Governance meets scalable inference: Domino + Amazon SageMaker
David Schulman2024-11-19 | 6 min read
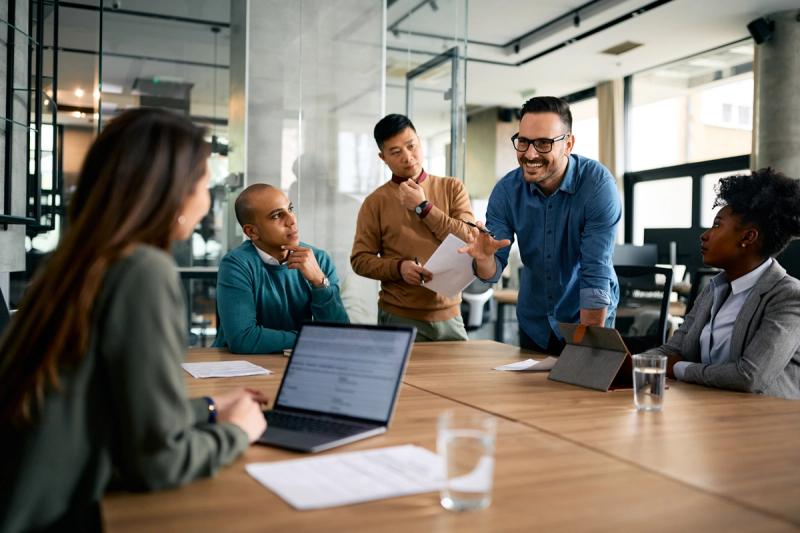
Co-authored by James Yi, Senior AI/ML Partner Solutions Architect, AWS, and Ayca Akguc, Director of Product Marketing, Domino Data Lab
In today’s AI-driven landscape, enterprises are racing to scale AI from pilot to production. Gartner® predicts that “Through 2025, at least 30% of GenAI projects will be abandoned after proof of concept (POC) due to poor data quality, inadequate risk controls, escalating costs or unclear business value." One of the biggest obstacles to production is fragmented governance, which complicates tracking, reproducibility, auditing, and compliance. This lack of cohesion leads to costly delays and heightened risks, making it difficult for organizations to consistently deliver on AI initiatives. To truly unlock the full potential of AI, enterprises need a seamless solution that merges robust governance with scalable deployment — this is where Domino’s integration with Amazon SageMaker excels. Let’s dive into how this partnership is revolutionizing AI deployment.
Domino + Amazon SageMaker: Best of both worlds
Data science leaders face mounting pressure to deliver models swiftly and consistently, whether for predictive analytics, fraud detection, personalization, or cutting-edge generative AI applications.
With Domino, enterprises can efficiently deliver mission-critical AI, ML, and data science solutions at scale with speed, cost-effectiveness, and compliance.
- Domino provides code-first data science teams with self-service access to their preferred tools, compute resources, and data without needing DevOps skills.
- Teams have centralized and secure access to data across all sources, environments, and locations.
- Domino accelerates the entire model lifecycle — from development and experimentation to deployment and monitoring — by enabling seamless automation and collaboration across teams.
- As the system of record for AI assets, Domino preserves institutional knowledge and supports audit and compliance needs. It ensures reproducible, reusable results and governance at every stage while enhancing quality and reducing risks.
