Data Science is more than Machine Learning
Domino2018-04-26 | 6 min read
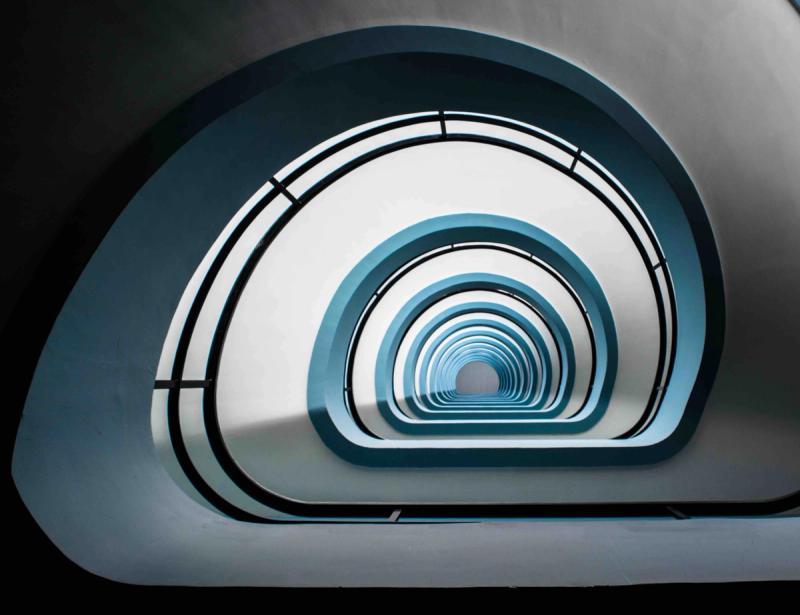
This Domino Data Science Field Note provides highlights and video clips from Addhyan Pandey’s Domino Data Pop-Up talk, “Leveraging Data Science in the Automotive Industry.” Addhyan Pandey is the Principal Data Scientist at Cars.com. Highlights covered in this blog post include Pandey using word2vec to identify duplicate vehicles on the platform, how his data science team refers to predictive models as “data products”, and the company’s overall approach to data science. While this post covers highlights and video excerpts, the full video of his talk is available. If this type of content interests you, visit the Domino Data Science Pop-Up Playlist or consider attending Rev.
Data Science in the Automotive Industry
Addhyan Pandey, Principal Data Scientist at Cars.com, explored how data science is implemented across the company and how data science is more than machine learning in his talk, “Leveraging Data Science in the Automotive Industry.” Pandey covers how
- a core value proposition for an e-commerce site is the relevance of their recommendation system
- he used word2vec to identify duplicate vehicles on the platform when he started at the company
- the data science team refers to predictive models as “data products”
- the company’s current approach to data science work includes building “a product that is robust, build[ing] a model that’s scalable, that’s accurate, and really has very low computational time.”
When the Problem is more than Connecting Buyers and Sellers
Pandey launched his talk with discussing how Cars.com revenue streams include subscription and advertising models. As a result, the problems, or questions, that the data science team tries to solve, go beyond connecting buyers and sellers. The objective is to “manage the overall lifecycle” of a car with multiple points of engagement with both buyers and sellers within the e-commerce marketplace. Pandey points out that a recommendation system, particularly its relevance, is a core value proposition for ensuring that users continue to engage with an e-commerce marketplace.
Using word2vec for Relevance
Pandey noted that when he started at Cars.com, he saw “a huge bunch of text” for the sellers notes and “if I were purchasing a car, I would never ever read that because I’m trained on a website that gives me precise information…." even to figure out “two similar vehicles, it was really tough.” This is a relevant recommendations problem. Pandey decided to address this problem by “putting all of those words in a vector space….aggregate all of those words together in that vector space and have a vector for a particular vehicle.” This allows data scientists to “compute the cosine similarity between the two.” Also, identifying duplicates vehicles on the platform was an added benefit of using word2vec.
Predictive Models as Data Products
Pandey also noted in the talk that “when you talk about data science, it's not really fair to just talk about a specific model… If I just build a model and don't really know how to implement it, I'm not doing justice to the entire system. We call all our predictive models as data products.” He discussed three data products that include
- “data pipelining, which is basically aggregating, doing a lot of data preparation for data scientists, so they don't end up wasting 80% of the time, and also sort of improves the overall real time recommendation predictions really fast.”
- “the algorithm itself. Data scientists really spend a lot of time trying to make the best model that they can for a given problem. That's our second biggest technical product.”
- "And then the third is, once you have this, how do you scale it? How do you make sure that your system is flawless? Or if not flawless, how can you reach that particular state of art perfection? And that's our machine learning platform. That's basically scaling all these predictive models for everyone to utilize and that's another way we sort of democratizing, or decentralizing, data science within the organization.”
“The Whole Picture” of Data Science. It’s Complicated.
Towards the end of the talk, Pandey discussed how his perspective of data science has changed over the years. In 2011, he saw data science as being a predictive model. Then as he worked with more teams, he expanded his perspective. In 2017, he indicates that the “whole picture” of data science is “more complicated” and that data science teams need to “build a product that is robust…build a model that is scalable, that’s accurate, and has very low computational time.”
While this blog post covers a few of the key highlights from Pandey’s talk, the full video is available for viewing. Additional recorded talks from the Domino Data Science events are also available. Yet, if you prefer to attend events in-person, then consider attending the upcoming Rev.
Domino Data Science Field Notes provide highlights of data science research, trends, techniques, and more, that support data scientists and data science leaders accelerate their work or careers. If you are interested in your data science work being covered in this blog series, please send us an email at writeforus(at)dominodatalab(dot)com.
Domino Data Lab empowers the largest AI-driven enterprises to build and operate AI at scale. Domino’s Enterprise AI Platform provides an integrated experience encompassing model development, MLOps, collaboration, and governance. With Domino, global enterprises can develop better medicines, grow more productive crops, develop more competitive products, and more. Founded in 2013, Domino is backed by Sequoia Capital, Coatue Management, NVIDIA, Snowflake, and other leading investors.