Domino 5.0: Rapidly Update Production Models for Optimal Performance
2022-01-31 | 6 min read

Introducing Integrated Model Monitoring in Domino 5.0
By Patrick Nussbaumer, Director of Product Marketing at Domino, on January 31, 2022, in Product Updates
Continuous drift and accuracy monitoring is critical to maintaining effective models that drive your business. In fact, industry estimates show a ~30% loss of business value by not monitoring. However, a survey conducted by DataIQ shows that only 10.5% of organizations have automated model monitoring for drift and quality.
Developing an enterprise model monitoring solution to ensure all models are operating at peak performance has proven to be challenging for many organizations. Why? Common challenges include:
- Complexity building a data pipeline to compare
- Prediction data with training data, and
- Model inference with ground truth data
- Creating dashboards to view monitoring performance
- Analyzing large numbers of features to identify the source of model degradation
- Time-consuming to reproduce the production model in development for remediation
Some companies have solved one or more of these problems, but they are challenged when they have to repeat the process over and over again, especially as more models are moved into production. This friction in the deployment to monitoring to remediation to re-deployment process severely limits model velocity. If you’re interested in seeing how your organization measures up, check out the Model Velocity Assessment for a free and easy way to identify where you may have issues.
Domino is the Enterprise MLOps platform that seamlessly integrates code-driven model development, deployment, and monitoring to support rapid iteration and optimal model performance so companies can be certain to achieve maximum value from their data science models.
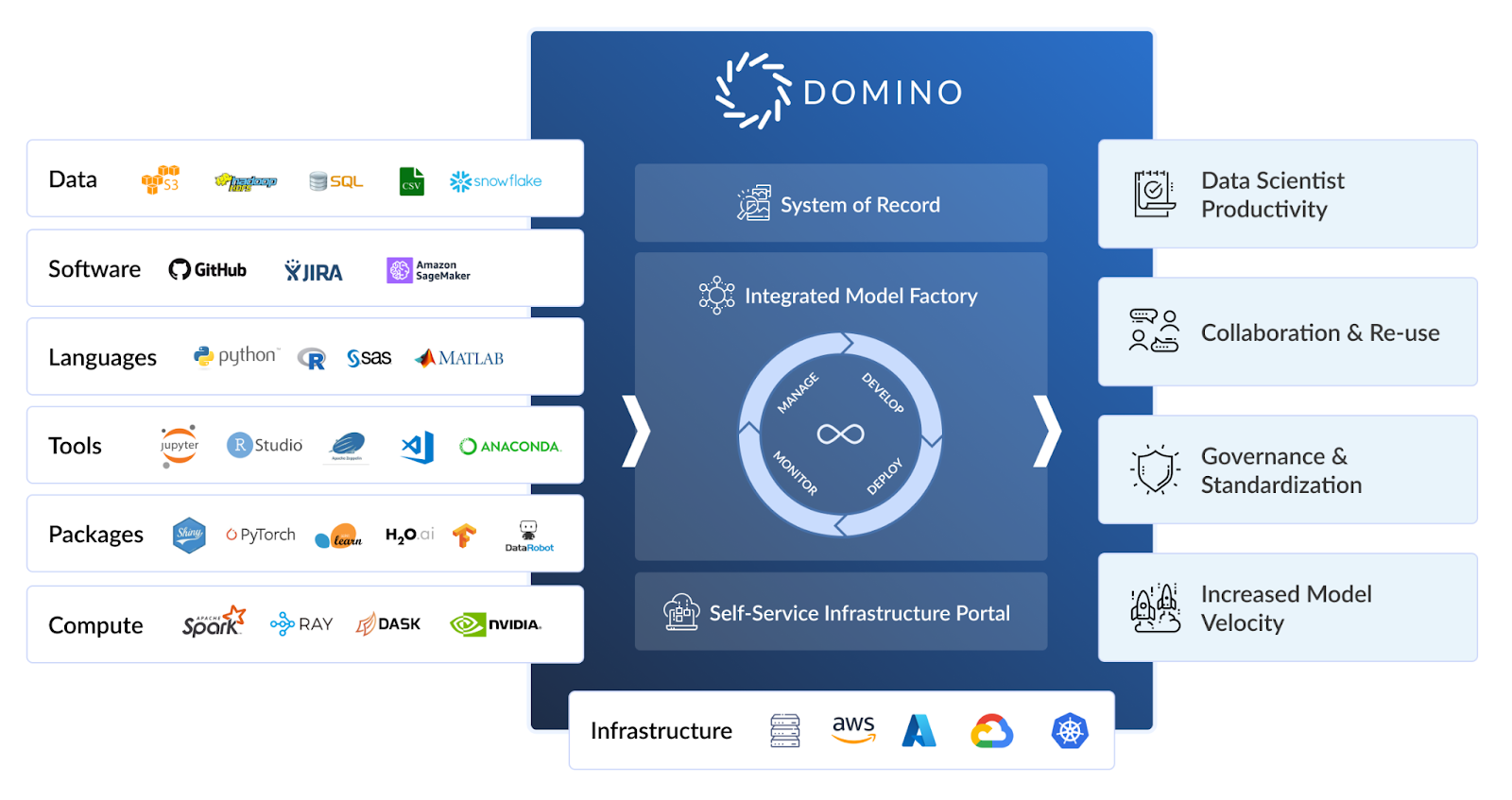
How it Works
The Only Closed-Loop Development-Deployment-Monitoring-Remediation-Deployment Process
Integrated Monitoring makes building monitoring pipelines easy. Domino automatically sets up prediction data capture pipelines and model monitoring for deployed models to ensure peak model performance.
Development-Deployment-Monitoring
With just two lines of code, data scientists can persist features, training data, and predictions for analysis and monitoring. One line of code is needed during training to capture the training data and another in the API call to capture the prediction data.
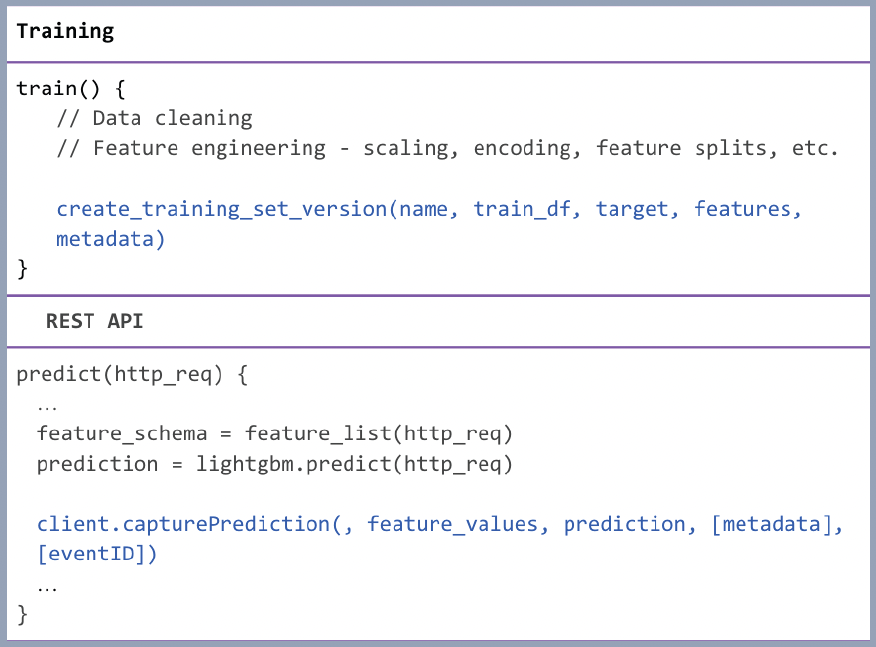
Once the model is deployed, the configuration of monitoring is simple.
