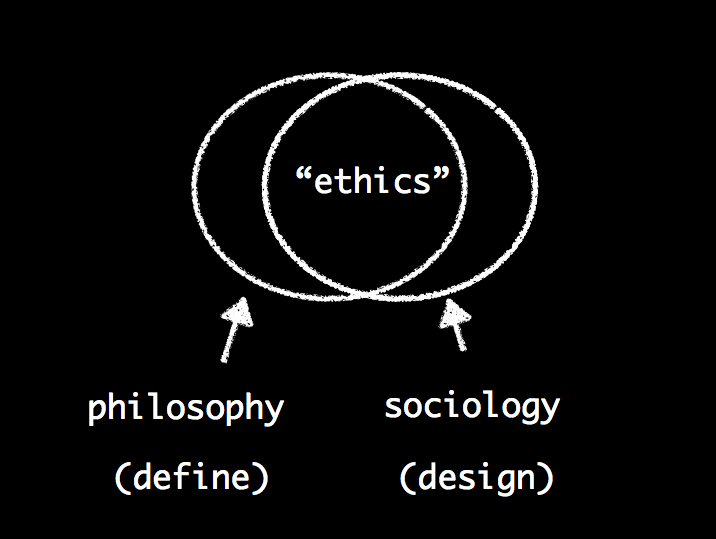
Chris recommended that industry consider articulating ethics as a set of principles with the understanding tension points that occur would be clearly communicated and considered. He then advocated designing a working system, adapted to new communities or companies, to operationalize these principles. Chris covered different sets of principles during his talk for people to consider. Yet, this post focuses on the Belmont principles.
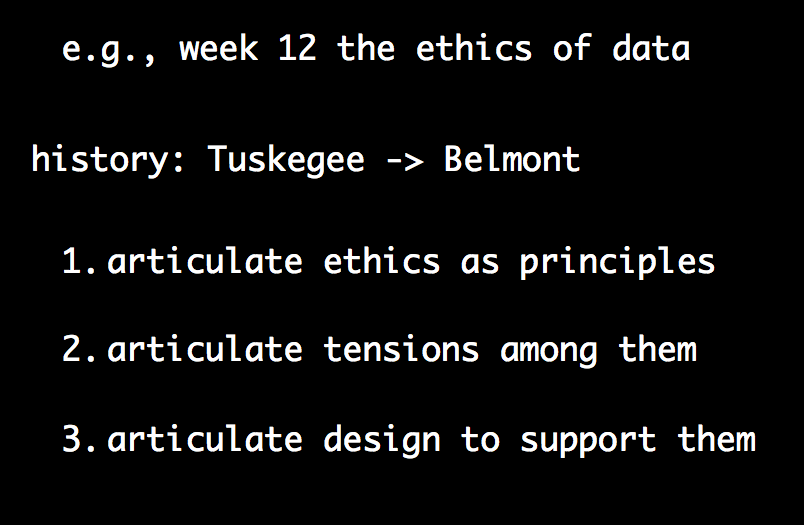
Chris drew from the Belmont principles that were developed in response to the Tuskegee Study. The Belmont principles are durable and “stress tested” as they have been around since the 70s. People continue to use them for interaction design and as a means to audit decisions (both before and after you make the decisions). Yet, Chris recommended updates based on how companies are using data and the current age we live in.
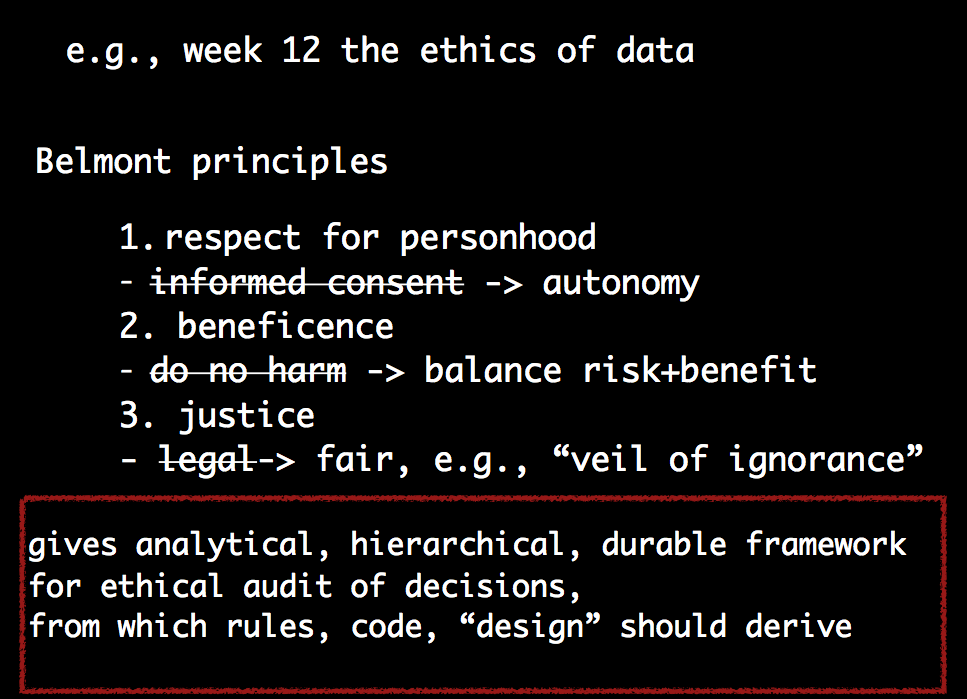
For instance, in the 70s there was emphasis on “informed consent” as a means to reach “respect for personhood.” Yet, today, Chris notes that
“informed consent is a murky concept, particularly when dealing with private companies, and everything they do with our data and all the ways we use their free services in exchange for free spying on us all of the time as Eben Moglen puts it”.
