0.05 is an arbitrary cut off: "Turning fails into wins”
Domino2018-02-15 | 5 min read
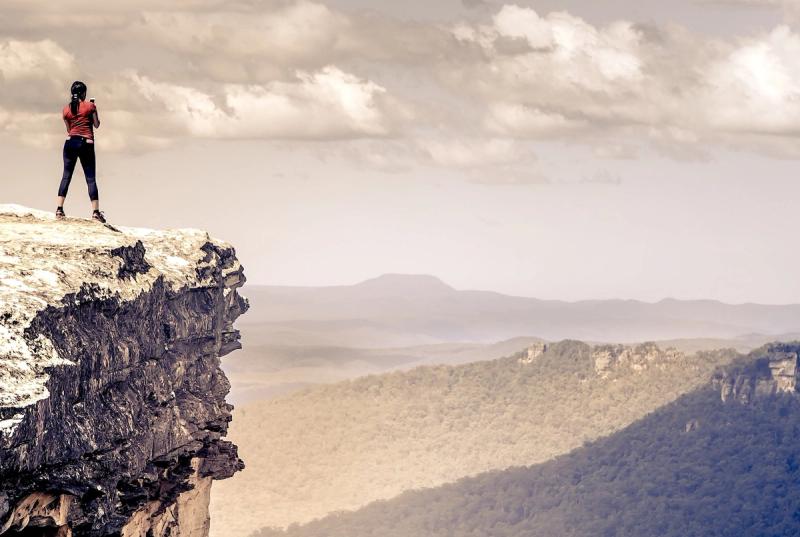
Grace Tang, Data Scientist at Uber, presented insights, common pitfalls, and “best practices to ensure all experiments are useful” in her Strata Singapore session, “Turning Fails into Wins”. Tang holds a Ph.D. in Neuroscience from Stanford University.
This Domino Data Science Field Note blog post provides highlights of Tang’s presentation that include rethinking failed experiments, challenging assumptions, identifying common pitfalls, as well as best practices to avoid bias and duplication of work through sharing knowledge. The post includes the full video presentation. The slides with detailed speaker notes are available for download.
Rethinking failed experiments
Grace Tang, as a data scientist that helps teams design and run experiments, covers bias, common pitfalls, and best practices for turning “failed” experiments into “wins” in her presentation. Tang challenges the assumption that p < 0.05 is always a “win” and p > 0.05 is a “fail” by pointing out that 0.05 is an “arbitrary cut off “and subject to potential change. Through humor, Tang also points out the pressures that people may experience when their primary goal is for their experiment to yield significant results. The implication being that such pressures may reinforce assumptions, lead to common pitfalls, and introduce bias into experiments.
Assumptions and common pitfalls
Tang continues to challenge assumptions by discussing how some “wins” are actually “fails”, particularly when digging deeper into the experiment design and finding out that bias or a confounding variable has been introduced into the experiment via biased sampling, non-random assignment, opt-in bias, too small sample size, too large sample size, or p-hacking. Tang also refers to how organizations may try to avoid “failed” experiments by “not testing at all”. While Tang dives into each potential common pitfall in her presentation, we will be covering p-hacking (also known as: data fishing or data dredging), in this post before jumping into the statistical best practices.
P-hacking, as Tang candidly discusses, is when bias or a false positive is introduced by repeatedly running the experiment until it is significant. Each time an experiment is run, “there’s a certain chance of getting a significant result just by chance”....and “as we run more tests, the chances of getting a false positive adds up”. This is a common enough pitfall that Tang references Randall Munroe’s “Significant”. While humorous, the comic effectively illustrates how p-hacking may provide a false positive. Additional information, albeit more serious in tone, on the effects and consequences of p-hacking is available in this PLOS paper.
Statistical best practices
Tang advocates that in order
“for our fails or non-significant results to be useful, and for any results to be useful, we need to set up our experiment according to statistical best practices so we can trust our results and draw accurate conclusions, and more importantly, adopt the right company culture and processes that encourages the reporting of all experiment results, even the null or harmful results” (Slide 36).
Just a few of the statistical best practices covered in Tang’s presentation include
- list hypotheses before running experiment as this may help discourage p-hacking later
- ”generalize conclusions only to included groups” in random sampling
- ensure that with random assignment there are “no systematic differences between treatment and control groups”...that “could become a confounding variable”. Consider using random generators or UUIDs (universally unique identifiers) and stay away from using properties like name, email, and phone numbers.
- consider using the Bonferroni correction to “keep the false positive rate under control” or correcting for multiple comparisons.
- sharing knowledge enables everyone to learn and “also prevents duplication of effort”
Conclusion
Tang closes out the presentation with emphasis on organizations creating a culture where the seeking the “truth” is emphasized more than “significance”. Tang also calls for using the results of “failed” experiments to feed into future design-build-test iterations in order to improve “strategy over time instead of relying on gut feel”. The combination of utilizing statistical and cultural best practices allows data scientists and their organizations to embrace how “failure is the mother of success”.
Domino Data Science Field Notes provides highlights of data science research, trends, techniques, and more, that support data scientists and data science leaders accelerate their work or careers. If you are interested in your data science work being covered in this blog series, please send us an email at writeforus@dominodatalab.com.
Domino Data Lab empowers the largest AI-driven enterprises to build and operate AI at scale. Domino’s Enterprise AI Platform provides an integrated experience encompassing model development, MLOps, collaboration, and governance. With Domino, global enterprises can develop better medicines, grow more productive crops, develop more competitive products, and more. Founded in 2013, Domino is backed by Sequoia Capital, Coatue Management, NVIDIA, Snowflake, and other leading investors.