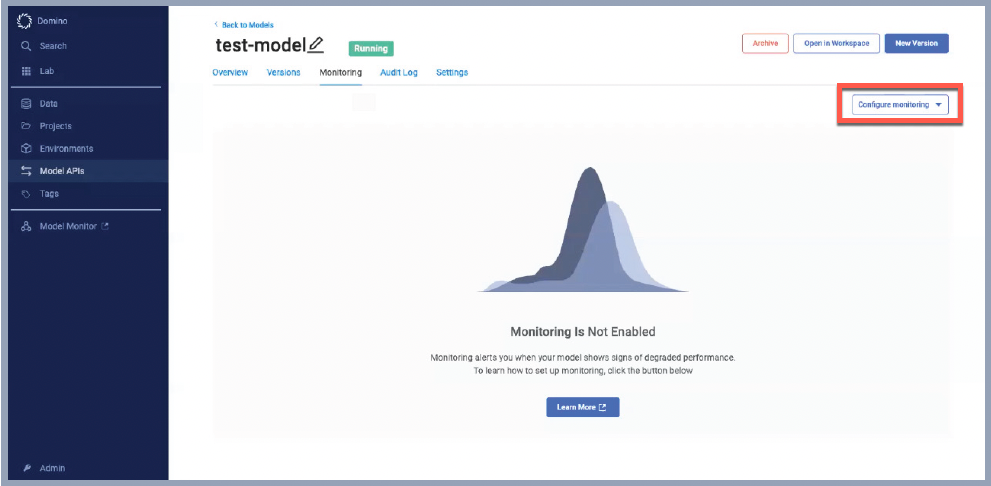
Simply select the training data and the appropriate version and monitoring are enabled.
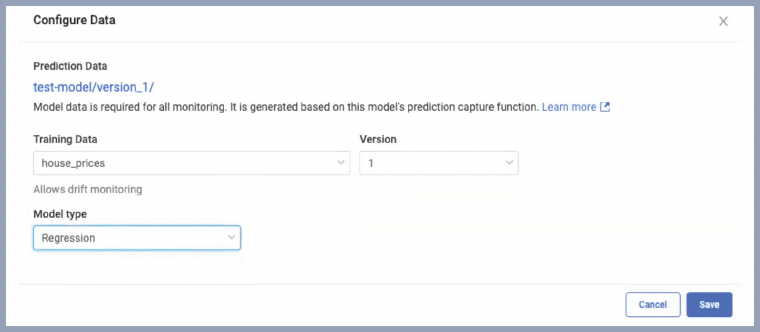
Next, configure checks and automatic alerts for data and model drift.
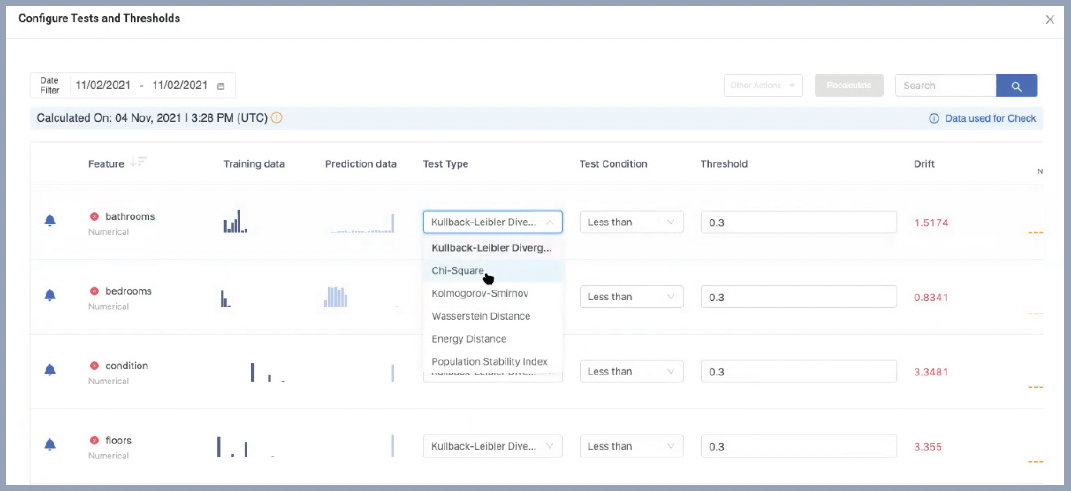
Domino will then connect to live prediction data and automate a continuous monitoring pipeline. As soon as predictions are made, Domino will show monitoring statistics.