Chris recommends appending informed consent to “autonomy” or answering the question with an affirmative: “Are users given the chance to make autonomous decisions about how their data is used?” Chris also recommends reconsidering “do no harm”, maximizing the benefit since one “can do no harm by never talking to you and or engaging with you”. The third principle is “justice." Chris recommends replacing “legal” with “fair” as in “setting up a system such that you would be happy to be any of the participants, any of the stakeholders." For example, with a rideshare system, was the system set up where the passenger, the driver, and the company are all equally happy? Whether the answer is “yes” or “no,” it provides a framework to consider during the audit and when developing a machine learning algorithm.
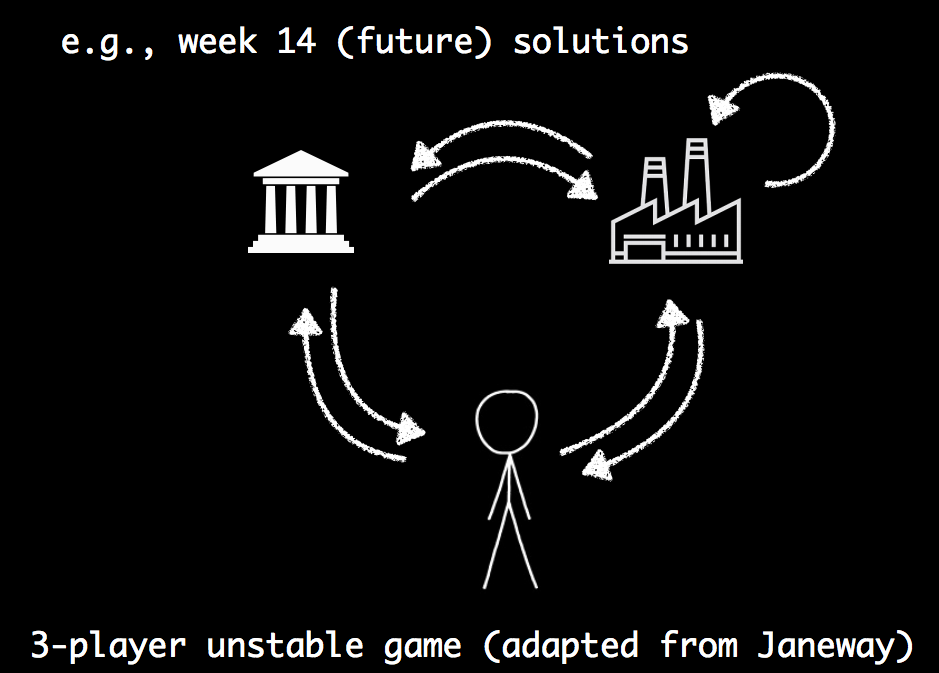
Checks and balances on corporate, state, and people power
Before a community -- including a company -- defines and designs for ethics, they must first come to realize it’s a good idea. Chris also proposed a framework for understanding the forces driving industry to consider ethics in the first place, describing the checks and balances as a “3-player unstable game” among state power, corporate power, and people power. He briefly reviewed current ways that state power is re-engaging around consumer protection and antitrust regulation, and how people power is emerging in the form of collective agency among employees including data scientists and software engineers.
Summary
Chris covered a broad scope of topics related to data ethics in his presentation, “What should future statisticians, CEOs, and senators know about the history and ethics of data?” While this post provided distilled excerpts from his seminar including a proposed framework, specific examples of industry contesting truths, and recommended principles for data scientists to consider, more insights are available in the deck or the video of an earlier iteration of his talk. Again, many thanks to Chris for his permission to excerpt his slides and for his feedback on this post prior to publication.
Ann Spencer is the former Head of Content for Domino where she provided a high degree of value, density, and analytical rigor that sparks respectful candid public discourse from multiple perspectives, discourse that’s anchored in the intention of helping accelerate data science work. Previously, she was the data editor at O’Reilly, focusing on data science and data engineering.