Mastering AI workflows in the enterprise with new enhancements to Domino Flows
Ayca Akguc2024-11-07 | 4 min read
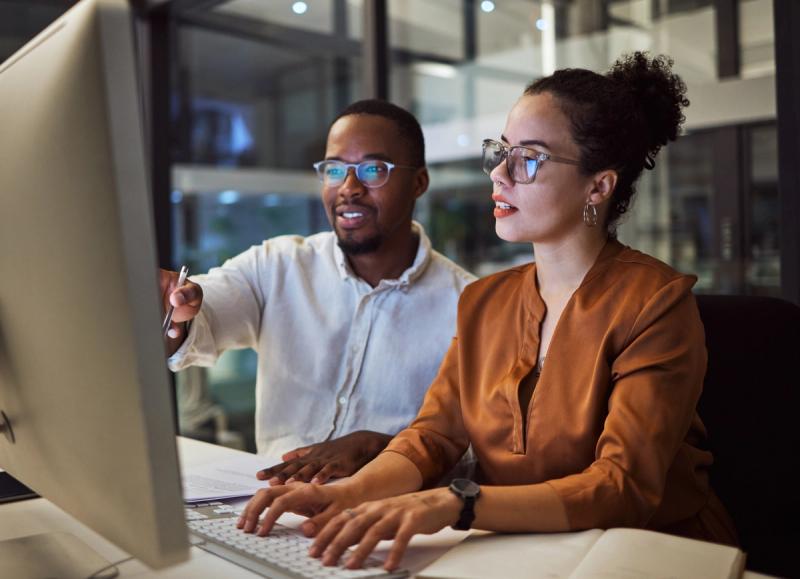
AI workflows are critical to modern data science operations, guiding teams through building, deploying, and maintaining machine learning models. These workflows require seamless coordination across teams and infrastructure and involve data preparation, experimentation, deployment, and retraining. As AI projects scale, managing these workflows becomes increasingly complex.
Domino Flows, launched a few months ago, simplifies workflow orchestration by automating key tasks. Domino Flows accelerates AI-driven research, particularly in industries like life sciences, with features like flow visualization, multi-infrastructure support, and targeted re-runs. Domino is now introducing enhancements to Flows, further streamlining workflow management for enterprise data science teams. In this post, we’ll explore these new features and share best practices to maximize the efficiency of workflows.
Bookmarking artifacts for quick access
Quickly accessing critical outputs — like models, datasets, or reports — is crucial in fast-paced enterprise AI environments. Domino’s artifact bookmarking feature allows users to flag essential outputs during flow execution for easy access and collaboration.
Top best practices include:
- Bookmark key outputs early: Identify and bookmark critical artifacts as soon as they’re generated during the flow. This proactive approach minimizes time spent searching for essential outputs later.
- Automatic visibility: Domino automatically makes the artifact visible to everyone with access to the project, making collaboration and reuse seamless.
By following these practices, teams can focus more on innovation and less on administrative tasks.
