Want to Make Your Data Scientists Un-poachable? Consider These Top Expert Tips
Lisa Stapleton2022-04-20 | 8 min read
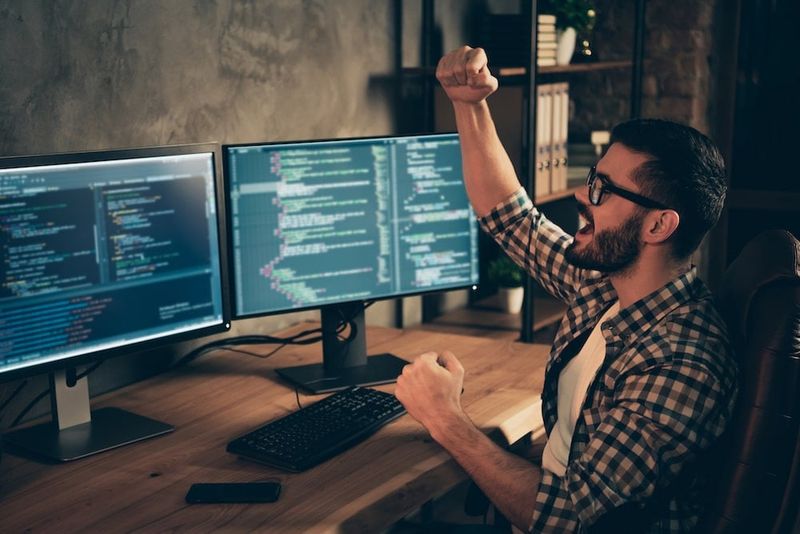
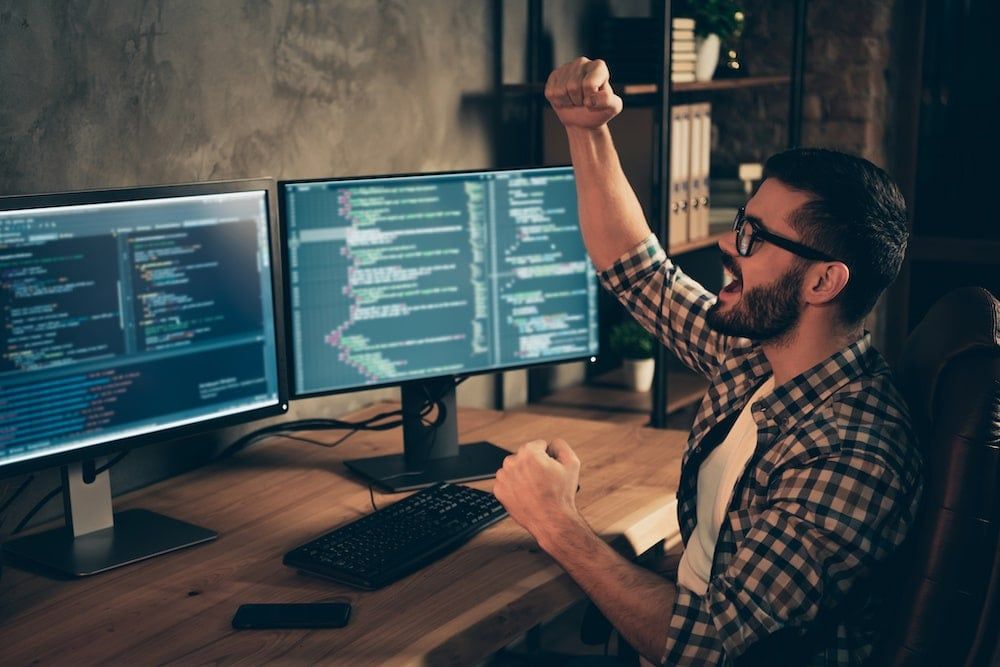
By Lisa Stapleton, Director of Content at Domino, on April 20, 2022 in Perspective
If you’re trying to use data for competitive advantage, the idea of high turnover of your data scientists in the wake of “The Great Resignation” is no doubt a sleep-killer. Data scientists were already in high demand and commanding high salaries, and the Burtch Works Report says the field is likely to experience high hiring rates in 2022. It's quite probable that this intense competition for data science talent could persist for quite a while. But some of the factors that data scientists and their managers contend with are surprising, and a few of the “fixes” to these problems are shockingly inexpensive to institute, according to a roundtable of experts on getting the most from data scientists.
“Of course, data scientists want to be paid—it’s a hot market, and there’s a lot of competition for their skills—but you can do a lot of non-monetary things to make them happy and satisfied in their work,” says John K. Thompson, global head of advanced analytics and AI at CSL Behring, one of the world’s largest biopharmaceutical companies. “If you give them the freedom to innovate, the freedom to experiment, the freedom to sometimes fail. Make it a place where they want to come and experiment, they’ll be almost un-poachable,” he says.
