Set Yourself Up for Generative AI Success with the Top “Low Hanging” Use Cases
Kjell Carlsson2023-09-28 | 4 min read
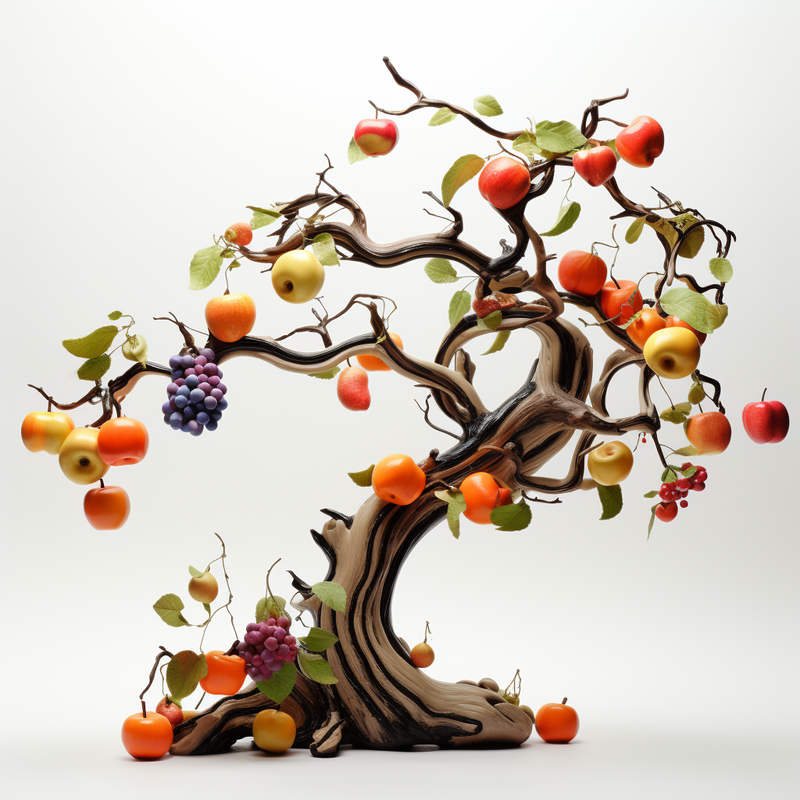
For most companies, Generative AI (GenAI) is no longer a question of if but how. In a Deloitte survey of CEOs, over half said that they were experimenting with GenAI and that it would increase their growth opportunities. Over three quarters said it would increase efficiency. Companies now face a critical decision that may determine whether their GenAI endeavors thrive and spread across the organization or peter out amid frustration and disillusionment. Namely, what GenAI use cases should they go after first? If they choose wisely and deliver rapid business value, they can spark a virtuous cycle of adoption, impact, and further investment. If they heed the siren call of risky, untested, and downright implausible applications of GenAI, they risk their own company-specific AI winter.
Thankfully, a consensus is emerging on a set of GenAI use cases that are lower risk, have a high likelihood of success, and have faster time-to-value. These “low-hanging” projects share common characteristics, distinguishing them from their high-risk counterparts. For example, they focus on augmenting humans versus attempting to replace them. This approach reduces the technical difficulty of the project – since humans can make up for any errors – and allows the projects to deliver value in stages. Further, users are more willing to participate and adopt solutions when they do not fear job losses for themselves or others. The projects also minimize risk by focusing on internal users who can ignore hallucinations versus external customers who may post them on social media.
What do these “low-hanging” projects look like? We’ve researched the projects our customers are undertaking, assessed the GenAI success stories reported in the media, and investigated adjacent use cases. We sought projects bearing the hallmarks of quick and reliable value delivery across a wide range of industries. Our research shows that the most promising projects fit into five classes that align with what the late HBS professor Clay Christensen described as “jobs to be done.” These are the common ways in which companies are putting GenAI to work, and they are:
- First Draft Generator: Providing a warm start on manual, repetitive, creative tasks
- Information Summarizer: Unlocking insights trapped across documents and text databases
- Customer Listener: Analyzing and improving omnichannel conversations at scale
- Data Synthesizer: Protecting privacy and sensitive data while also kick-starting other ML projects
- Application Enhancer: Improving enterprise apps with GenAI capabilities such as search, chat, translation, and more
We collected our findings in The Domino Guide to Low-Hanging Generative AI Projects. The guide details the most common projects and use cases in each of the five classes and their common characteristics. We also touch upon the capabilities required to operationalize these projects successfully. The capabilities range from access to the latest models to the governance framework necessary to ensure they are used responsibly.
If you are looking to accelerate your company’s GenAI journey, we recommend you investigate Domino’s unique GenAI capabilities. Domino’s open platform democratizes access to the full GenAI stack – providing the broadest set of models, tools, techniques, and infrastructures teams need to develop and operationalize GenAI solutions at scale. It also helps bridge the GenAI talent gap with code-generating assistants for fine-tuning GenAI models and templates for common GenAI use cases - including low-hanging projects described here. Across the full lifecycle and all of these technologies, it provides enterprise-grade security and governance and helps companies optimize their AI spend by helping them track costs and leverage the best of hybrid cloud. Find out more.
Kjell Carlsson is the head of AI strategy at Domino Data Lab where he advises organizations on scaling impact with AI technologies. Previously, he covered AI, ML, and data science as a Principal Analyst at Forrester Research. He has written dozens of reports on AI topics ranging from computer vision, MLOps, AutoML, and conversation intelligence to augmented intelligence, next-generation AI technologies, and data science best practices. He has spoken in countless keynotes, panels, and webinars, and is frequently quoted in the media. Dr. Carlsson is also the host of the Data Science Leaders podcast and received his Ph.D. from Harvard University.
RELATED TAGS
Subscribe to the Domino Newsletter
Receive data science tips and tutorials from leading Data Science leaders, right to your inbox.
By submitting this form you agree to receive communications from Domino related to products and services in accordance with Domino's privacy policy and may opt-out at anytime.