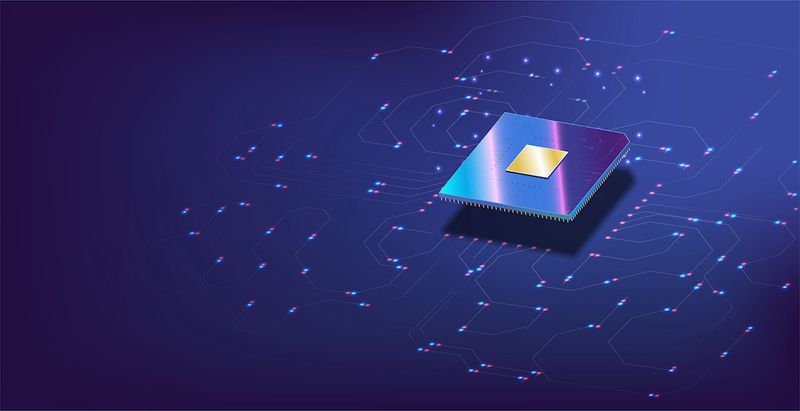
When it comes to scaling your AI capabilities, you need more graphics processing units (GPUs). They’re the fastest and most cost-effective way to train your deep learning models that power your AI applications. The parallel processing power of GPUs boosts performance for AI use cases ranging from natural language understanding (NLU) – such as speech recognition, text analytics, and virtual agents – to computer vision – such as defect detection, object recognition, and facial analysis. Indeed, they are critical for nearly every AI application built on unstructured and semi-structured data.
With GPUs you can develop more accurate deep learning models – faster – for new, innovative AI applications. They help your data scientists deliver better business outcomes, leverage the latest AI innovations, and spend less time waiting in frustration for model training jobs to complete. However, to leverage GPUs effectively and at scale, you need a GPU strategy. This blog post explains why and lays out the five key elements your GPU strategy should address.
Providing GPU Infrastructure at Enterprise Scale Has Been Difficult & Expensive
While it is trivial to spin up a cloud GPU instance and deliver proof-of-concept deep learning projects, nearly all enterprises struggle to provide even the modest GPU capabilities their data science teams need today, let alone what they’ll need in the near future. Few data scientists have access to GPU clusters, and those that do are bogged down in time-consuming and error-prone manual work.
Companies balk at the usurious fees cloud vendors charge for GPU instances and for transferring the large data volumes needed to and from the cloud, yet they have few, if any, individuals with the rare, expensive talents to build and maintain GPU clusters on-premises. Worse, the cost-and-management headache is set to grow, as AI applications proliferate, edge computing takes off, and unstructured data volumes grow exponentially.
Five Pillars of an Effective Enterprise GPU Strategy
If this story sounds familiar, that’s because it is. The challenge of providing GPU infrastructure for data scientists is similar to the historical challenge of providing CPU infrastructure for application developers, only harder. AI workloads are growing faster, are even more irregular than typical applications, and involve a new hardware and software stack.
Further, that stack is expanding and enterprises need to support a growing number of machine-learning libraries and distributed computational frameworks to meet the needs of their data scientists. Enterprises can solve their existing challenges, and get ahead of their future ones but, much like AI itself, it won’t happen automagically. You need a GPU strategy to take advantage of the GPU opportunity. Here are the key elements your strategy should address:
- Leadership. All too frequently, GPU capabilities emerge created by individual data science teams, often without IT support, either because they’ve been driven by the desire to experiment or because they have had no choice if they want to get their work done. However, this is a recipe for creating siloed GPU capabilities, that are available to a fraction of the users that need them and that are wasteful in terms of cost and effort. To build scalable GPU capabilities, a leader must be given the people, the budget, and the responsibility for creating, delivering, and continuously upgrading these capabilities. They must also be rewarded and held accountable for the results.
- Hybrid capabilities. Both on-prem and cloud GPUs have their tradeoffs. On-prem GPUs are cheaper but inflexible, while cloud GPUs can scale up and down to meet the highly variable volume of AI model training jobs. While it has been straightforward to access cloud GPUs, it is now easier for enterprises to deploy and manage their own on-prem GPU clusters as well, thanks to new converged infrastructure solutions such as the NVIDIA DGX systems. Sooner or later, every enterprise needs both on-prem and cloud GPUs, and will need to implement an integrated platform that dynamically supports workloads on both.
- Automation and self service. AI workloads are lumpy, with projects that start and progress in fits and starts. Further, the capacity and environments that users need – the languages, libraries and frameworks – can vary dramatically from project to project. It is enough to drive crazy both whoever is administering the cluster and users waiting for their environment to be provisioned. The solution lies in platforms (like Domino Data Lab) that automate provisioning and deprovisioning of environments and democratize access on demand.
- Governance and reproducibility. Your administrators need governance tools to manage, optimize the use of your GPU infrastructure, and prevent users from unintentionally bringing down the cluster, or incurring runaway costs. Even more important in the long run is to implement solutions that ensure your models are reproducible long after they are deployed, by capturing all of the details of the environment, data, code and libraries used. This information is critical not just for regulators and customer trust, but for diagnosing problems and continuous improvement.
- An open, futureproof architecture. Nothing is certain in the probabilistic world of AI, except that tomorrow’s frameworks, libraries and hardware will be different from today’s. Indeed, instead of consolidation, the trend is in the opposite direction of proliferation. You should try and standardize your GPU toolsets where possible, but your GPU strategy will need a plan to support an already wide array of AI tools, and will need to be open, modular and flexible enough to swap in new components as they are developed.
Speed Matters, for Model Training & Competitive Advantage
For every organization planning to transform itself with data science and AI, it is not a question of “if” your organization will need to rapidly grow its GPU capabilities, it is a question of “when.” For companies that are further along in their use of AI, that “when” has already come and gone. These companies are either implementing strategies to provide both the GPU hardware and software their data science teams need, or they’re finding their AI ambitions curtailed.
Fortunately, there are now offerings that provide the orchestration, automation, and even self-service capabilities necessary to leverage GPUs in hybrid environments – such as the new collaboration between Domino Data Lab and NVIDIA. However, to use them effectively and to ensure that you build a futureproof foundation for your GPU needs now and into the future, you need to get cracking on your GPU strategy.
For more information on scaling AI with MLOps and GPU platforms:
- Download Operationalize AI at Scale with MLOps to learn about key considerations for purpose-built AI infrastructure and MLOps platforms.
- Read about Domino and NVIDIA’s Spring 2022 GTC announcements, including new support for NVIDIA Fleet Command, NVIDIA NGC, and LaunchPad curated lab.
- See Domino’s sessions at NVIDIA Spring 2022 GTC conference.
Kjell Carlsson is the head of AI strategy at Domino Data Lab where he advises organizations on scaling impact with AI technologies. Previously, he covered AI, ML, and data science as a Principal Analyst at Forrester Research. He has written dozens of reports on AI topics ranging from computer vision, MLOps, AutoML, and conversation intelligence to augmented intelligence, next-generation AI technologies, and data science best practices. He has spoken in countless keynotes, panels, and webinars, and is frequently quoted in the media. Dr. Carlsson is also the host of the Data Science Leaders podcast and received his Ph.D. from Harvard University.
RELATED TAGS
Subscribe to the Domino Newsletter
Receive data science tips and tutorials from leading Data Science leaders, right to your inbox.
By submitting this form you agree to receive communications from Domino related to products and services in accordance with Domino's privacy policy and may opt-out at anytime.