Can financial institutions mitigate model risks without stalling innovation?
Jarrod Vawdrey2024-11-12 | 7 min read
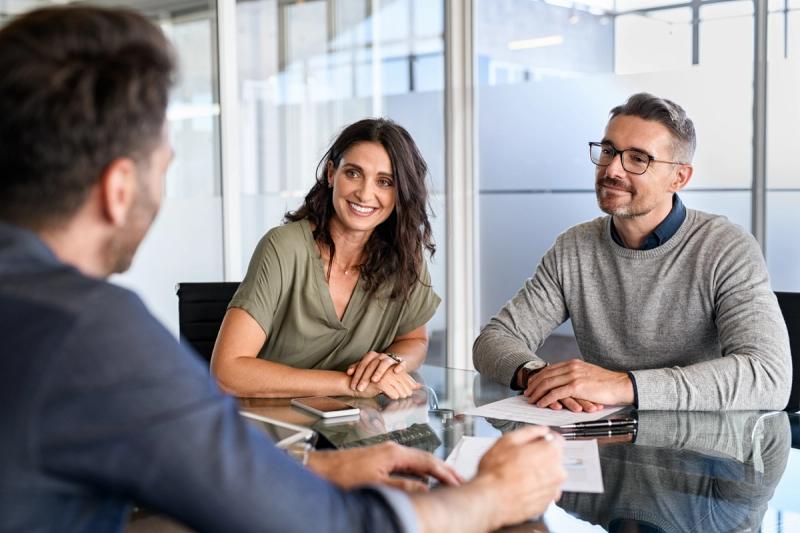
In an era where AI technology is evolving at breakneck speed, traditional risk and governance management systems are struggling to keep pace. Models and data are increasingly complex, and new regulations are emerging continuously. This rapid evolution presents significant challenges: the risk of making poor, indefensible decisions based on degraded, non-performant, irrelevant, or biased data and models. While AI-driven decision-making holds transformative potential, its downside could be devastating without proper governance guardrails in place.
Model risk management (MRM) is a crucial discipline within financial institutions. It provides a structured framework of processes and controls to govern the lifecycle of quantitative AI and data science models. By design, MRM also ensures model accuracy, reliability, and appropriate use. MRM capabilities include maintaining the integrity of model development and implementation processes, ongoing model validation, performance monitoring, and aligning model risk with an institution’s overall risk appetite. This comprehensive approach covers a range of use cases, from credit scoring and loss forecasting to regulatory reporting and asset valuation. Explore more insights by reading this MRM white paper.
MRM matters now more than ever
First, regulators are increasingly focused on the challenges posed by AI and machine learning, recognizing the vital role of models in financial decision-making. For example, in the United States alone, agencies like the Federal Reserve, OCC, SEC, and FDIC have issued guidelines forming the backbone of MRM practices. These regulations ensure that financial institutions effectively manage model risk and maintain robust governance practices.
In addition, several high-profile failures to manage model risks underscore the critical need for robust MRM:
- 2008-2009 Global Financial Crisis: Complex models used for mortgage-backed securities misjudged risk, leading to a market meltdown exceeding $20 trillion. This disaster highlighted the systemic risks of poorly validated models and prompted stricter regulations.
- “Power Key” Trading Error: A coding error led to a global firm incurring a $440 million loss due to a flood of erroneous orders. This incident demonstrates the severe financial consequences of model implementation errors.
- The "London Whale" Incident: A U.S. bank’s misuse of a risk-hedging tool resulted in billions in losses due to inadequate model governance and validation, showcasing the importance of comprehensive risk assessment beyond mere parameter adjustments.
MRM systems cannot be an afterthought — any modern MRM solution needs to keep pace with current and future regulations, simplify compliance documentation, and mitigate a wide variety of risks in an automated, comprehensive way.
Why is MRM so hard to pull off?
AI can be a “black box”
AI/ML models often operate as "black boxes," making their decision-making processes opaque. This complexity challenges risk management teams to adapt MRM practices without stifling innovation. Ensuring transparency in model decisions is essential for regulatory compliance and customer trust.
Data quality matters
Advanced models require vast amounts of data. Ensuring data quality is crucial to prevent biased or unfair outcomes. For example, a major tech company's image classification model misidentified dark-skinned individuals as "gorillas," a problem that could lead to unfair financial practices if not addressed.
More models, more risks
Financial institutions, like JPMorgan Chase with over 1,000 models in use, face challenges in managing the interactions and dependencies among these models. Even minor inaccuracies can have significant downstream effects, requiring meticulous oversight and monitoring.
Model monitoring never stops
The dynamic nature of financial markets necessitates real-time monitoring of model performance. Advanced techniques like multi-armed bandit algorithms for dynamic pricing require sophisticated monitoring systems to ensure models stay within acceptable risk parameters.
Modernizing MRM compliance with Domino
Domino's Enterprise AI Platform provides a comprehensive ecosystem for end-to-end model development, integrating MRM capabilities seamlessly. Domino’s governance solution ensures that models are auditable and governable throughout their lifecycle, balancing innovation with regulatory compliance. Domino Governance addresses all MRM requirements, with:
- Model inventory and lifecycle management: Centralized repository for tracking model lineage and dependencies.
- Global system of record: Ensures consistency and comprehensive record-keeping.
- Automated evidence generation: Simplifies compliance and auditing with automated documentation.
- Policy adherence and risk minimization: Templates and checklists ensure consistent risk management practices.
- Integrated model monitoring: Provides a unified view for observing model health and performance.
By leveraging Domino’s platform, financial institutions can manage model risk effectively while embracing advanced analytics and machine learning. Learn more about AI governance with Domino’s model risk management solution.
Conclusion
As financial institutions increasingly rely on complex models, robust model risk management becomes imperative for trusting, replicating and justifying decision-making to regulators, employees and customers. Domino’s solutions streamline regulatory reporting, enhances transparency, and supports safe innovation. By integrating advanced MRM capabilities, institutions can confidently navigate the complexities of AI and machine learning, ensuring they harness the technologies’ full potential while holistically managing associated risks.
Explore more ways to enhance your MRM practices with a modern approach to risk management and read the latest MRM white paper. For additional insights, join the upcoming webinar on how to modernize model risk management — without stalling innovation.
Jarrod Vawdrey is a fixture in the data science community, and is Advisor and former CEO of A42 Labs, a leading provider of AI and ML software and services for building, deploying and managing business critical data workloads at scale. Jarrod holds multiple patents in the field of AI and data science.