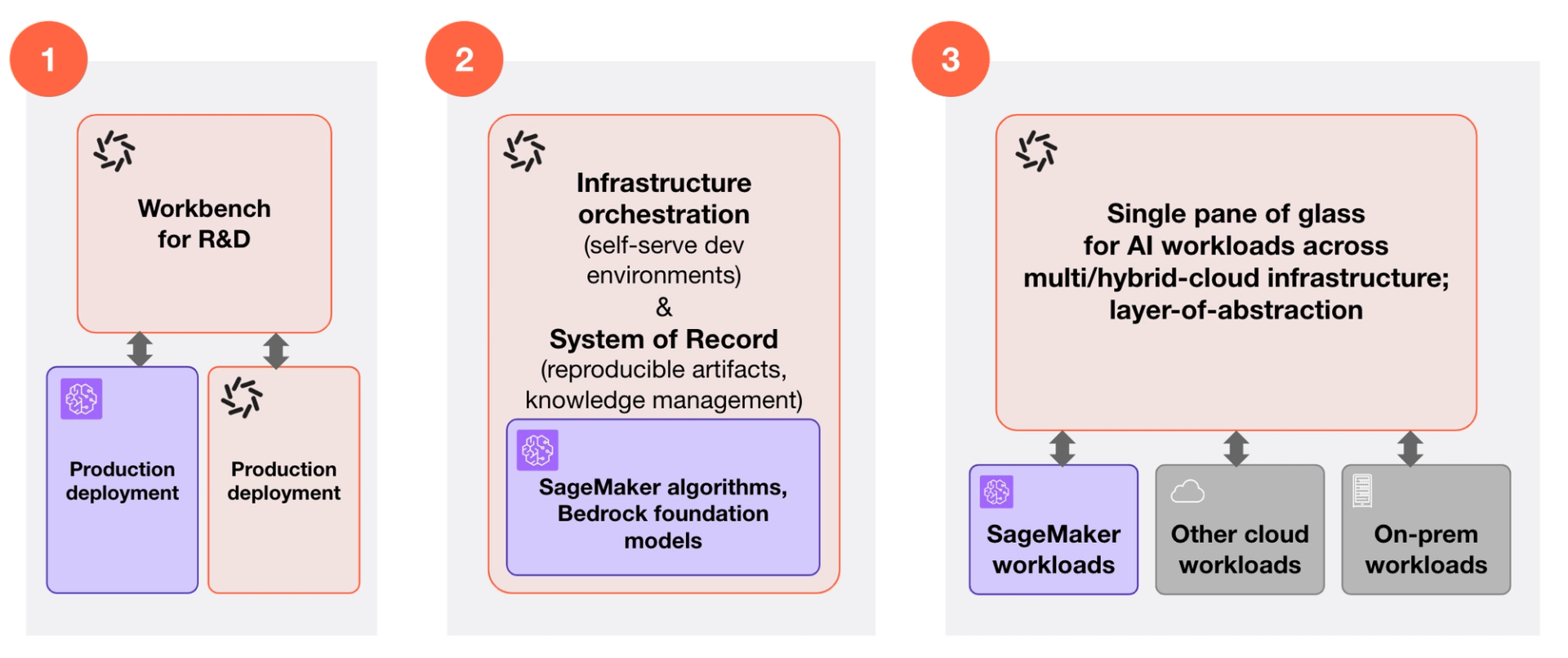
With Domino and Amazon SageMaker, enterprises can build a powerful, flexible, and efficient AI/ML infrastructure that unlocks the following core capabilities:
- Research to production pipeline: Leverage Domino for research and experimentation, then seamlessly deploy production-ready models to Amazon SageMaker.
- AI innovation synergy: Utilize Domino to manage the complete model lifecycle while integrating cutting-edge AI innovations from AWS, such as Amazon Titan foundation models.
- Multi-environment orchestration: Employ Domino as the central hub for orchestrating production workloads across various environments, including Amazon SageMaker, providing a unified management layer for diverse deployment scenarios, enhancing flexibility and control.