Thompson has also written extensively on how to lead data science teams effectively, and authored Building Analytics Teams: Harnessing Analytics and Artificial Intelligence for Business Improvement. He also served as one of the experts on a roundtable discussion led by Harvard Business Review contributor and management thought leader Tom Davenport, which looked at what really matters to data science leaders and to the effectiveness of their teams.
The Data Science Environment Counts
The ability of data scientists to use the tools most familiar to them is critical to the job satisfaction of many data scientists, a fact that is often a woefully under-appreciated element of their job satisfaction.
“Data science is more of an art than a science at this point, and you want to enable these artists to be as productive as they can be,” says Thompson. The trick, he says, is to “treat them like the artisans that they really are.”
“Almost all the data scientists in my shop have their own environment set up and customized so they can work the way they can be most creative and innovative,” he adds. “So if they say they like R, we provide them with a great R environment. If it’s Python, we set them up with all the Python tools they want. We don’t require them to standardize—we give them what they need to be creative, innovative and have the highest ability to be creative.”
MLOps Tech Helps Keep Data Scientists Happy
Luckily, the ability to let individuals within a team choose the tools they know—to achieve their highest productivity—is now a more manageable proposition, due to tools-agnostic MLOps technology such as Domino Data Lab’s. A radical departure from the days when every data scientist had to use the exact same tools as the rest of the team or organization, MLOps “marries and automates ML model development and operations, aiming to accelerate the entire model life cycle process,” as a recent Deloitte report puts it. And, when done right, MLOps gives data scientists the freedom to choose their environments.
Thompson isn’t alone in understanding the connection between an easy-to-use, free-to-choose tailored modeling environment, and encouraging data scientists to do their best, most creative work. That’s something that’s important both to data scientists themselves and to their leaders, as Michael Eiden, global head of AI and Machine Learning at Arthur D. Little (ADL), explains.
"We wanted a data science platform for data science professionals who are at the leading edge of their discipline. Other platforms were too simple for hardcore data scientists. Our data scientists feel right at home on the Domino [Enterprise MLOps] platform and appreciate how much freedom they have in experimenting. They are not constrained by CPU, hardware limitations, or tooling. And the more flexibility they have, the more creative they can be."
Jim Swanson, Johnson & Johnson’s Enterprise CIO, understands the value in empowering data scientists to use whichever tools make each individual most productive. Enterprise MLOps can be a powerful enabler of creativity. “We see this as an enabler for very smart people,” says Swanson in this video.
A Surprisingly Common Roadblock to a More Data-Driven Business
Developing a more collaborative environment is also critical. Data science is increasingly a collaboration not just within teams of data scientists themselves; rather, it's often a cross-departmental effort that is at times somewhat fraught.
For example, the relationship between data scientists and IT is surprisingly problematic. A recent DataIQ survey found that about one-third (33.7%) of the organizations they surveyed see 'conflict between data science and IT’ as one of their biggest challenges. Even companies that rate themselves as ‘Advanced’ or ‘Reaching Maturity’ in terms of their level of adoption of data and analytics are not immune to conflict. For both of these groups, ‘conflict between data science and IT’ is their biggest challenge (52.4% and 50% respectively).
“‘Conflict between data science and IT manifests itself in many ways, and it often starts because IT treats data science models like they do other software projects,” says Thompson. Part of easing conflict comes from analyzing the different pressures and requirements placed on IT and data science departments. IT pros don’t always understand how different modeling can be from software development.
“Models require re-training, are developed in an experimental fashion, and are made using many different software tools. There is no need to ‘retrain’ software code, but [data science] production models need to be retrained frequently,” he says.
Part of the remedy for the situation might be the realization that data science—and data scientists—don’t fit neatly into existing structures. For that reason, it’s necessary to rethink a host of related practices and established ways of thinking about what data scientists do and how they work.
“Companies that realize the most significant value from data science understand that models are a new type of digital life that calls for different people, processes, and platforms [than those that IT typically supports],” says Thompson.
For more on hiring and retaining data scientists in 2022, check out this helpful page, Managing Data Science Teams. Also, at Rev 3, John Kahan, Microsoft's Chief Data Analytics Officer Emeritus discusses the topic of winning the data science talent race.
Lisa Stapleton is a technology writer and editor in San Jose, CA. She has written and edited for Infoworld, InformationWEEK, LinuxInsider.com, and many other business and technical publications. She is now Domino's Content Director.
RELATED TAGS