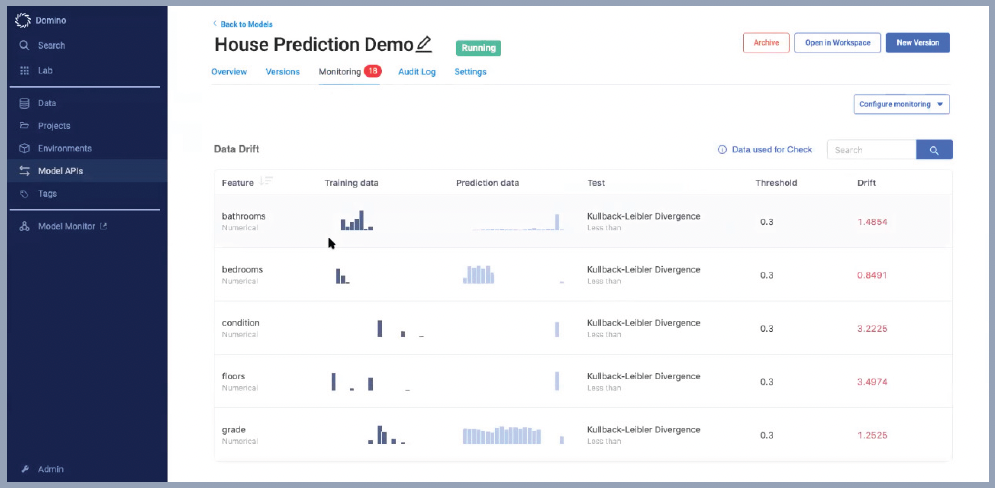
The model is visible in a centralized dashboard that provides a single pane of glass for models, summaries, and drift details. Users have the ability to drill into details and configure alerts for drifting features and model quality issues.
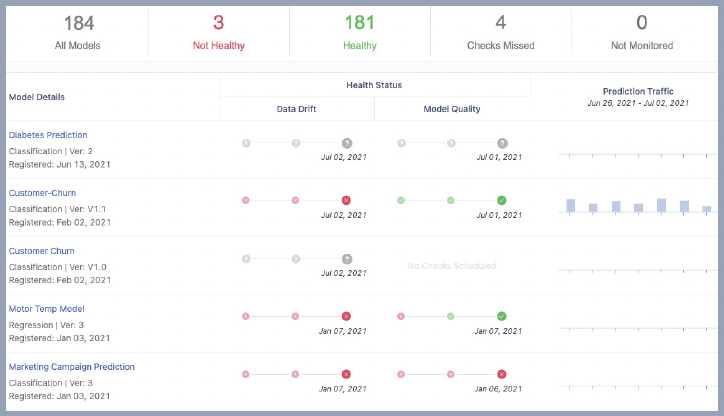
Remediation-Deployment
When a model’s performance has degraded, easily drill into model performance for more details.