Develop and govern with Domino, deploy to Amazon SageMaker
Let’s dive into how companies can harness Domino for research and experimentation, then seamlessly deploy production-ready models to Amazon SageMaker. Here’s how this integration empowers data science teams:
- Reduce operational overhead:
- Automate model packaging, transfer, and scaling within CI/CD workflows
- Simplify the transition from development to production
- Eliminate manual steps to accelerate deployment and ensure consistency
- Ensure control and compliance:
- Track full model lineage for every build and deployment
- Maintain a unified, traceable system of record
- Simplify audits and gain complete visibility across the model lifecycle
- Maximize performance and efficiency:
- Configure SageMaker features like autoscaling directly within Domino
- Enable dynamic scaling based on real-time demand
- Leverage AWS Inferentia for high-performance, cost-effective inference
Deployment made simple: A quick walkthrough
1. Set up deployment targets
- Configure SageMaker as an external deployment target within Domino
- Define resources, permissions, and other settings like regions with ease
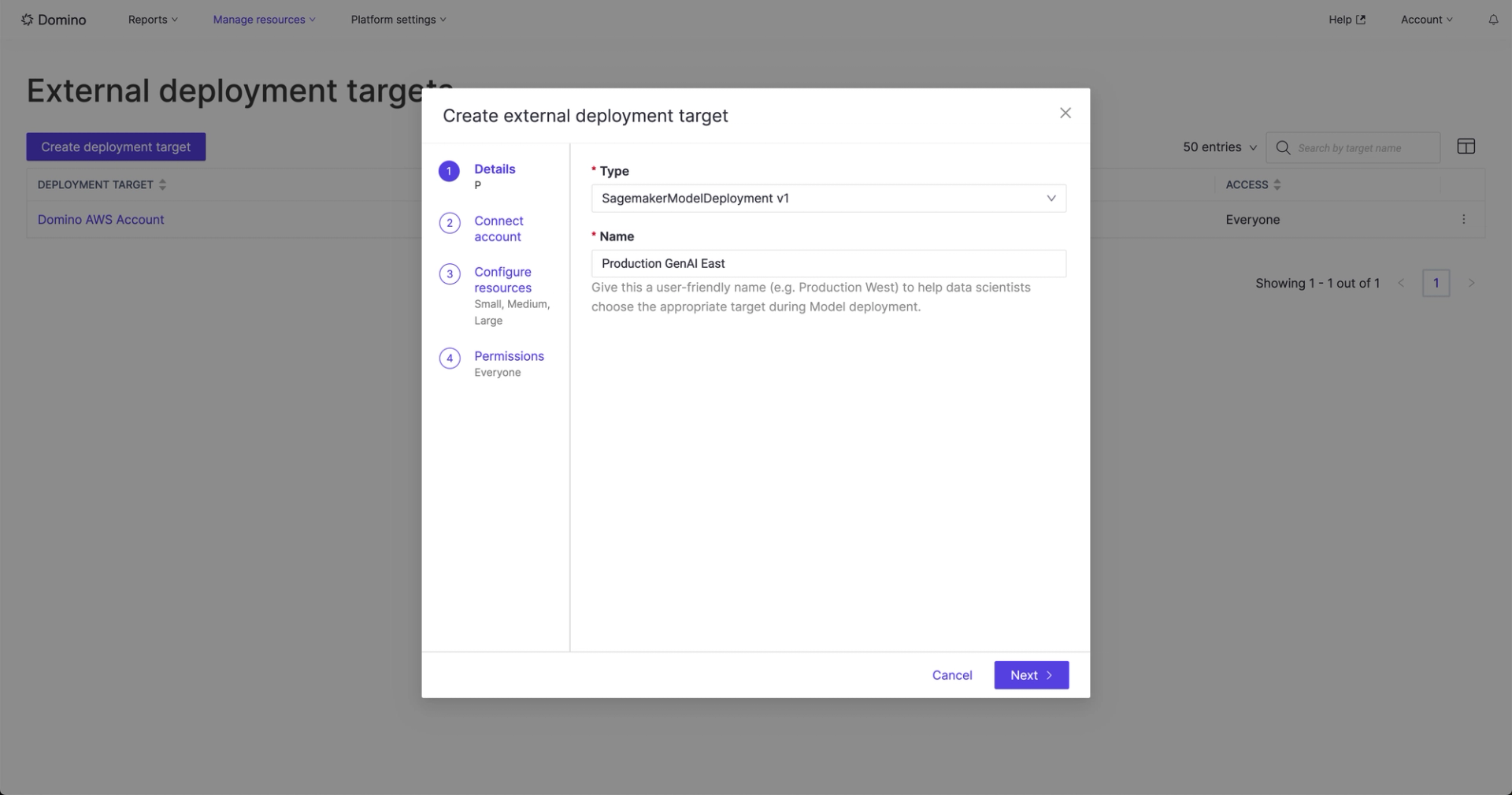
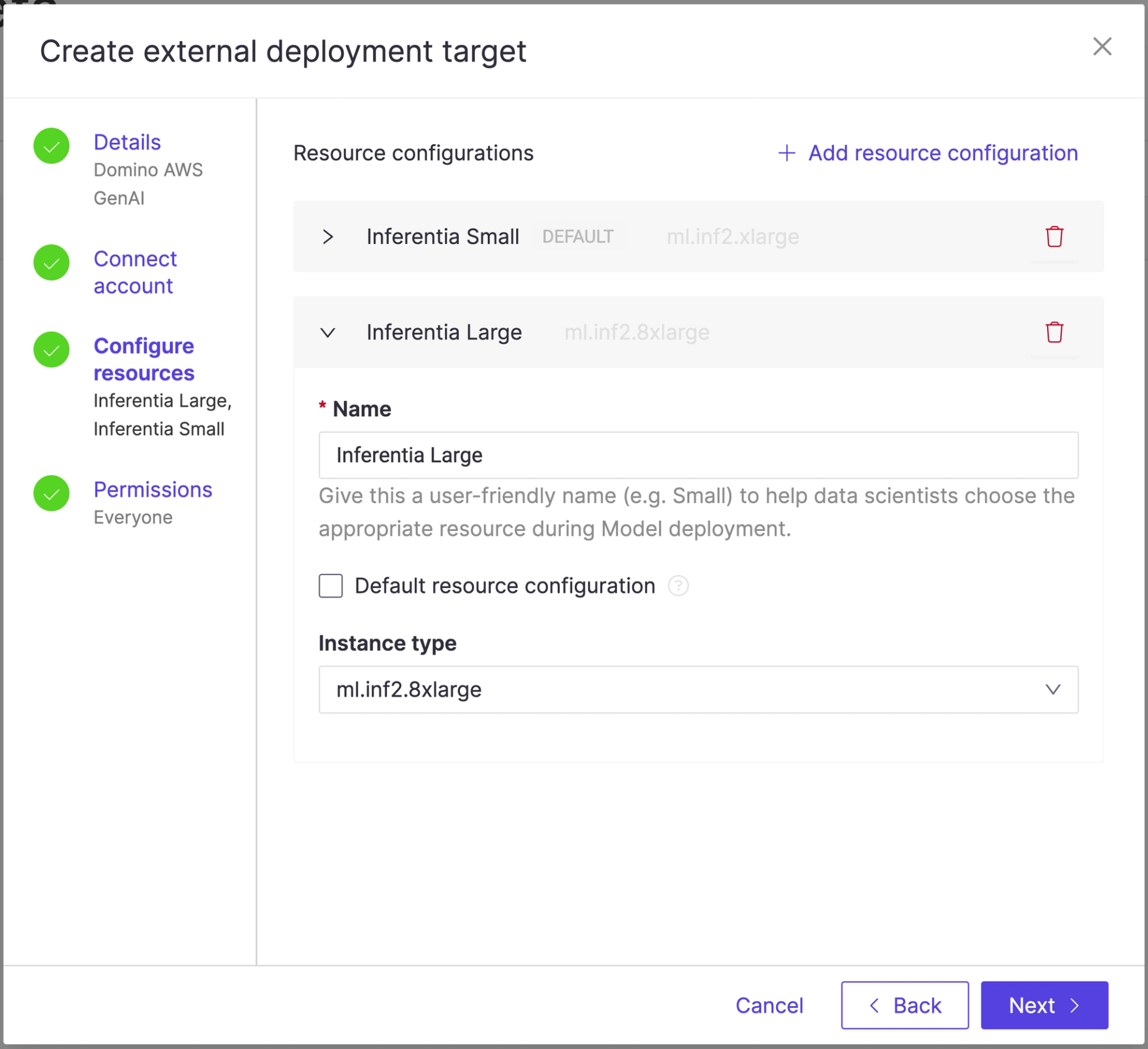
2. Deploy models in a few clicks
