Data Science for the Birds (and Everyone)
Chad Wilsey2020-02-21 | 10 min read
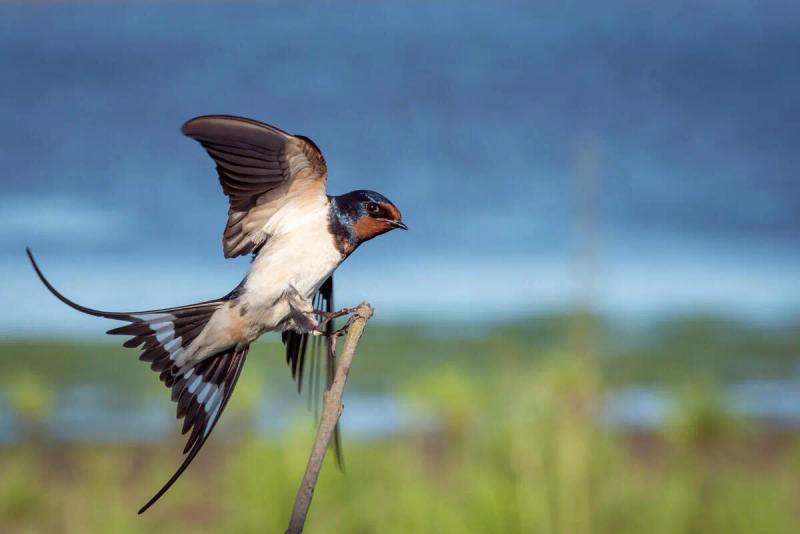
How Audubon’s data science work is making an impact
Editor’s note: This is part of a series of articles sharing best practices from companies on the road to become model-driven. Some articles will include information about their use of Domino.
I am a scientist by training. I have a Ph.D. in ecology, a master’s degree in conservation biology, and an undergraduate degree in biology. Today, I am interim chief scientist for a non-profit that’s been around for over 100 years: The National Audubon Society.
You’ve probably heard of us. We’re focused on bird conservation across the Americas. Why birds? Ecologists have long observed that people thrive where birds thrive. So if we can protect the birds, we can help better protect the earth for all of us.
In the past six years, we’ve grown our data science practice significantly. We want to understand the effects of climate change on bird populations and inform our conservation and advocacy efforts. Like most organizations, we’ve experienced a dramatic increase in the volume of data captured through the use of mobile phones and web services. These technologies have expanded what we call “community science,” where volunteers can share sightings and other relevant data. We’ve constructed 180,000 models that analyzed more than 140 million observations from scientists and community members, and predicted the impact of different warming scenarios on 604 bird species. We then built the Birds and Climate Visualizer, a zip code-based online tool that allows anyone to see the impacts of climate change in their community.
On the surface, building a data science team at Audubon may seem like a different animal than building one in the for-profit world. Many might assume that it would be easy to scale data science here, since, after all, our organization was founded on science and data. But in reality, we face many of the same cultural and organizational challenges that companies across industries face in scaling their work across their organization. Audubon has “business units,” focused on different priorities, such as coasts, working lands or climate. Then, there are state field offices that work on more local conservation issues. As I mentioned, we’re a more than 100-year-old science-based organization. There was a lot of great quantitative work already going on. We had to show how machine learning models would add value to what we were already doing. Also, we had to transition from a science team that operated in isolation to one that was integrated with business units addressing their problems.
To break down silos, streamline processes, and retain talent, our science team operates like a Data Science Center of Excellence (CoE). In this blog, I’ll discuss our journey to build a robust science team and the steps we’ve taken to ensure we have the right skills, processes, and technologies in place to incorporate a model-driven approach to bird conservation efforts.
Where did we start?
At a recent Data Science Pop-up, Domino Chief Data Scientist Josh Poduska and representatives from Slalom Consulting talked about defining a CoE’s capabilities. Our science team today addresses many of the same elements they described.
We’re midstream in our work, but our efforts have already had a significant impact in terms of output and end user adoption.