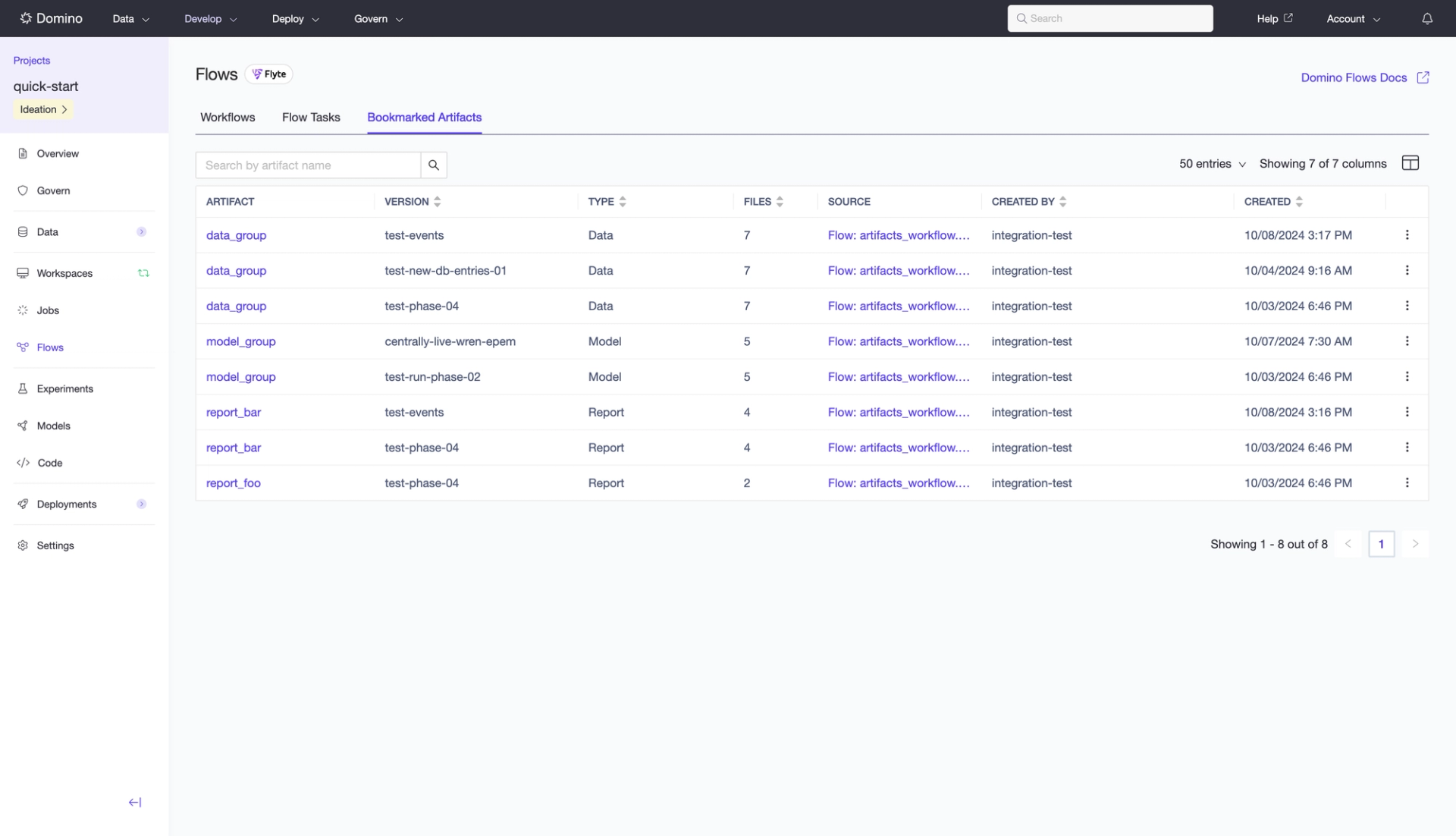
Gaining clarity with visual workflow maps
AI workflows can become complex, with multiple dependencies across tasks. A clear visual representation of the workflow is essential for debugging, optimizing performance, and enhancing team collaboration. Domino’s visual workflow mapping offers an interactive, graphical layout of the entire flow.
Top best practices include:
- Review dependencies regularly: Use the visual map to review task dependencies regularly and identify bottlenecks or redundant steps. This can lead to significant optimizations and reduced computational overhead.
- Leverage version comparisons: Use Domino’s version comparison feature to track changes between iterations and assess how modifications — such as new datasets or tuning parameters — affect overall performance.
These practices help teams stay organized and quickly identify areas for improvement.