- Select SageMaker as the deployment target
- Configure instance parameters and enable auto-scaling directly from Domino's interface
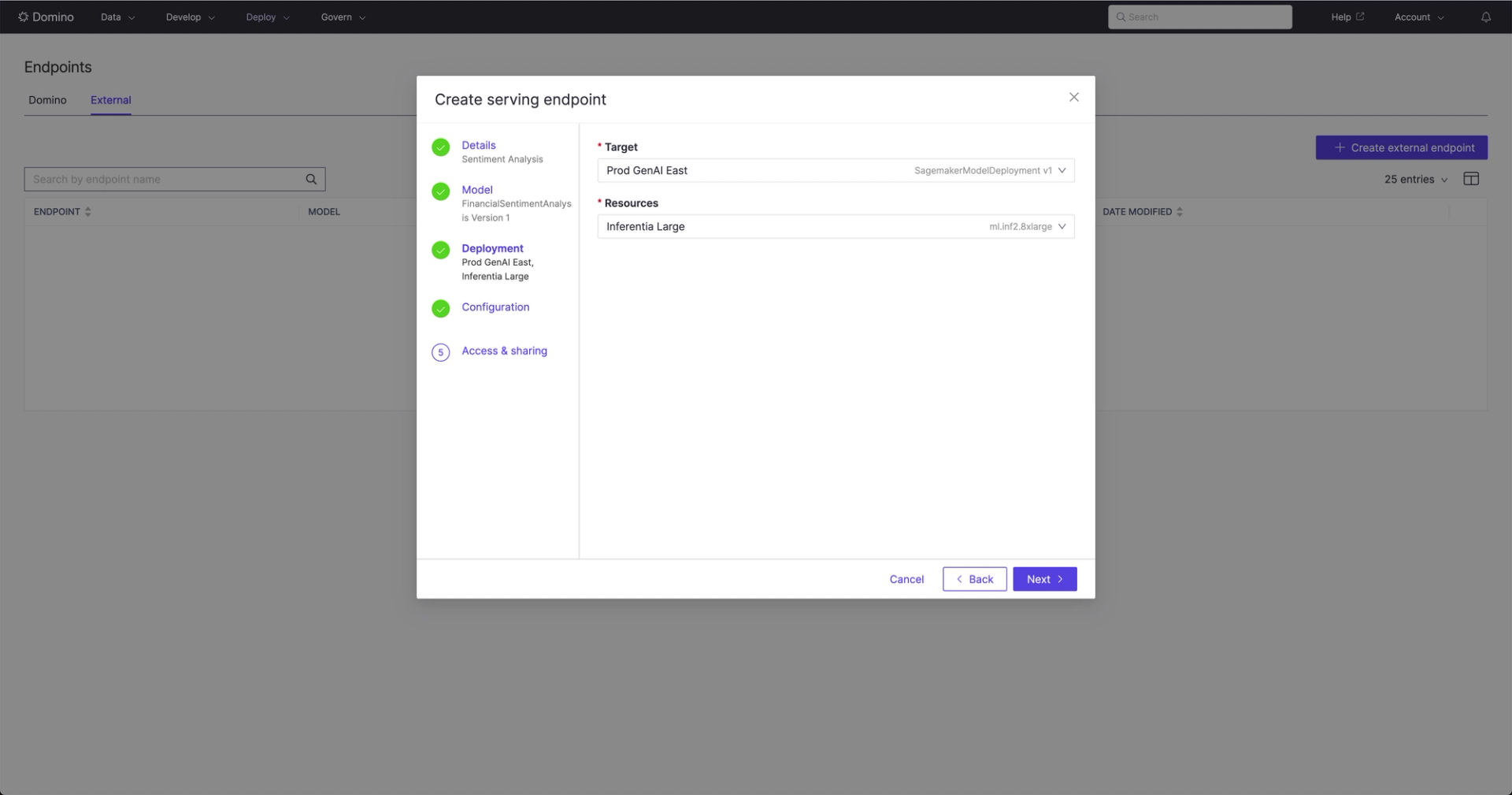
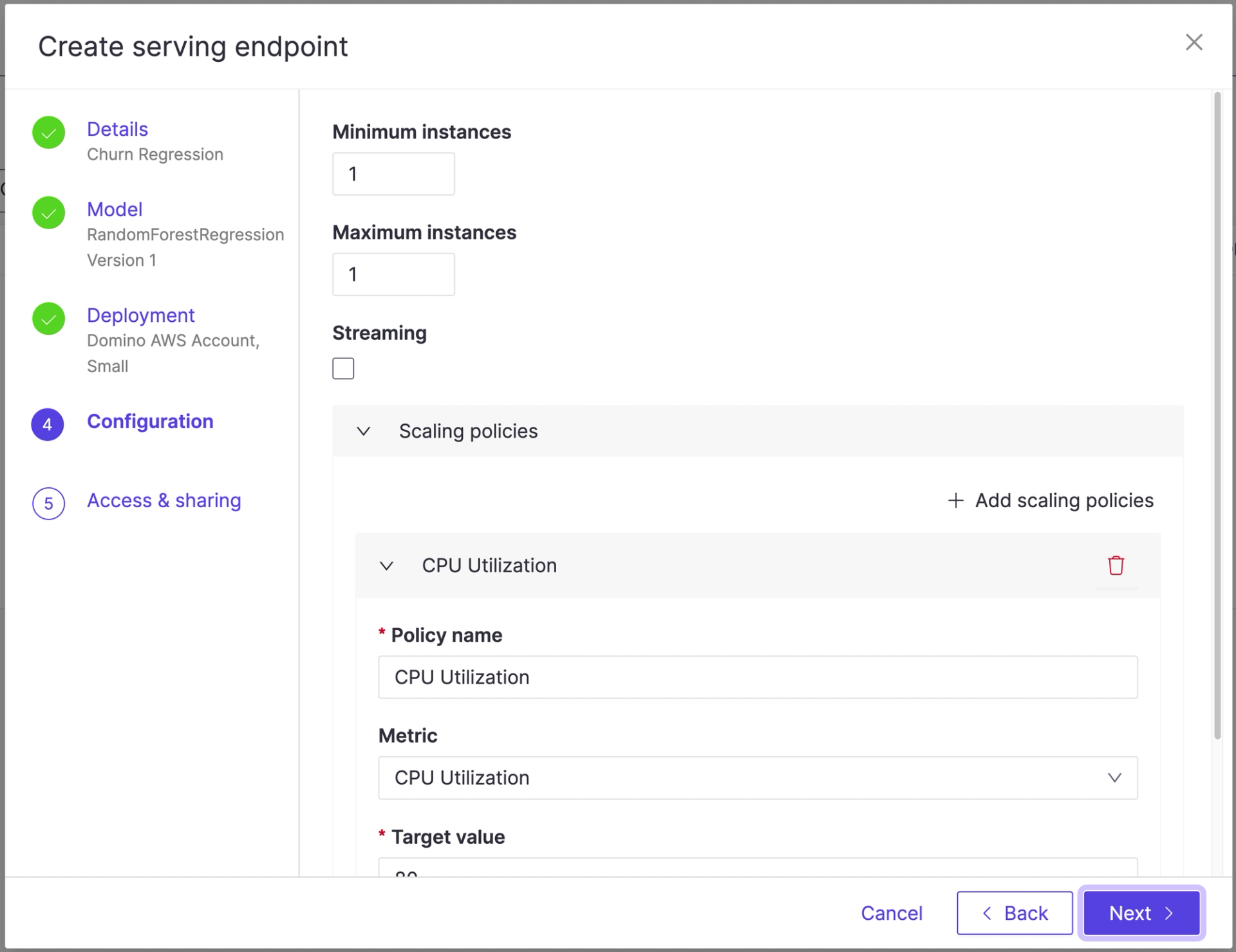
3. Monitor and manage with precision
- Track status, resource usage, and compliance from Domino's centralized interface
- Ensure models remain optimized and auditable throughout their lifecycle
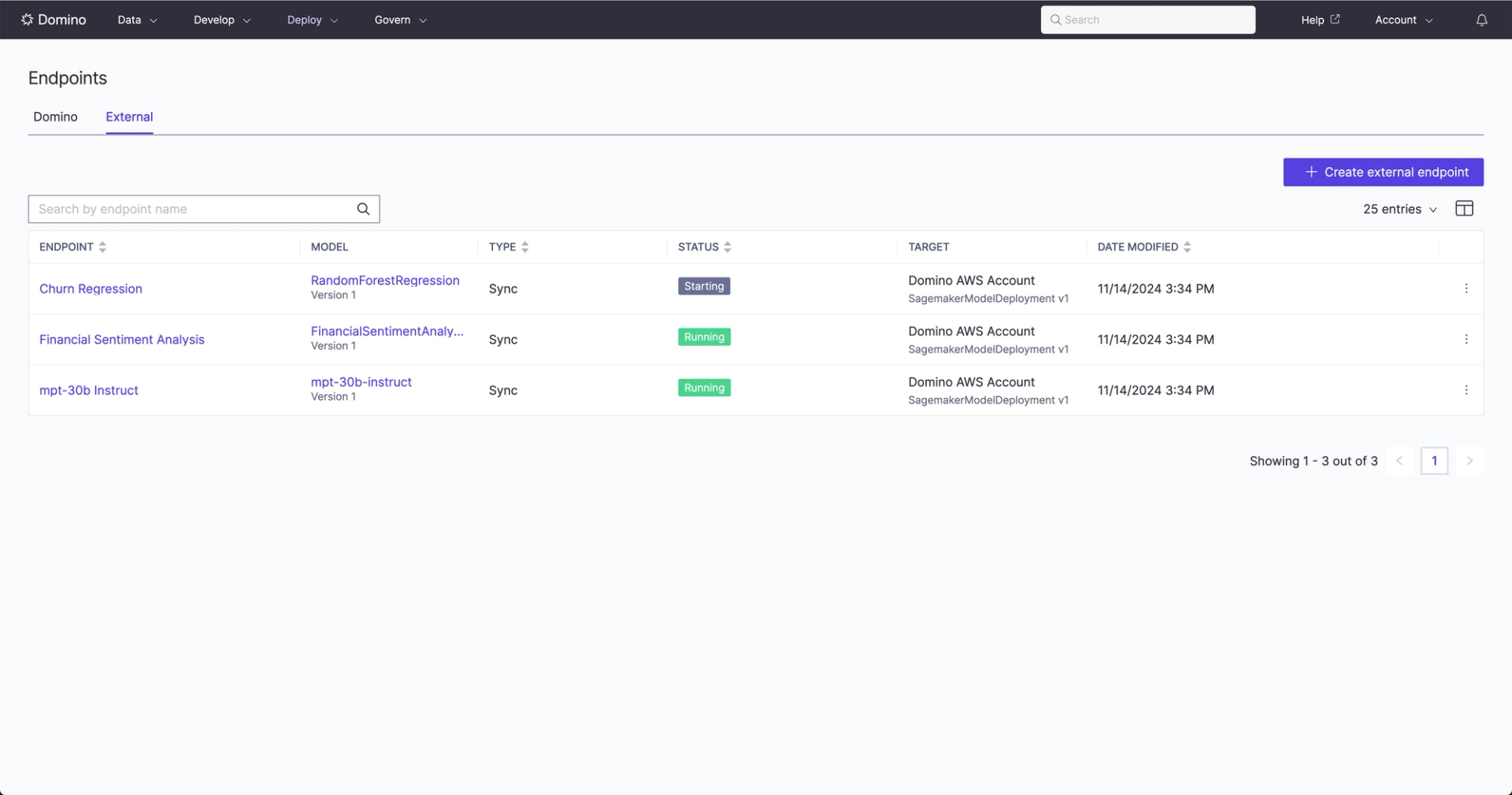
Move your pilots to production seamlessly while enhancing compliance
Domino's integration with Amazon SageMaker addresses the key challenges of model deployment; this powerful combination empowers enterprises to:
- Accelerate time-to-value for AI initiatives
- Maintain rigorous governance and compliance
- Optimize costs and performance at scale
- Tackle the unique demands of generative AI deployment
Ready to supercharge your AI deployment? Watch this demo video to see the Domino and Amazon SageMaker integration in action. For more insights, check out our AWS partner page to learn how Domino and Amazon SageMaker can transform your AI initiatives, and join us on Wednesday, December 11th for a webinar featuring AWS.
Source attribution
Gartner, 10 Best Practices for Scaling Generative AI Across the Enterprise, By Arun Chandrasekaran, Leinar Ramos, Alberto Pietrobon, 10 January 2024. GARTNER is a registered trademark and service mark of Gartner, Inc. and/or its affiliates in the U.S. and internationally and is used herein with permission. All rights reserved.
David Schulman is a data and analytics ecosystem enthusiast in Seattle, WA. As Head of Partner Marketing at Domino Data Lab, he works with closely with other industry-leading ecosystem partners on joint solution development and go-to-market efforts. Prior to Domino, David lead Technology Partner marketing at Tableau, and spent years as a consultant defining partner program strategy and execution for clients around the world.