Here are five steps we took as we created a science team that aligns with building a functional Center of Excellence:
- Shaped our efforts around our "customer," to align all data science work against organizational goals. We spent a great deal of time meeting with leaders across Audubon conservation strategies to discuss each group's priorities and demonstrate a clear connection with our work. That was a critical first step and involved a great deal of cultural change and communication. The strong relationships and successes we built led to more opportunities. For example, we worked to develop a model for one of our state offices—it was the first data science project of its kind with a state office. Word of the project's success spread, and opportunities began to snowball with other state leaders wanting to partner with us.
- Clarified the capabilities we could provide. We also identified what types of data science would provide the most value to the organization. We now, as a result, focus on three key areas:
- Predictive modeling to help groups define a problem or threat that can impact birds, anticipate future challenges, and identify policies to mitigate those threats successfully.
- Trending to show where bird populations have been declining and where they have been increasing so teams can measure the success of their initiatives.
- Spatial optimization that looks across data and to help our organization find the optimal places to do conservation work.
- Developed core values to guide talent management. We've grown from a team of two to 16 people doing data science. To help attract and retain top talent and ensure we have the skills as we scale our work, we developed core values to guide our team. Of course, we looked for strong data science skills to create publication-quality science. But beyond that, our values emphasized soft skills, such as humility, empathy, and patience, which are crucial to gaining end user buy-in for our work. We also included a growth mindset in our core values to ensure that, as a team, we are continually learning and applying new skills. Having a growth mindset helps us innovate more and increases job satisfaction for our data scientists. We have a high retention rate, and I believe that giving people a chance to learn and grow in their positions is key to that.
- Invested in technology infrastructure, tools, and data partnerships that facilitate data science at scale. For example, we evaluated different data sets that would help identify the places most important to conservation. We now draw data from a number of organizations that collect bird sightings, such as eBird, iNaturalist, and Movebank. We still have data deficiencies, but we have processes to help us identify new data sources to fill the gaps. We also want to make sure our data science team is as productive as possible. This led us to the Domino data science platform, which allows us to collaborate, iterate, and explore different parameter settings much faster. We built 160 machine learning models for each of the 604 species, covering two different seasons--winter and summer--for a total of over 180,000 models in just a few months. Having the ability to reproduce and build on results has had a considerable impact on our output. Most recently, we’ve begun reevaluating our technology infrastructure. We have a lot of data in CSV files and a variety of operational systems. A data warehouse would allow us to deploy data services to end users more efficiently.
- Identified success metrics to show our impact. In the for-profit world, CoEs typically identify key performance indicators (KPIs) for cost savings or revenue increases to measure the success of their data science efforts. Here, we do the same, but instead of cost and revenue, we talk about habitats. Specifically, how much habitat have we protected and how many birds we have saved?
What does the data tell us?
Today, climate change stands as the biggest threat to bird populations. We've been able to show through our work how bird ranges would change under different warming scenarios. Would a species expand into a new area if their existing range was no longer habitable, or would they face extinction instead? The most vulnerable species are ones that face significant loss of their current range and are not likely to move to new areas. The prognosis isn't good. According to our latest report on birds and climate change, Survival by Degrees, nearly two-thirds of North American birds are at risk of extinction if we experience a 3 degree Celsius increase. But with the deep insight we've gained in recent years, we can also now see the impact of potential policy changes: immediate and aggressive action to keep global temperatures down to a 1.5 degree Celsius increase can improve the chances for 76 percent of those species at risk. Our data science work will continue to contribute valuable insight to this conversation and help mobilize our nearly one million members and supporters to take action. And as we build our science team under the model of a CoE, we're confident that our reach as an organization will grow.
For more information
- Case study on the National Audubon Society
- Press Release: New Audubon Science: Two-Thirds of North American Birds at Risk of Extinction Due to Climate Change
- Birds and Climate Visualizer
- Climate Issue of Audubon Magazine
RELATED TAGS