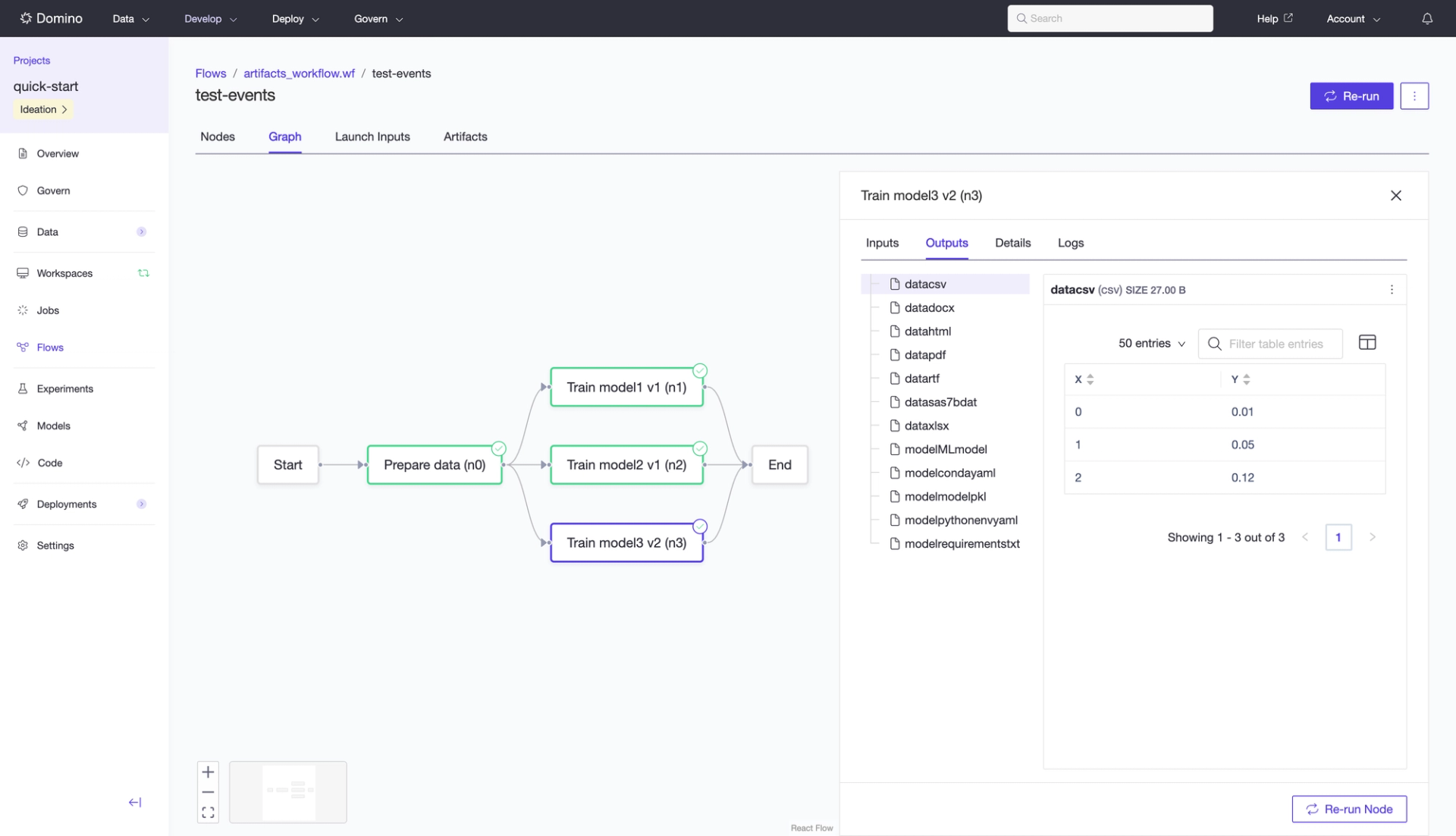
Streamlining iteration with effortless re-runs
Data scientists often need to retrain models with new data or adjust parameters to improve performance. Domino Flows simplifies this process by allowing seamless re-runs of workflows or specific nodes directly from the Domino UI.
Top best practices include:
- Use prefilled inputs for speed: Domino’s ability to prefill inputs from previous executions reduces manual setup time, making rerun workflows with new data or adjusted parameters easier.
- Target specific nodes: Instead of re-running entire workflows, enable caching for expensive tasks in the workflow and focus on re-running specific nodes that need updating. This saves time and compute resources.
With streamlined iteration, teams can move quickly from hypothesis to production.