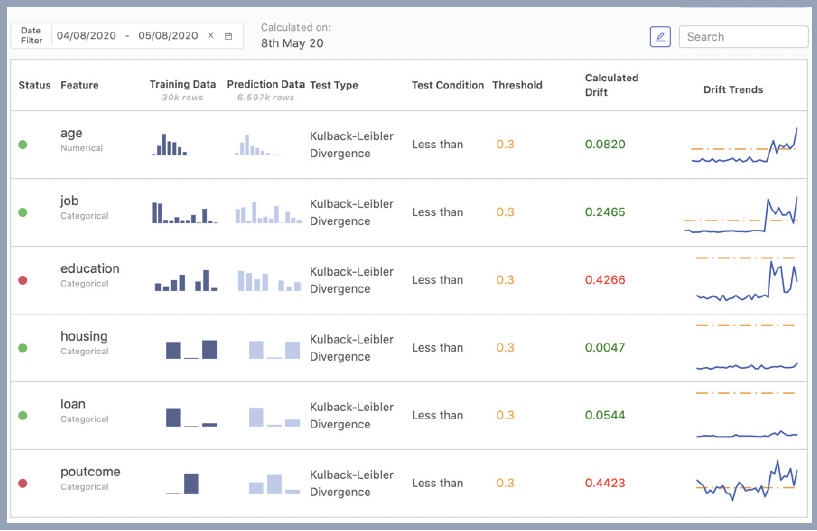
When problems arise with model accuracy, Domino generates a model quality report with actionable insights that highlight issues in your model and data. The report includes a number of analyses to help data scientists understand and diagnose drift.
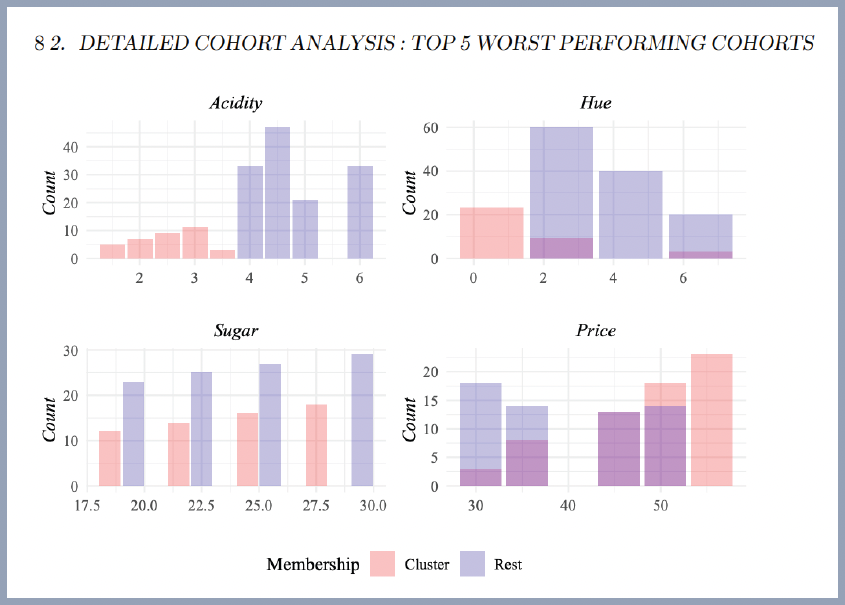
When you’re ready to remediate your models, easily launch the development environment with the original reconstituted state of your models.
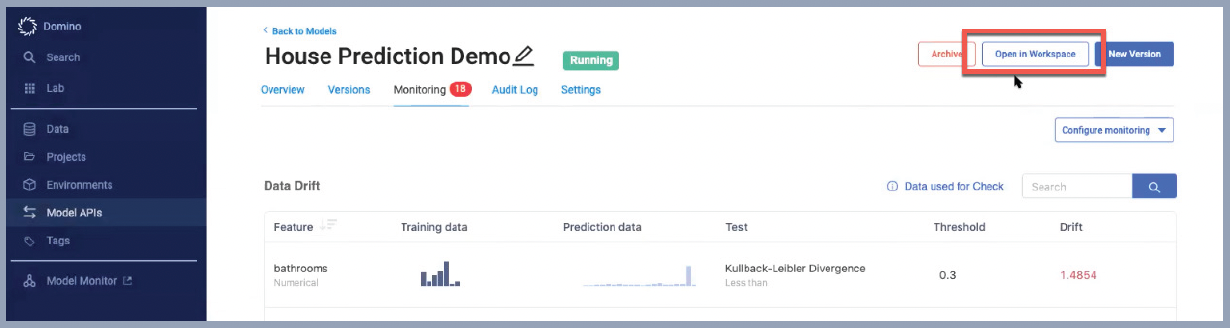
Once your workspace is launched, it will have the original code, notebooks, training data and docker image used to build the model. Also loaded will be the production data needed to debug, analyze and compare with the original training data.
