Improving the partnership between Data Science and IT
Karina Babcock2020-02-07 | 8 min read
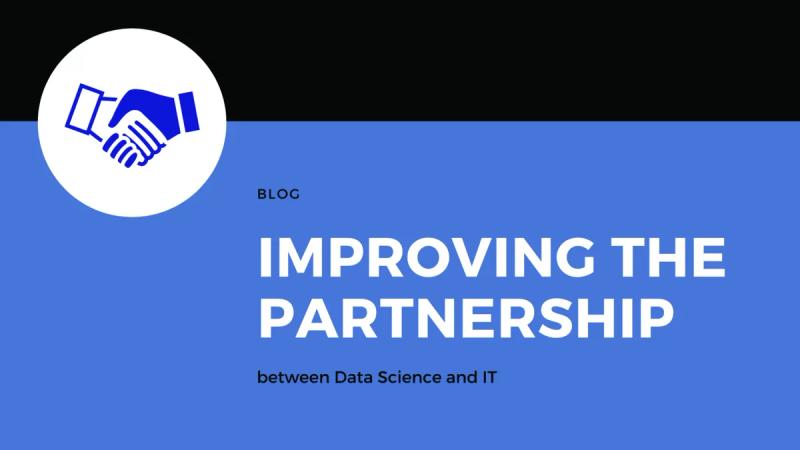
Editor’s note: This is part of a series of articles sharing best practices from companies on the road to become model-driven. Some articles will include information about their use of Domino.
The relationship between Data Science and IT can be complicated. While the end goal may be the same—to help the business win through model-driven innovation—there’s often tension on how to get there.
On one hand, data scientists drive innovation by building models that automate or inform business processes. To do their jobs well, they need an open environment that embraces cutting-edge tools, scalable compute, and exploration.
On the other hand, IT provides and manages the technology landscape that makes it all possible. They are responsible for ensuring platforms are safe, governed, scalable, compliant, and cost-effective. And they serve many internal customers, not just Data Science.
Friction can quickly arise as a result of these separate workflows and priorities. Given their differences, how can data science and IT more seamlessly work together in building a model-driven organization?
In recent months, data science leaders have shared their experiences in tackling this challenge at Domino Data Science Pop-ups and other events. In this blog, we share some of their strategies which include making data science real and relevant, recognizing and addressing the concerns and constraints IT faces, and actively preparing for model “handoff” together.
Make data science real and relevant
An ongoing challenge for data science teams is often getting IT to allocate resources to implement and monitor new models. To combat this, Matt Cornett, formerly senior director of Data Operations and Tools at the Gap, includes the business context of a project with any resource request, sharing how models will integrate into existing workflows and deliver value to customers. “Instead of leaving it abstract,” said Cornett, “I like to connect it to a business result and a customer experience.”
At Vevo, the video hosting service, Director of Data Science Eyal Golshani makes a point to identify use cases that solve an IT problem in particular. It’s a great way, he says, to demonstrate the value of data science and necessary processes. “Once IT sees what the process looks like and how it can help them achieve their goals, they become more bought in, more willing to help out and evangelize the process,” Golshani explained.
Recognize IT concerns and constraints
What keeps your IT teams up at night? According to Cornett, understanding “where IT may be coming from” helps his team get ahead of potential issues and build a more integrated effort between data science and IT. For example, it’s easy for IT teams to want to lock down data to protect customer information and privacy. Addressing data privacy and security concerns at the outset of any project can help clear the path a bit, especially when data scientists seek access to new data types.
Cornett also advises looking for ways to relieve IT of costly, time-consuming activities. For example, Cornett’s team often creates a prototype of a new platform, such as a data mart, before asking IT to build it. “It’s an opportunity to help IT reduce costs and keep moving projects forward,” said Cornett. “Rather than asking IT to spend six months gathering requirements, our team can use that time to iterate and then turn over a clean set of requirements for IT to build.”
Additionally, using Domino, Cornett has seen data science teams move models into production within minutes rather than waiting months and spending tens of thousands of dollars recreating models to run on production systems.
Similarly, Trupanion’s Director of Data Science, David Jaw, shared in a recent interview with Domino how his team uses the Domino data science platform to free IT from activities like provisioning resources or creating APIs, which has improved collaboration. “Without this capability, we would have constantly been going back and forth with IT,” Jaw said. “Instead, we can seamlessly push forward together on our shared mission.”
Prepare for the handoff together
As models transition from innovative lab experiments to real-world products, data science and IT teams must coordinate on a host of activities, from how to integrate models into downstream systems to monitoring for model drift. Lee Davidson, who leads Morningstar’s Quant Research team and the company’s Head of Technology and Product Analytics, Jeff Hirsch, work closely to ensure their teams “integrate early and often” to productionalize new models. Here are a few steps they take:
- Outline project stages, touchpoints, and ways of working together from the outset. At Morningstar, the teams track projects against five stages:
- Exploration
- Active research
- Model development
- Productionize
- Launch and maintain
The Quant team regularly updates IT as projects move through each stage to keep them apprised of progress. At the development phase, when active IT support is needed, the Quant team shifts from its more open-ended, research-oriented processes to an agile approach used by the IT team.
- Sketch out how the handoff will work. For example, will researchers be responsible for delivering production-level and thoroughly tested code, or will they only need to provide a prototype? What processes will be required to help those who didn’t build a model maintain model performance over time? “Several years ago, we did this mostly by the seat of our pants, and there were some challenges and pressure as a result,” said Davidson. “We’re getting more clarity on these now.”
- Create a checklist of activities required during the handoff, such as performing QA testing or sharing Jupyter notebooks, and ensuring staff members have the skills to work closely together. “There’s a gray area where the handoff happens,” Hirsch said. “Both teams need to move more toward the middle: The IT org needs to ensure its QA staff can interpret the results of Jupyter notebooks, for example, while data scientists need to learn some of the skills of IT engineers.”
There are a lot of moving parts on the journey to becoming model-driven. As these data science and technology leaders are finding, taking time to improve communications, discuss challenges, and clarify responsibilities can pay off handsomely in reducing friction and helping them more rapidly and efficiently achieve their goals.
Learn more
- Listen to Morningstar’s panel at the Chicago Domino Data Science Pop-up to learn more ways Data Science and IT can partner.
- Listen to Matt Cornett and Eyal Golshani speak at the Domino Data Science Pop-up in San Francisco to hear their full presentation on partnering with IT.
- Read David Jaw’s blog on Trupanion’s work to become model driven or view hispresentation, Auto-adjudicating Veterinary Claims at Trupanion, originally given at the Rev 2 Data Science Leaders Summit in May 2019.
RELATED TAGS