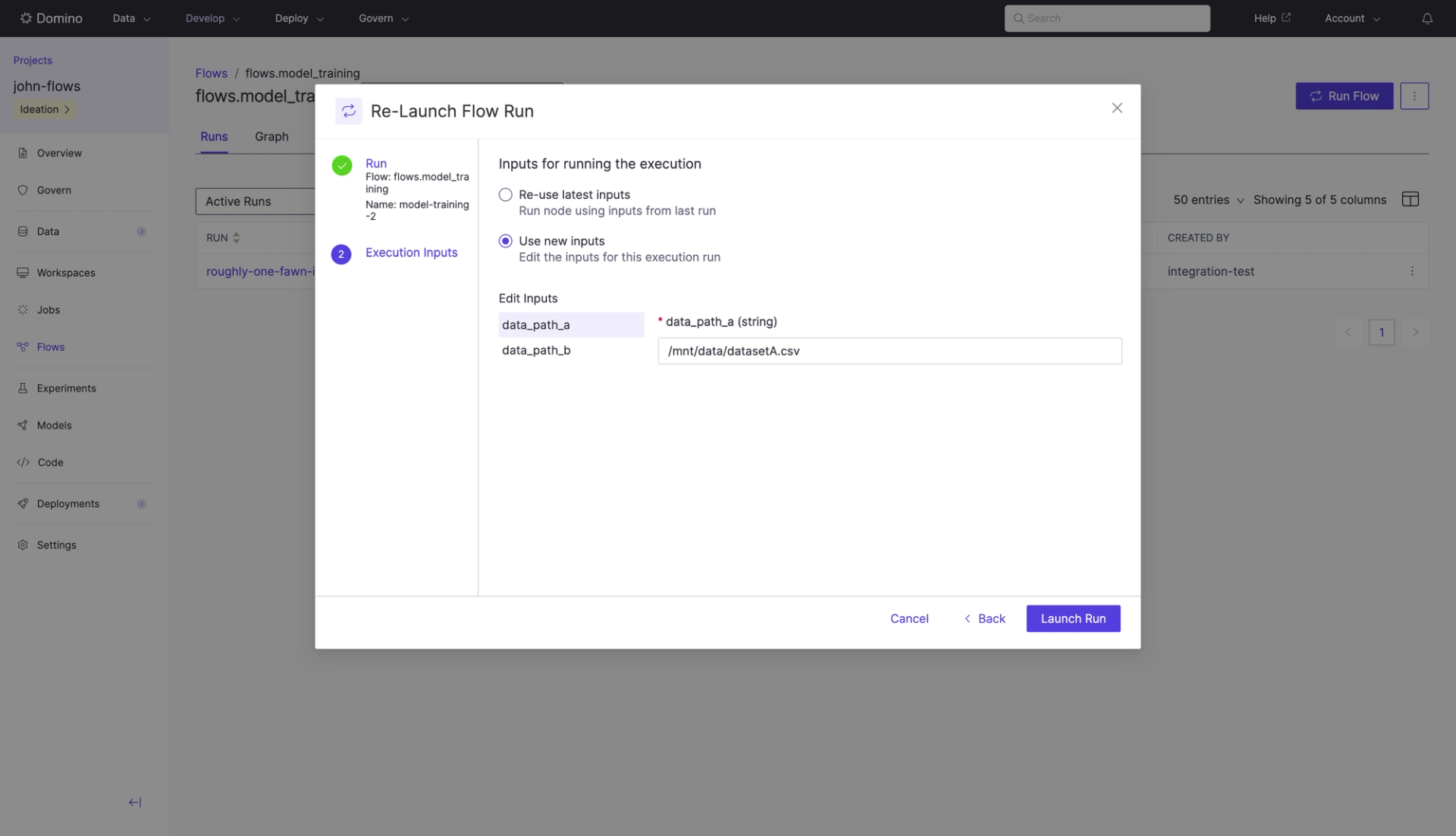
Elevate your AI workflows: See Domino Flows in action
With these new capabilities, Domino Flows empowers data science teams to manage AI workflows with greater clarity and efficiency. These enhancements reduce friction, enabling teams to focus on driving innovation.
Ready to see Domino in action? Join us for a live demo to explore how these features can transform your workflow orchestration and supercharge your AI projects. Don’t miss this opportunity to see how Domino can help your team work smarter and faster!
Ayca Akguc is a product marketing manager with 15+ years in tech product development and marketing. As Director of Product Marketing at Domino Data Lab, she focuses on empowering enterprises to harness AI responsibly and effectively, specializing in data science, ML, and AI audiences.