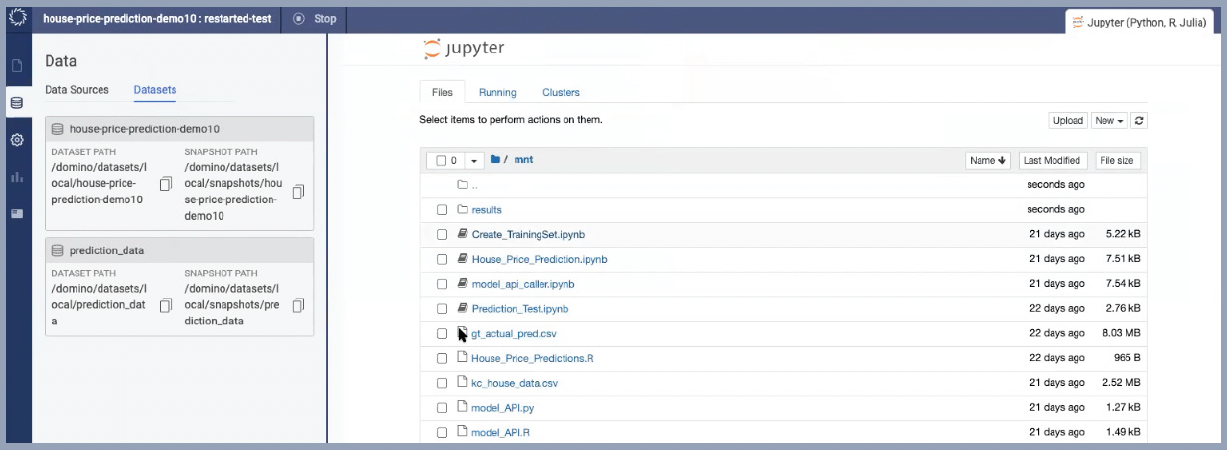
Once a model has been retrained and performance remediated, re-deployment and monitoring of the new model version are just a few clicks away.
Conclusion
Domino, with a focus on helping organizations improve model velocity, has released features for the first integrated, closed-loop experience to drive model velocity and maintain maximum performance of data science models. With capabilities that help automatically set up monitoring and prediction data pipelines, seamless integration with dashboards; alerts, and the generation of actionable insights reports; and the ability to quickly set up a development environment to remediate models and get them back into production again, organizations are able to focus on developing new models while ensuring deployed models are providing business value.
New and existing customers who install or upgrade to Domino 5.0 will automatically have access to a specific capacity of monitored data so they can get started with a closed-loop monitoring system. Customers can work with their sales teams to forecast and purchase additional capacity as needed.
About the Author
Patrick Nussbaumer is a Director of Product Marketing at Domino Data Lab.
He has a BS degree in Electrical Engineering from The University of Illinois at Urbana-Champaign, and an MBA from the University of California - Irvine. Patrick has nearly 30 years of experience in the data and analytics industry.
RELATED TAGS
Subscribe to the Domino Newsletter
Receive data science tips and tutorials from leading Data Science leaders, right to your inbox.
By submitting this form you agree to receive communications from Domino related to products and services in accordance with Domino's privacy policy and may opt-out at anytime.