Subject archive for "practical-techniques"
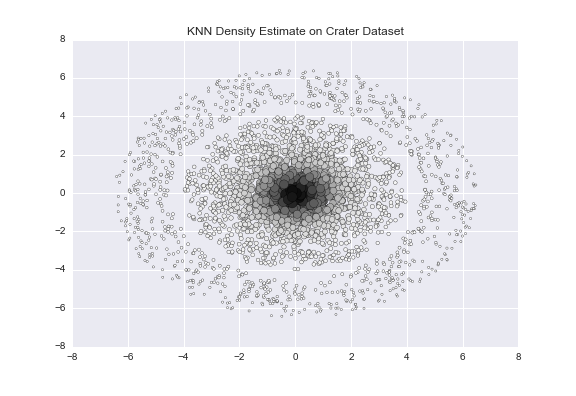
Density-based clustering
Original content by Manojit Nandi - Updated by Josh Poduska.
By Manojit Nandi28 min read
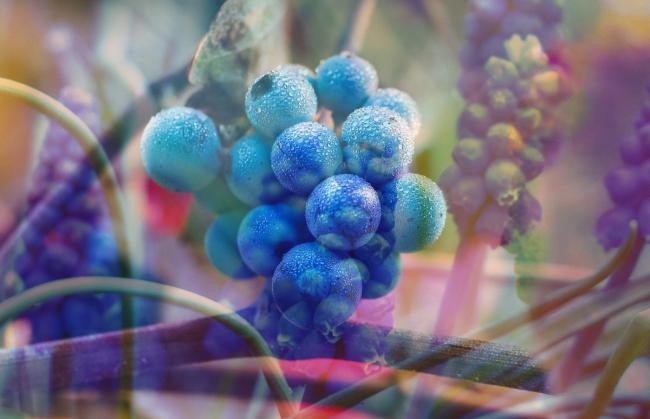
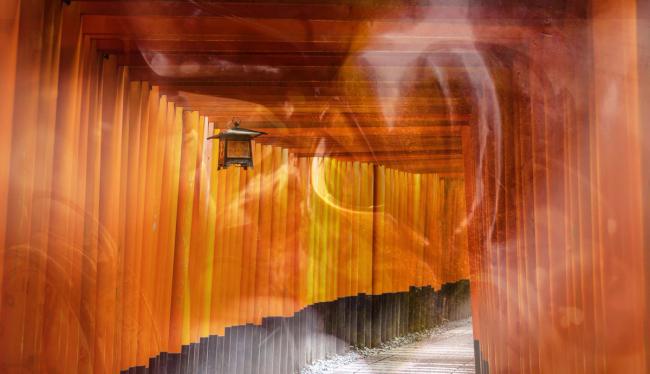
Time series with R
This article delves into methods for analyzing multivariate and univariate time series data.
By Domino13 min read
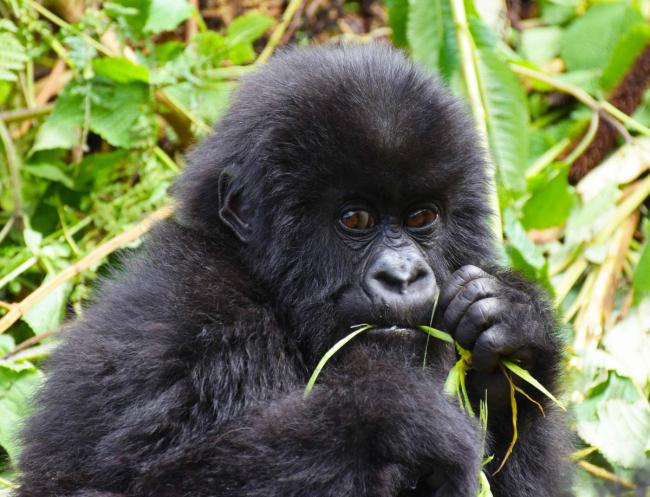
Natural language processing in Python using spaCy: An introduction
This article provides a brief introduction to natural language using spaCy and related libraries in Python.
By Paco Nathan15 min read
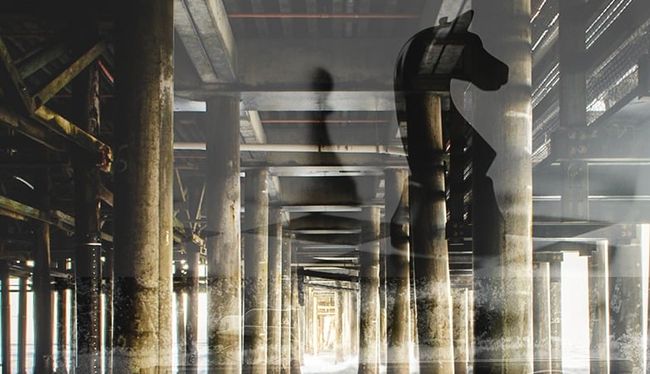
Deep reinforcement learning
This article provides an excerpt "Deep Reinforcement Learning" from the book, Deep Learning Illustrated by Krohn, Beyleveld, and Bassens. The article includes an overview of reinforcement learning theory with focus on the deep Q-learning. It also covers using Keras to construct a deep Q-learning network that learns within a simulated video game environment. Many thanks to Addison-Wesley Professional for the permission to excerpt the chapter.
By John Joo58 min read
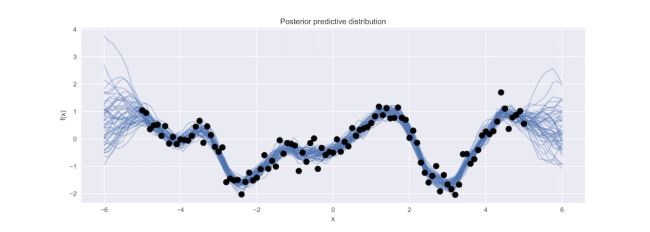
Fitting gaussian process models in Python
A common applied statistics task involves building regression models to characterize non-linear relationships between variables. It is possible to fit such models by assuming a particular non-linear functional form, such as a sinusoidal, exponential, or polynomial function, to describe one variable's response to the variation in another. Unless this relationship is obvious from the outset, however, it involves possibly extensive model selection procedures to ensure the most appropriate model is retained. Alternatively, a non-parametric approach can be adopted by defining a set of knots across the variable space and use a spline or kernel regression to describe arbitrary non-linear relationships. However, knot layout procedures are somewhat ad hoc and can also involve variable selection. A third alternative is to adopt a Bayesian non-parametric strategy, and directly model the unknown underlying function. For this, we can employ Gaussian process models.
By Chris Fonnesbeck27 min read
Subscribe to the Domino Newsletter
Receive data science tips and tutorials from leading Data Science leaders, right to your inbox.
By submitting this form you agree to receive communications from Domino related to products and services in accordance with Domino's privacy policy and may opt-out at anytime.